Deep Imbalanced Time-Series Forecasting via Local Discrepancy Density
MACHINE LEARNING AND KNOWLEDGE DISCOVERY IN DATABASES: RESEARCH TRACK, ECML PKDD 2023, PT V(2023)
摘要
Time-series forecasting models often encounter abrupt changes in a given period of time which generally occur due to unexpected or unknown events. Despite their scarce occurrences in the training set (i.e., data imbalance), abrupt changes incur loss that significantly contributes to the total loss (i.e., heteroscedasticity). Therefore, they act as noisy training samples and prevent the model from learning generalizable patterns, namely the normal states. To resolve overfitting problem posed by heteroscedasticity and data imbalance, we propose a reweighting framework that down-weights the losses incurred by abrupt changes and up-weights those by normal states. For the reweighting framework, we first define a measurement termed Local Discrepancy (LD) which measures the degree of abruptness of a change in a given period of time. Since a training set is mostly composed of normal states, we then consider how frequently the temporal changes appear in the training set based on LD (i.e., estimated LD density). Our reweighting framework is applicable to existing time-series forecasting models regardless of the architectures. Through extensive experiments on 12 time-series forecasting models over eight datasets with various in-output sequence lengths, we demonstrate that applying our reweighting framework reduces MSE by 10.1% on average and by up to 18.6% in the state-of-the-art model.
更多查看译文
关键词
Time-series forecasting,Data imbalance,Noisy samples
AI 理解论文
溯源树
样例
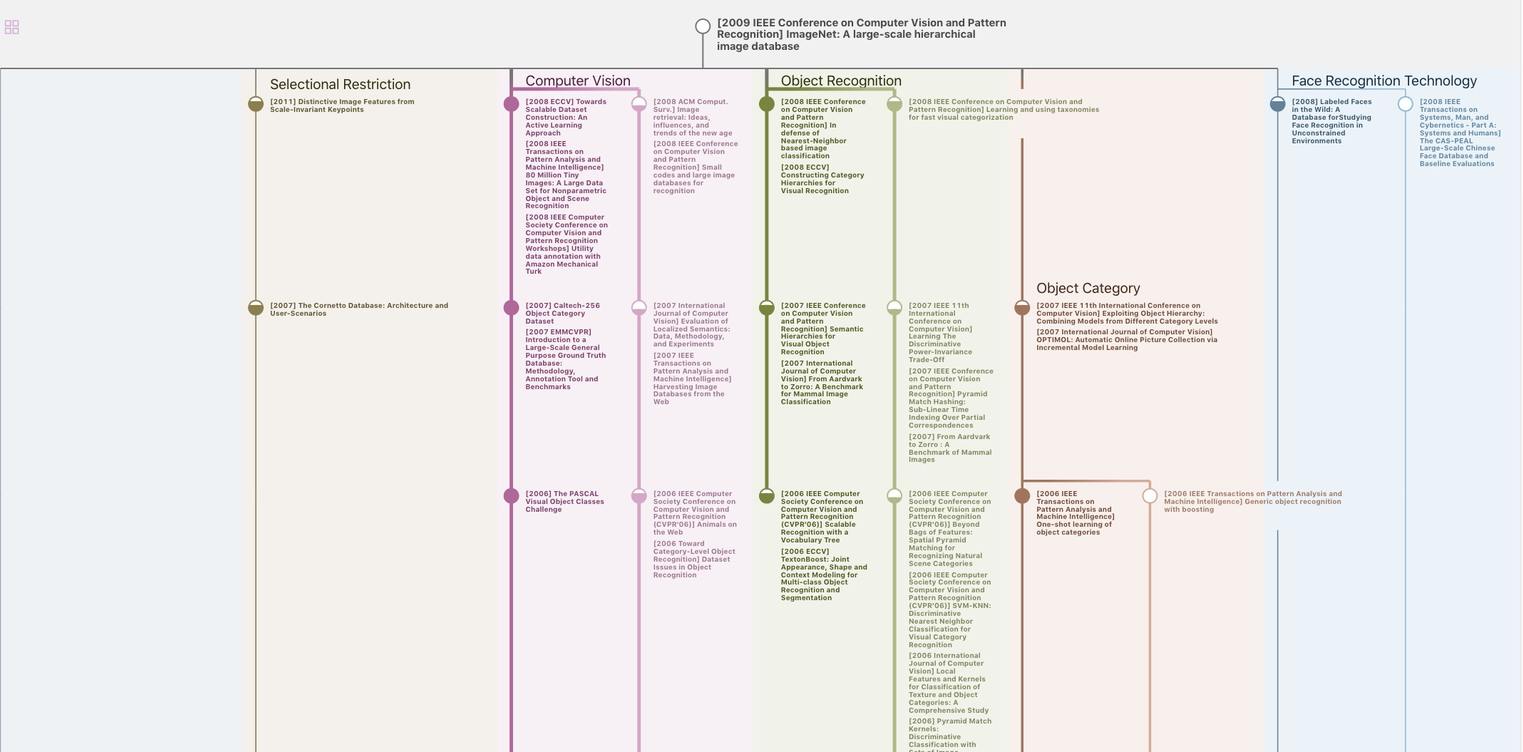
生成溯源树,研究论文发展脉络
Chat Paper
正在生成论文摘要