SAR Image Ship Target Detection Adversarial Attack and Defence Generalization Research.
Sensors (Basel, Switzerland)(2023)
摘要
The synthetic aperture radar (SAR) image ship detection system needs to adapt to an increasingly complicated actual environment, and the requirements for the stability of the detection system continue to increase. Adversarial attacks deliberately add subtle interference to input samples and cause models to have high confidence in output errors. There are potential risks in a system, and input data that contain confrontation samples can be easily used by malicious people to attack the system. For a safe and stable model, attack algorithms need to be studied. The goal of traditional attack algorithms is to destroy models. When defending against attack samples, a system does not consider the generalization ability of the model. Therefore, this paper introduces an attack algorithm which can improve the generalization of models by based on the attributes of Gaussian noise, which is widespread in actual SAR systems. The attack data generated by this method have a strong effect on SAR ship detection models and can greatly reduce the accuracy of ship recognition models. While defending against attacks, filtering attack data can effectively improve the model defence capabilities. Defence training greatly improves the anti-attack capacity, and the generalization capacity of the model is improved accordingly.
更多查看译文
关键词
SAR image,adversarial attack,adversarial defence,generalization,ship target detection
AI 理解论文
溯源树
样例
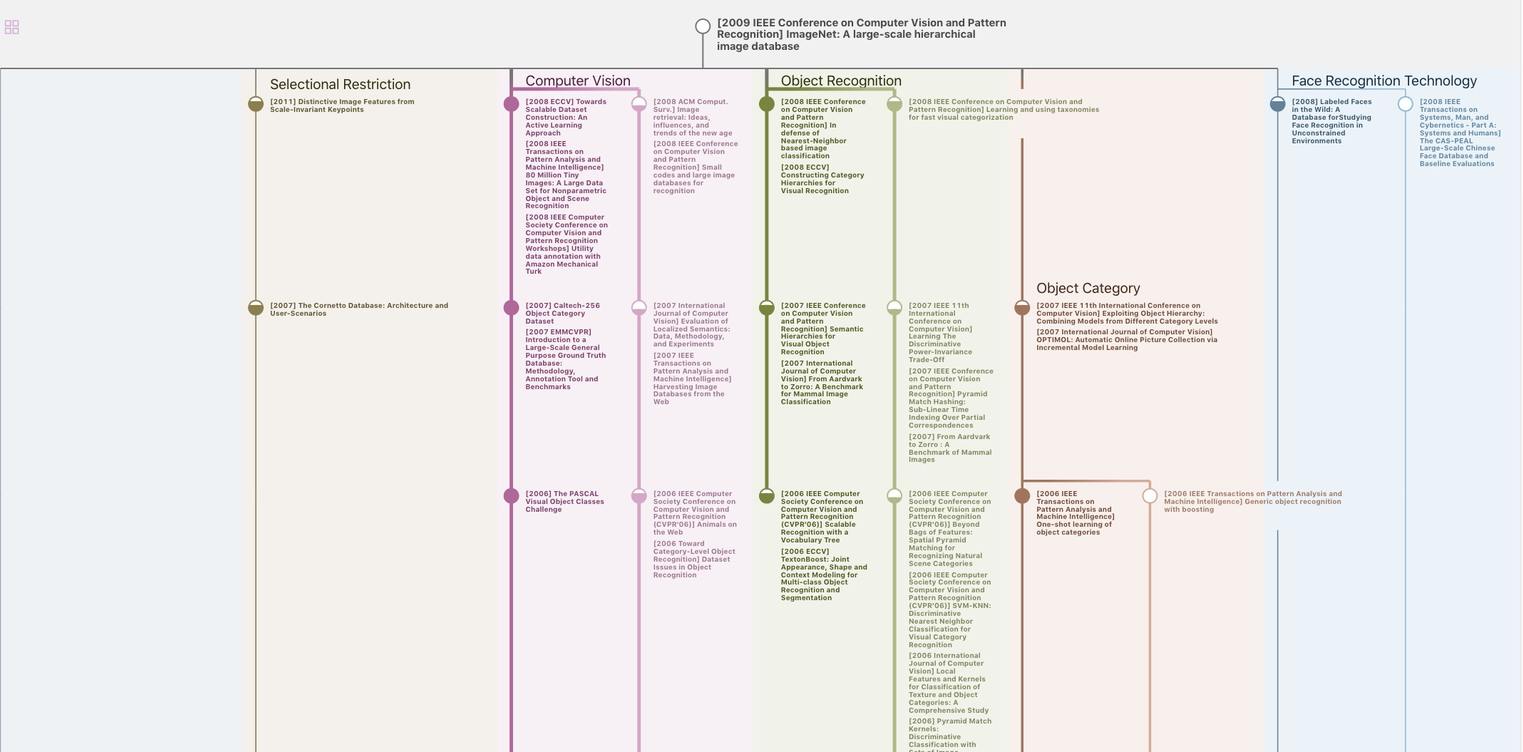
生成溯源树,研究论文发展脉络
Chat Paper
正在生成论文摘要