Elucidation of genome-wide understudied proteins targeted by PROTAC-induced degradation using interpretable machine learning
biorxiv(2023)
摘要
Proteolysis-targeting chimeras (PROTACs) are hetero-bifunctional molecules that induce the degradation of target proteins by recruiting an E3 ligase. PROTACs have the potential to inactivate disease-related genes that are considered undruggable by small molecules, making them a promising therapy for the treatment of incurable diseases. However, only a few hundred proteins have been experimentally tested for their amenability to PROTACs, and it remains unclear which other proteins in the entire human genome can be targeted by PROTACs. In this study, we have developed PrePROTAC, an interpretable machine learning model based on a transformer-based protein sequence descriptor and random forest classification. PrePROTAC predicts genome-wide targets that can be degraded by CRBN, one of the E3 ligases. In the benchmark studies, PrePROTAC achieved a ROC-AUC of 0.81, an average precision of 0.84, and over 40% sensitivity at a false positive rate of 0.05. When evaluated by an external test set which comprised proteins from different structural folds than those in the training set, the performance of PrePROTAC did not drop significantly, indicating its generalizability. Furthermore, we developed an embedding SHapley Additive exPlanations (eSHAP) method, which extends conventional SHAP analysis for original features to an embedding space through in silico mutagenesis. This method allowed us to identify key residues in the protein structure that play critical roles in PROTAC activity. The identified key residues were consistent with existing knowledge. Using PrePROTAC, we identified over 600 novel understudied proteins that are potentially degradable by CRBN and proposed PROTAC compounds for three novel drug targets associated with Alzheimer's disease. Author summaryMany human diseases remain incurable because disease-causing genes cannot by selectively and effectively targeted by small molecules. Proteolysis-targeting chimera (PROTAC), an organic compound that binds to both a target and a degradation-mediating E3 ligase, has emerged as a promising approach to selectively target disease-driving genes that are not druggable by small molecules. However, not all of proteins can be accommodated by E3 ligases, and be effectively degraded. Knowledge about the degradability of a protein will be crucial for PROTAC design. However, only hundreds of proteins have been experimentally tested if they are amenable to the PROTACs. This leaves us uncertain about which other proteins in the entire human genome can be targeted by PROTACs. In this paper, we propose an intepretable machine learning model, PrePROTAC, which takes advantage of powerful protein language modeling. PrePROTAC achieves high accuracy when evaluated with an external dataset which comes from different gene families from the proteins in the training data, suggesting the generalizability of this model. We apply PrePROTAC to the human genome, and identify more than 600 understudied proteins that are potentially responsive to PROTACs. Furthermore, we design PROTAC compounds for three novel drug targets associated with Alzheimer's disease.
更多查看译文
关键词
proteins,interpretable machine learning,degradation,genome-wide,protac-induced
AI 理解论文
溯源树
样例
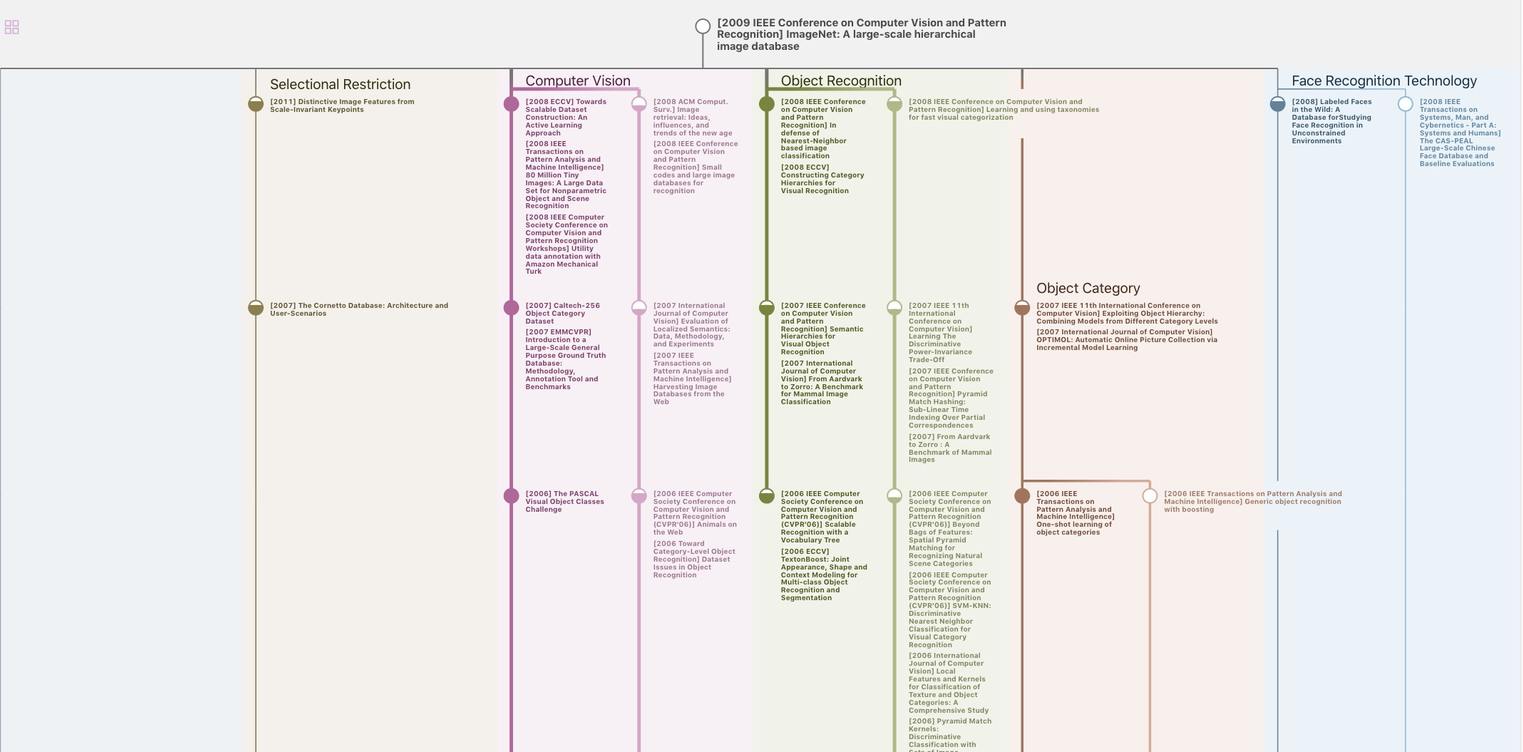
生成溯源树,研究论文发展脉络
Chat Paper
正在生成论文摘要