MASS: Mobility-Aware Sensor Scheduling of Cooperative Perception for Connected Automated Driving
IEEE TRANSACTIONS ON VEHICULAR TECHNOLOGY(2023)
摘要
Timely and reliable environment perception is fundamental to safe and efficient automated driving. However, the perception of standalone intelligence inevitably suffers from occlusions. A new paradigm, Cooperative Perception (CP), comes to the rescue by sharing sensor data from another perspective, i.e., from a cooperative vehicle (CoV). Due to the limited communication bandwidth, it is essential to schedule the most beneficial CoV, considering both the viewpoints and communication quality. Existing methods rely on the exchange of meta-information, such as visibility maps, to predict the perception gains from nearby vehicles, which induces extra communication and processing overhead. In this article, we propose a new approach, learning while scheduling, for distributed scheduling of CP. The solution enables CoVs to predict the perception gains using past observations, leveraging the temporal continuity of perception gains. Specifically, we design a mobility-aware sensor scheduling (MASS) algorithm based on the restless multi-armed bandit (RMAB) theory to maximize the expected average perception gain. An upper bound on the expected average learning regret is proved, which matches the lower bound of any online algorithm up to a logarithmic factor. Extensive simulations are carried out on realistic traffic traces. The results show that the proposed MASS algorithm achieves the best average perception gain and improves recall by up to 3.1 percentage points compared to other learning-based algorithms. Finally, a case study on a trace of LiDAR frames qualitatively demonstrates the superiority of adaptive exploration, the key element of the MASS algorithm.
更多查看译文
关键词
Feature extraction,Bandwidth,Vehicle-to-everything,Point cloud compression,Wireless sensor networks,Wireless communication,Laser radar,Cooperative perception (CP),mobility-aware,restless multi-armed bandit,sensor scheduling
AI 理解论文
溯源树
样例
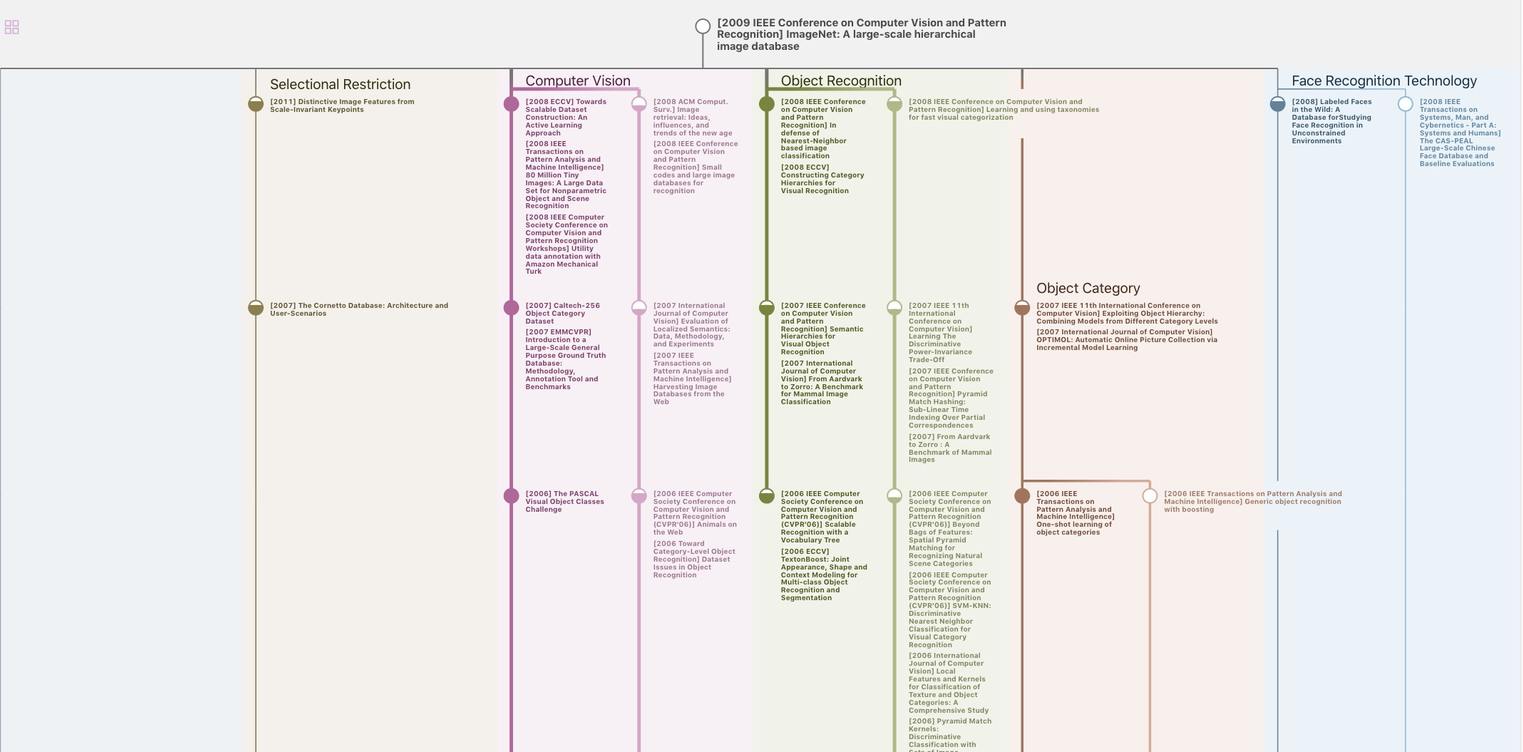
生成溯源树,研究论文发展脉络
Chat Paper
正在生成论文摘要