Toward a blind image quality evaluator in the wild by learning beyond human opinion scores
Pattern Recognition(2023)
摘要
•A unified learning framework is proposed to learn computational opinion-free BIQA models from synthetically-distorted images for the BIQA in the wild without using human scores. Agent-specific and agent-agnostic modules are designed to learn FR-IQA annotators' agree-to-disagree information.•A simple and easy-to-implement yet effective UDA method, incorporating an adaptive weight loss and domain mix-up, to reduce the distributional shift between synthetically-distorted images and the authentically-distorted ones captured in the wild. To the best of our knowledge, our work is the first to exploit adversarial UDA for IQA.•Extensive experiments on two large-scale realistic IQA datasets demonstrate that our proposed method achieves state-of-the-art performance when being evaluated using both human opinion scores and gMAD competition.
更多查看译文
关键词
Blind image quality assessment,Opinion-free,Pseudo binary label,Unsupervised domain adaptation,gMAD competition
AI 理解论文
溯源树
样例
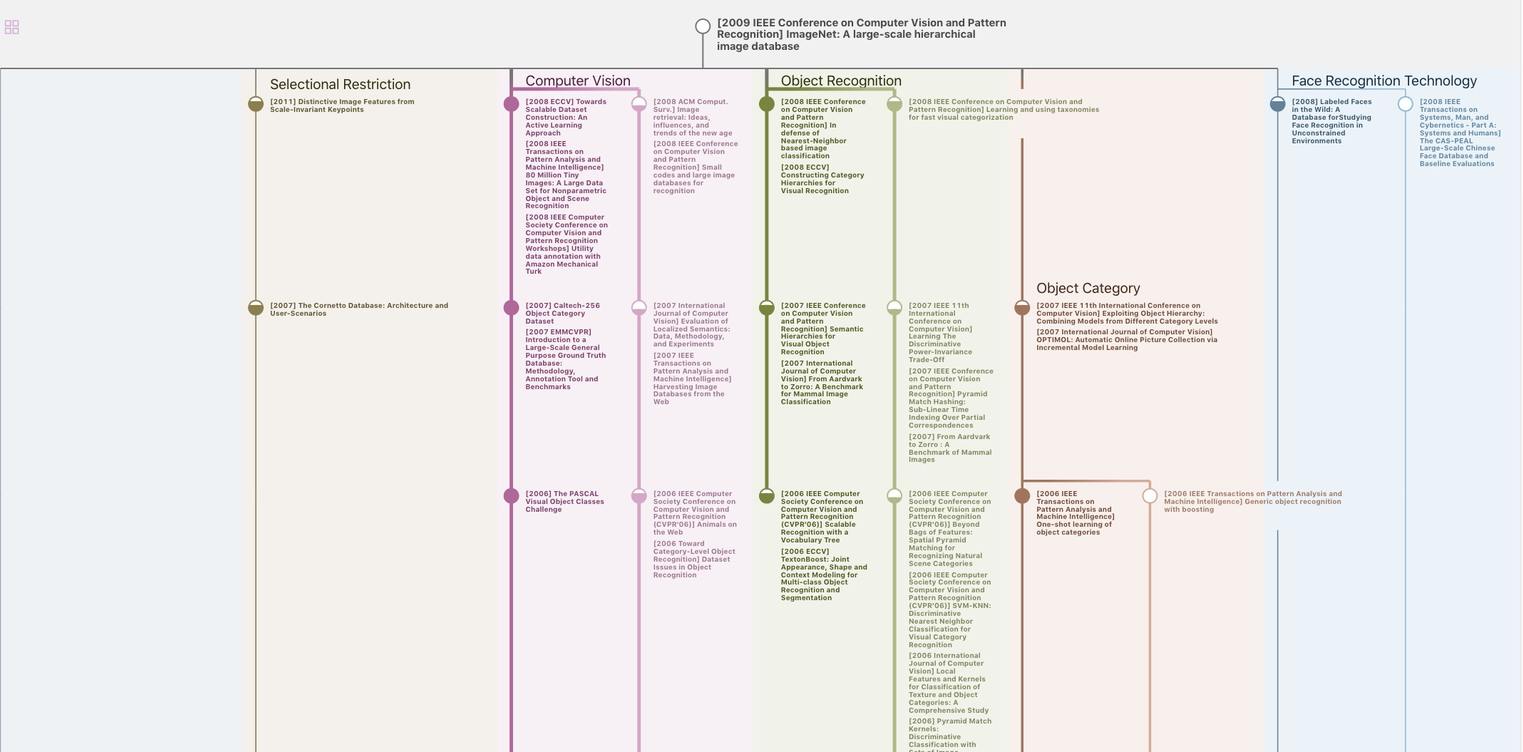
生成溯源树,研究论文发展脉络
Chat Paper
正在生成论文摘要