Permutation Tests for Assessing Potential Non-Linear Associations between Treatment Use and Multivariate Clinical Outcomes
MULTIVARIATE BEHAVIORAL RESEARCH(2024)
摘要
In many psychometric applications, the relationship between the mean of an outcome and a quantitative covariate is too complex to be described by simple parametric functions; instead, flexible nonlinear relationships can be incorporated using penalized splines. Penalized splines can be conveniently represented as a linear mixed effects model (LMM), where the coefficients of the spline basis functions are random effects. The LMM representation of penalized splines makes the extension to multivariate outcomes relatively straightforward. In the LMM, no effect of the quantitative covariate on the outcome corresponds to the null hypothesis that a fixed effect and a variance component are both zero. Under the null, the usual asymptotic chi-square distribution of the likelihood ratio test for the variance component does not hold. Therefore, we propose three permutation tests for the likelihood ratio test statistic: one based on permuting the quantitative covariate, the other two based on permuting residuals. We compare via simulation the Type I error rate and power of the three permutation tests obtained from joint models for multiple outcomes, as well as a commonly used parametric test. The tests are illustrated using data from a stimulant use disorder psychosocial clinical trial.
更多查看译文
关键词
Chi-square distribution,joint tests,likelihood ratio test,linear mixed effects model,multiple outcomes
AI 理解论文
溯源树
样例
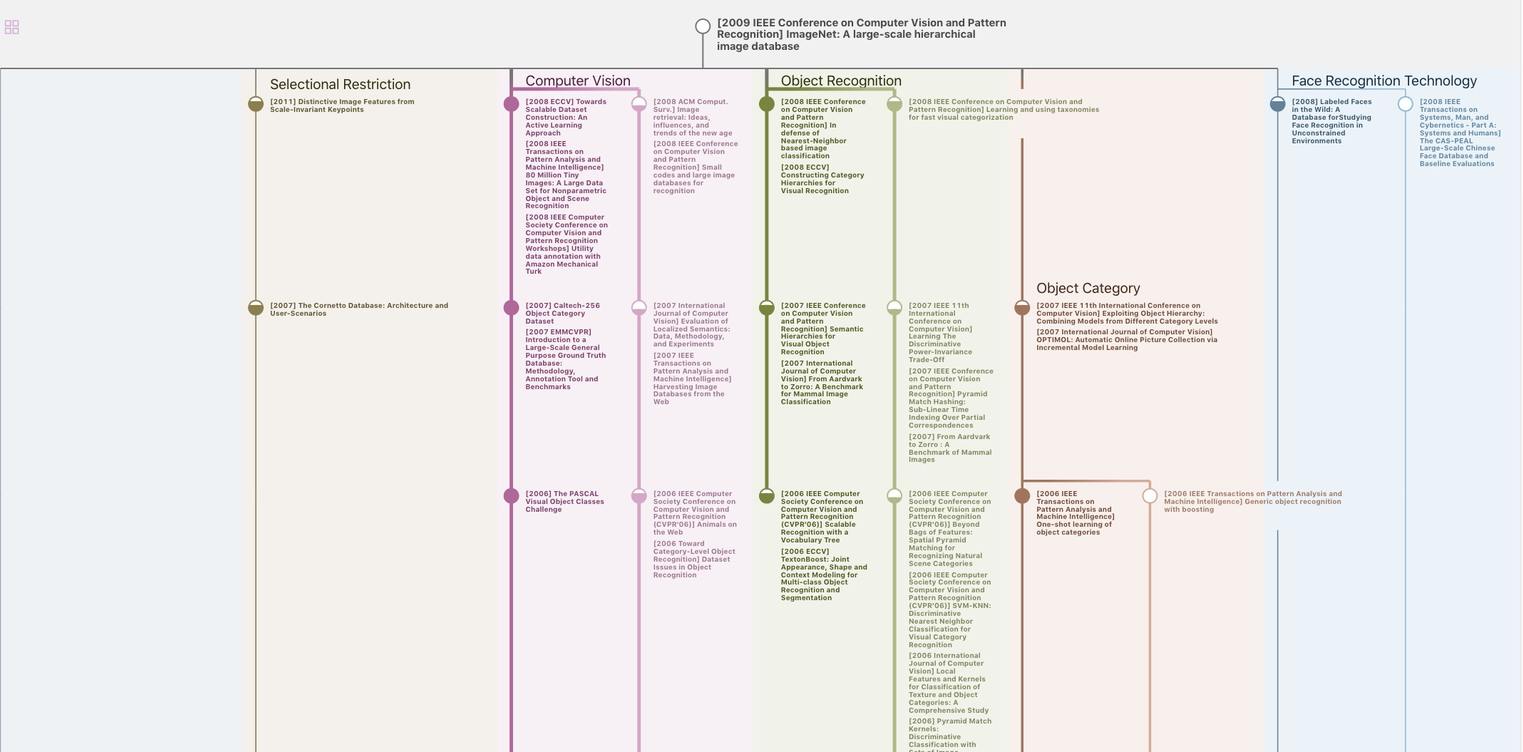
生成溯源树,研究论文发展脉络
Chat Paper
正在生成论文摘要