Reconstructing the pressure field around a swimming fish using a physics-informed neural network
biorxiv(2023)
摘要
Hydrodynamic pressure is a physical quantity that is utilized by fish and many other aquatic animals to generate thrust and sense the surrounding environment. To advance our understanding of how fish react to unsteady flows, it is necessary to intercept the pressure signals sensed by their lateral line system. In this study, the authors propose a new, non-invasive method for reconstructing the instantaneous pressure field around a swimming fish from 2D particle image velocimetry (PIV) measurements. The method uses a physics-informed neural network (PINN) to predict an optimized solution for the velocity and pressure fields that satisfy in an L2 sense both the Navier Stokes equations and the constraints put forward by the measurements. The method was validated using a direct numerical simulation of a swimming mackerel, Scomber scombrus, and was applied to empirically obtained data of a turning zebrafish, Danio rerio. The results demonstrate that when compared to traditional methods that rely on directly integrating the pressure gradient field, the PINN is less sensitive to the spatio-temporal resolution of the velocity field measurements and provides a more accurate pressure reconstruction, particularly on the surface of the body.
### Competing Interest Statement
The authors have declared no competing interest.
更多查看译文
关键词
swimming fish,pressure field,neural network,physics-informed
AI 理解论文
溯源树
样例
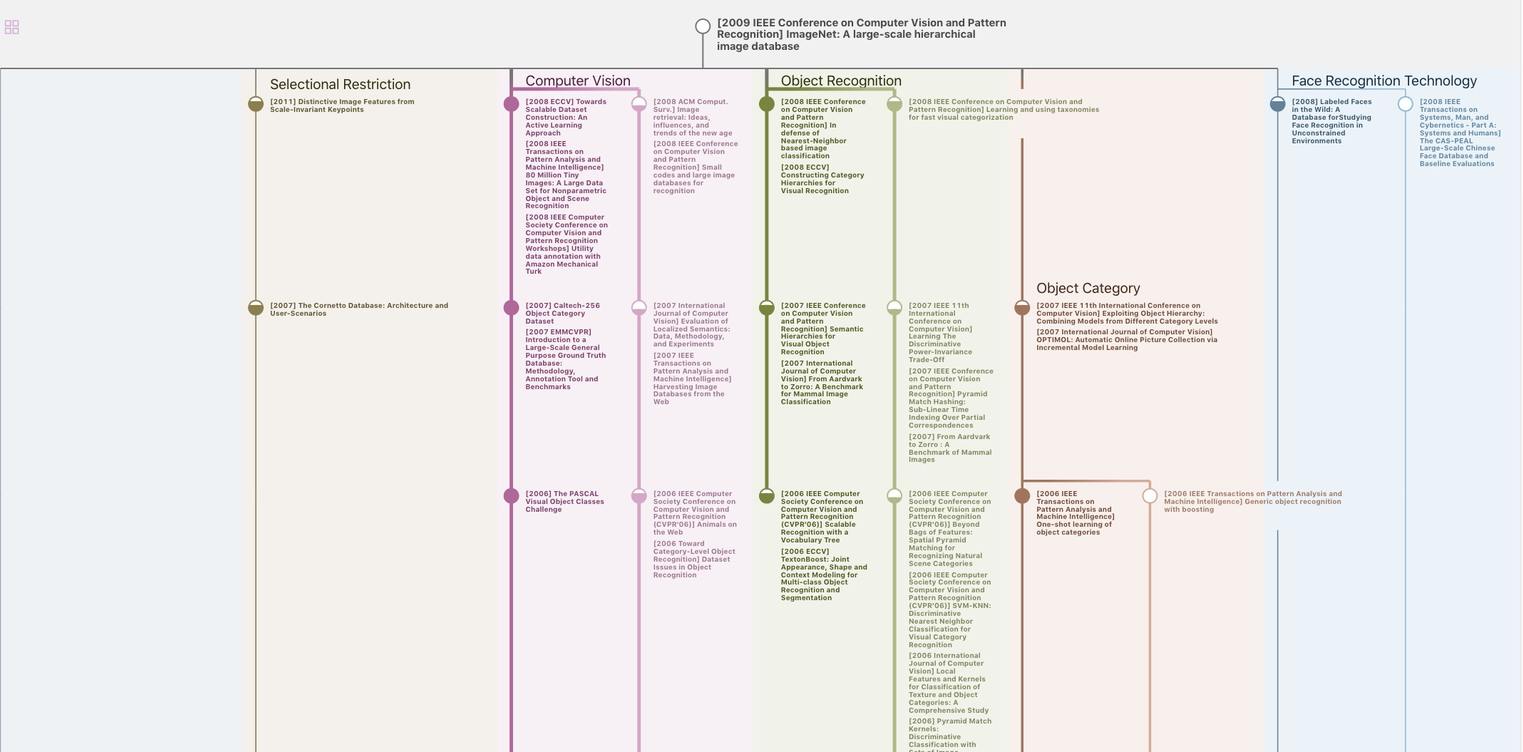
生成溯源树,研究论文发展脉络
Chat Paper
正在生成论文摘要