Framework for dual-energy-like chest radiography image synthesis from single-energy computed tomography based on cycle-consistent generative adversarial network
MEDICAL PHYSICS(2024)
摘要
BackgroundDual-energy (DE) chest radiography (CXR) enables the selective imaging of two relevant materials, namely, soft tissue and bone structures, to better characterize various chest pathologies (i.e., lung nodule, bony lesions, etc.) and potentially improve CXR-based diagnosis. Recently, deep-learning-based image synthesis techniques have attracted considerable attention as alternatives to existing DE methods (i.e., dual-exposure-based and sandwich-detector-based methods) because software-based bone-only and bone-suppression images in CXR could be useful. PurposeThe objective of this study was to develop a new framework for DE-like CXR image synthesis from single-energy computed tomography (CT) based on a cycle-consistent generative adversarial network. MethodsThe core techniques of the proposed framework are divided into three categories: (1) data configuration from the generation of pseudo CXR from single energy CT, (2) learning of the developed network architecture using pseudo CXR and pseudo-DE imaging using a single-energy CT, and (3) inference of the trained network on real single-energy CXR. We performed a visual inspection and comparative evaluation using various metrics and introduced a figure of image quality (FIQ) to consider the effects of our framework on the spatial resolution and noise in terms of a single index through various test cases. ResultsOur results indicate that the proposed framework is effective and exhibits potential synthetic imaging ability for two relevant materials: soft tissue and bone structures. Its effectiveness was validated, and its ability to overcome the limitations associated with DE imaging techniques (e.g., increase in exposure dose owing to the requirement of two acquisitions, and emphasis on noise characteristics) via an artificial intelligence technique was presented. ConclusionsThe developed framework addresses X-ray dose issues in the field of radiation imaging and enables pseudo-DE imaging with single exposure.
更多查看译文
关键词
chest X-ray radiographs,deep learning,dual-energy,synthesized dual-energy imaging
AI 理解论文
溯源树
样例
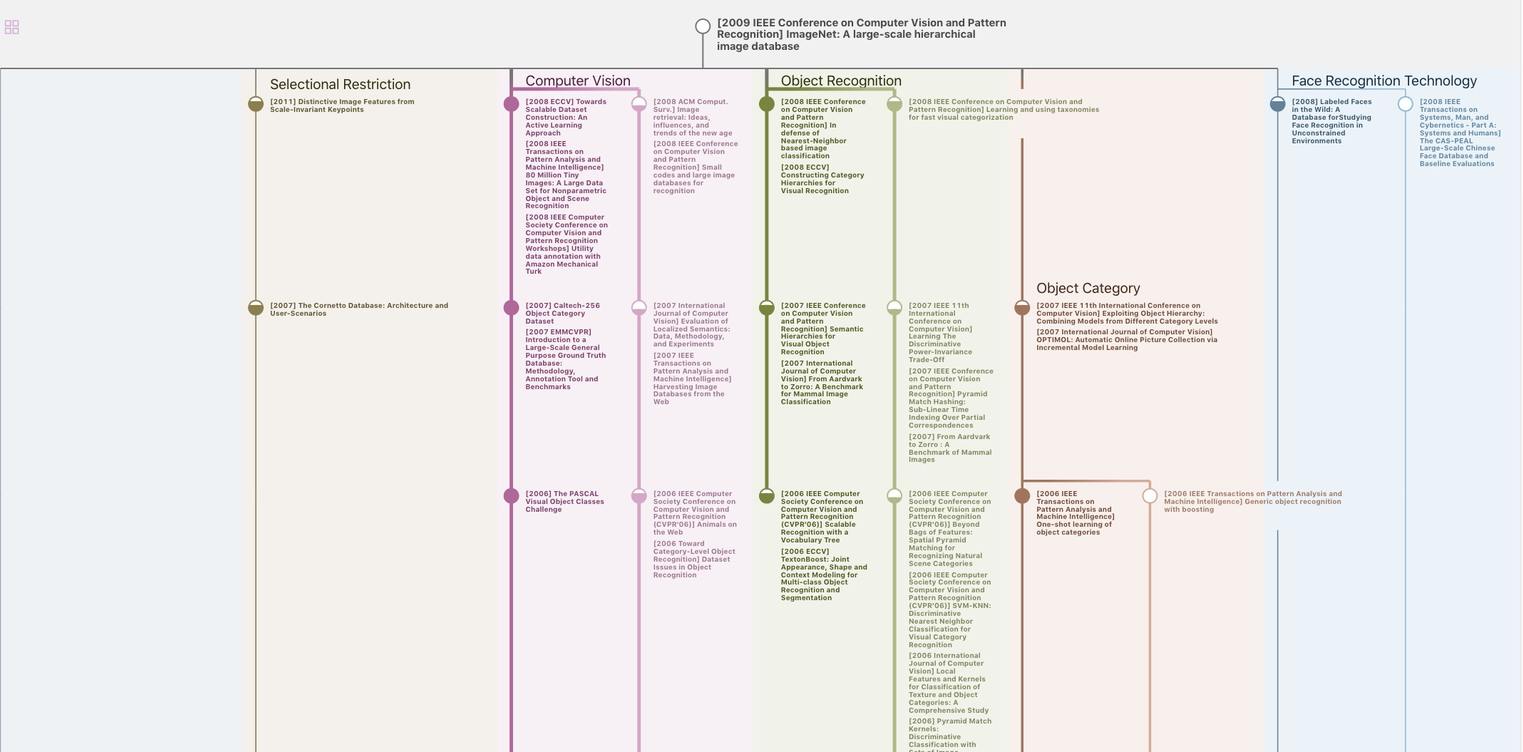
生成溯源树,研究论文发展脉络
Chat Paper
正在生成论文摘要