Semi-supervised clustering with two types of background knowledge: Fusing pairwise constraints and monotonicity constraints
INFORMATION FUSION(2024)
摘要
This study addresses the problem of performing clustering in the presence of two types of background knowledge: pairwise constraints and monotonicity constraints. Pairwise constraint relate pair of instances, indicating if they must or must not belong to the same cluster, while monotonicity constraints impose and order relationship between the cluster labels, and are applied to the dataset globally. To fuse these to types of background knowledge, the formal framework to perform clustering under monotonicity constraints is, firstly, defined, resulting in a specific distance measure. Pairwise constraints are integrated afterwards by designing an objective function which combines the proposed distance measure and a pairwise constraint based penalty term, in order to fuse both types of information. This objective function can be optimized with an EM optimization scheme. The proposed method serves as the first approach to the problem it addresses, as it is the first method designed to work with the two types of background knowledge mentioned above. Our proposal is tested with previous approaches from different clustering paradigms in monotonic datasets, including a real-world case of study. Bayesian statistical tests are used to provide empirical evidence in favor of our proposal, and fund subsequent conclusions: the proposed method can be used obtain better results than previous approaches within the monotonic constrained clustering paradigm.
更多查看译文
关键词
Pairwise constraints,Monotonicity constraints,Expectation-minimization,Semi-supervised learning,Machine learning
AI 理解论文
溯源树
样例
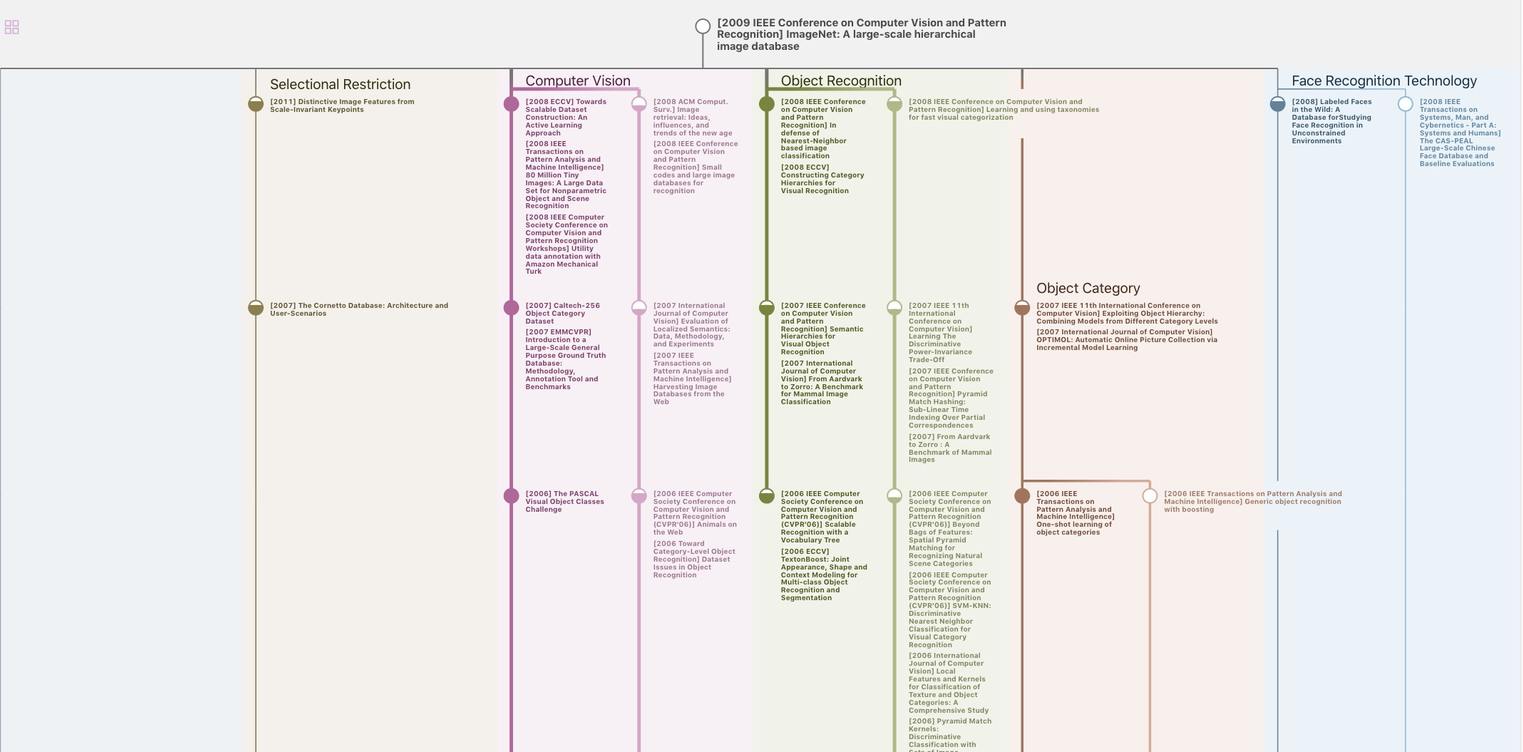
生成溯源树,研究论文发展脉络
Chat Paper
正在生成论文摘要