Ensemble deep learning of embeddings for clustering multimodal single-cell omics data
biorxiv(2023)
摘要
Motivation Recent advances in multimodal single-cell omics technologies enable multiple modalities of molecular attributes, such as gene expression, chromatin accessibility, and protein abundance, to be profiled simultaneously at a global level in individual cells. While the increasing availability of multiple data modalities is expected to provide a more accurate clustering and characterisation of cells, the development of computational methods that are capable of extracting information embedded across data modalities is still in its infancy.
Results We propose SnapCCESS for clustering cells by integrating data modalities in multimodal singlecell omics data using an unsupervised ensemble deep learning framework. By creating snapshots of embeddings of multimodality using variational autoencoders, SnapCCESS can be coupled with various clustering algorithms for generating consensus clustering of cells. We applied SnapCCESS with several clustering algorithms to various datasets generated from popular multimodal single-cell omics technologies. Our results demonstrate that SnapCCESS is effective and more efficient than conventional ensemble deep learning-based clustering methods and outperforms other state-of-the-art multimodal embedding generation methods in integrating data modalities for clustering cells. The improved clustering of cells from SnapCCESS will pave the way for more accurate characterisation of cell identity and types, an essential step for various downstream analyses of multimodal single-cell omics data.
Availability and implementation SnapCCESS is implemented as a Python package and is freely available from .
### Competing Interest Statement
The authors have declared no competing interest.
更多查看译文
关键词
ensemble deep learning,embeddings,deep learning,single-cell
AI 理解论文
溯源树
样例
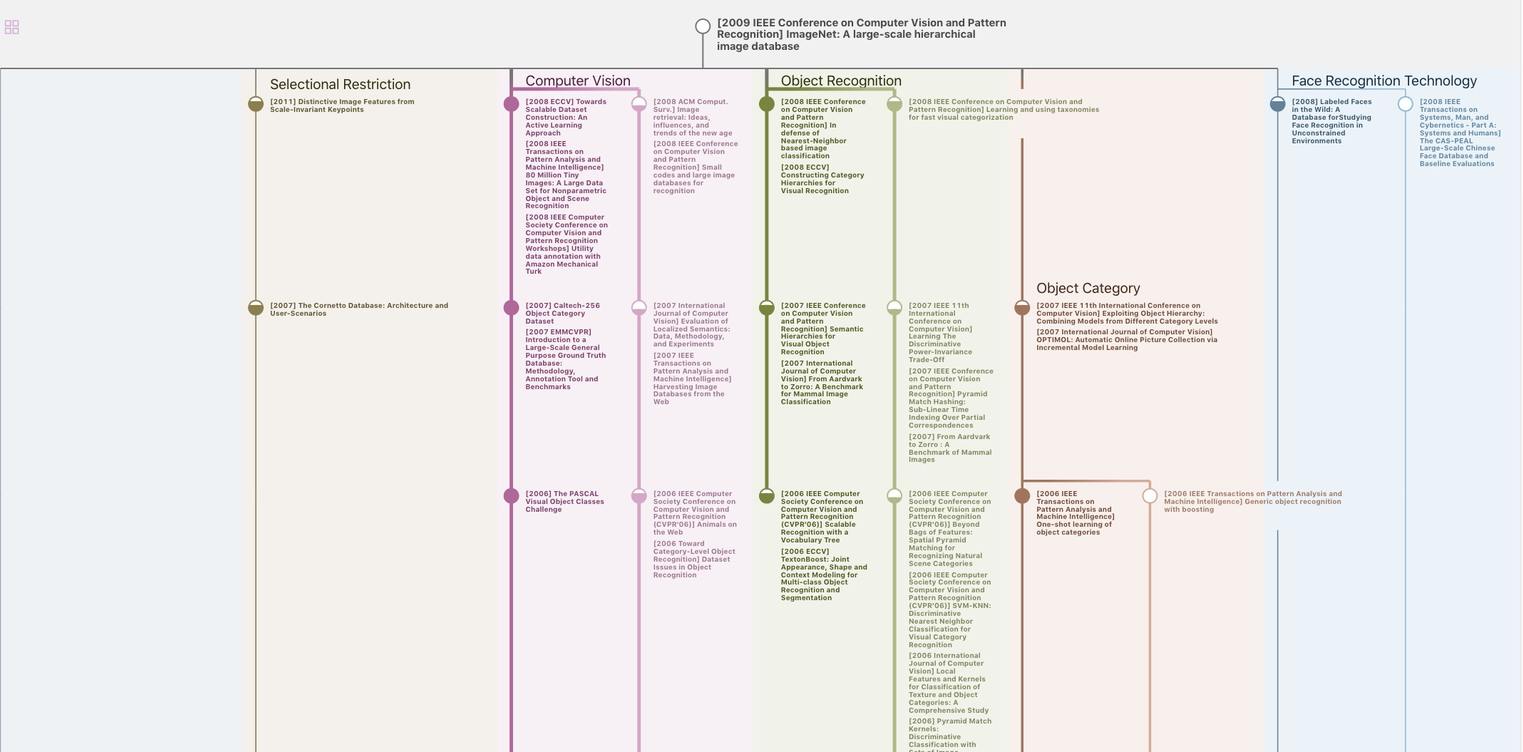
生成溯源树,研究论文发展脉络
Chat Paper
正在生成论文摘要