Rethink Long-tailed Recognition with Vision Transforms.
ICASSP 2023 - 2023 IEEE International Conference on Acoustics, Speech and Signal Processing (ICASSP)(2023)
Abstract
In the real world, data tends to follow long-tailed distributions w.r.t. class or attribution, motivating the challenging Long-Tailed Recognition (LTR) problem. In this paper, we revisit recent LTR methods with promising Vision Transformers (ViT). We figure out that 1) ViT is hard to train with long-tailed data. 2) ViT learns generalized features in an unsupervised manner, like mask generative training, either on long-tailed or balanced datasets. Hence, we propose to adopt unsupervised learning to utilize long-tailed data. Furthermore, we propose the Predictive Distribution Calibration (PDC) as a novel metric for LTR, where the model tends to simply classify inputs into common classes. Our PDC can measure the model calibration of predictive preferences quantitatively. On this basis, we find many LTR approaches alleviate it slightly, despite the accuracy improvement. Extensive experiments on benchmark datasets validate that PDC reflects the model's predictive preference precisely, which is consistent with the visualization.
MoreTranslated text
Key words
metric,long-tailed learning,vision transformers,representation learning,imbalanced data
AI Read Science
Must-Reading Tree
Example
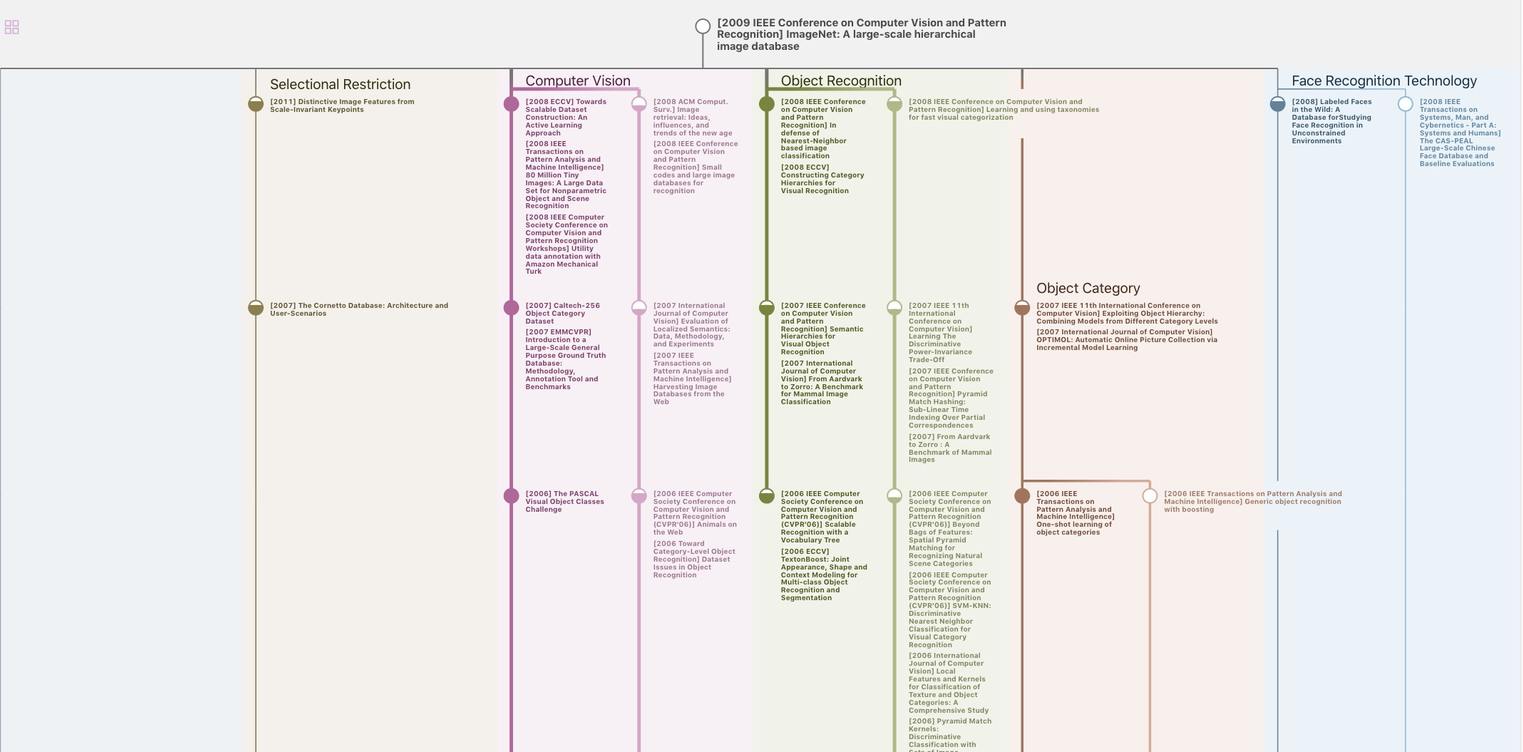
Generate MRT to find the research sequence of this paper
Chat Paper
Summary is being generated by the instructions you defined