Toward Fairness in Text Generation via Mutual Information Minimization based on Importance Sampling
arxiv(2023)
摘要
Pretrained language models (PLMs), such as GPT2, have achieved remarkable empirical performance in text generation tasks. However, pretrained on large-scale natural language corpora, the generated text from PLMs may exhibit social bias against disadvantaged demographic groups. To improve the fairness of PLMs in text generation, we propose to minimize the mutual information between the semantics in the generated text sentences and their demographic polarity, i.e., the demographic group to which the sentence is referring. In this way, the mentioning of a demographic group (e.g., male or female) is encouraged to be independent from how it is described in the generated text, thus effectively alleviating the social bias. Moreover, we propose to efficiently estimate the upper bound of the above mutual information via importance sampling, leveraging a natural language corpus. We also propose a distillation mechanism that preserves the language modeling ability of the PLMs after debiasing. Empirical results on real-world benchmarks demonstrate that the proposed method yields superior performance in term of both fairness and language modeling ability.
更多查看译文
关键词
text generation,fairness,mutual information minimization,mutual information
AI 理解论文
溯源树
样例
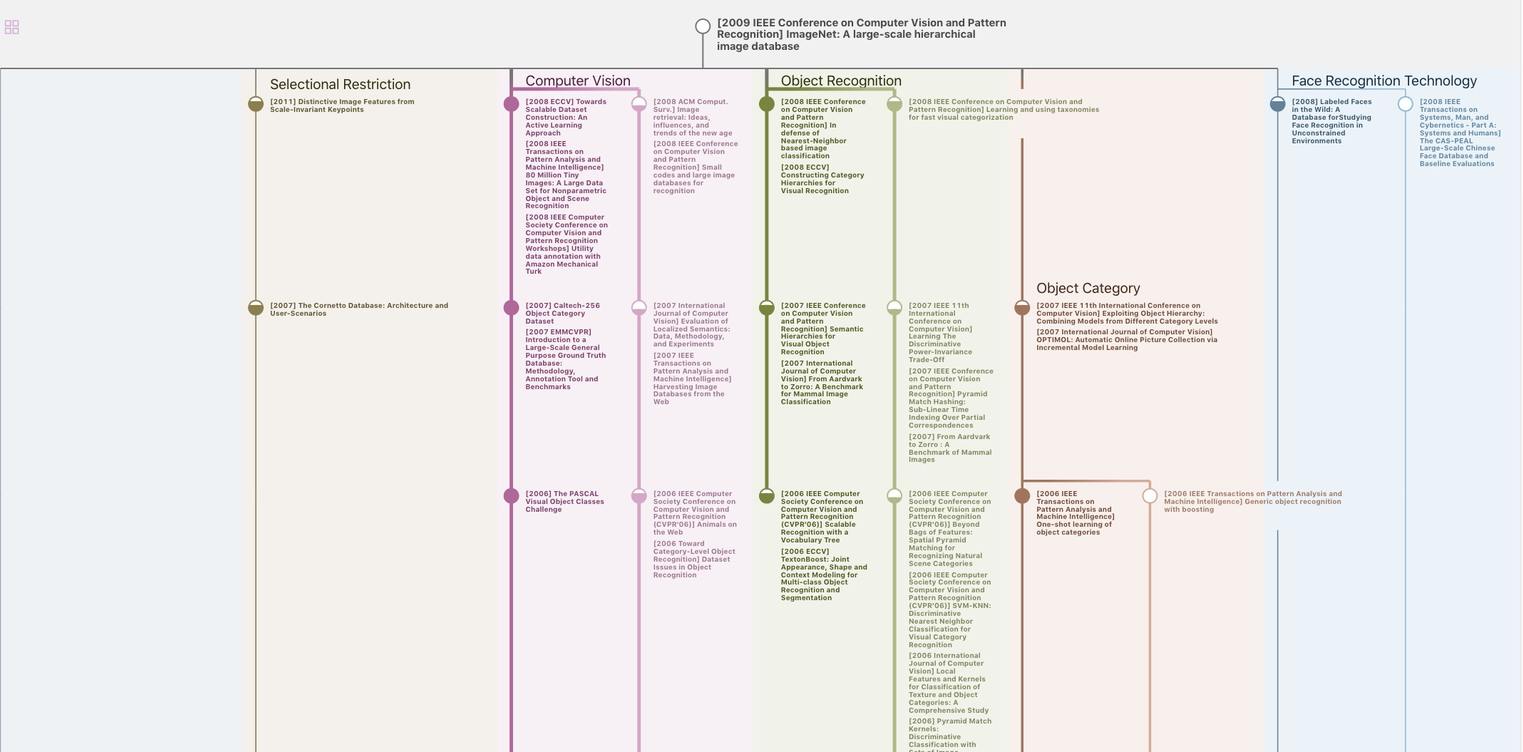
生成溯源树,研究论文发展脉络
Chat Paper
正在生成论文摘要