Pacybara: Accurate long-read sequencing for barcoded mutagenized allelic libraries
biorxiv(2023)
摘要
Summary Long read sequencing technologies, an attractive solution for many applications, often suffer from higher error rates. Alignment of multiple reads can improve base-calling accuracy, but some applications, e.g. sequencing mutagenized libraries where multiple distinct clones differ by one or few variants, require the use of barcodes or unique molecular identifiers. Unfortunately, sequencing errors can interfere with correct barcode identification, and a given barcode sequence may be linked to multiple independent clones within a given library.
Here we focus on the target application of sequencing mutagenized libraries in the context of multiplexed assays of variant effects (MAVEs). MAVEs are increasingly used to create comprehensive genotype-phenotype maps that can aid clinical variant interpretation. Many MAVE methods use long-read sequencing of barcoded mutant libraries for accurate association of barcode with genotype. Existing long-read sequencing pipelines do not account for inaccurate sequencing or non-unique barcodes. Here, we describe Pacybara, which handles these issues by clustering long reads based on the similarities of (error-prone) barcodes while also detecting barcodes that have been associated with multiple genotypes. Pacybara also detects recombinant (chimeric) clones and reduces false positive indel calls. In three example applications, we show that Pacybara identifies and correctly resolves these issues.
Availability and Implementation Pacybara, freely available at , is implemented using R, Python and bash for Linux. It has both a single-threaded implementation and, for GNU/Linux clusters that use Slurm, PBS, or GridEngine schedulers, a multi-node version.
Supplementary Material Supplementary materials are available at Bioinformatics online.
### Competing Interest Statement
FP is a shareholder and advisor for SeqWell, Constantiam, BioSymetrics, and a shareholder of Ranomics.
更多查看译文
AI 理解论文
溯源树
样例
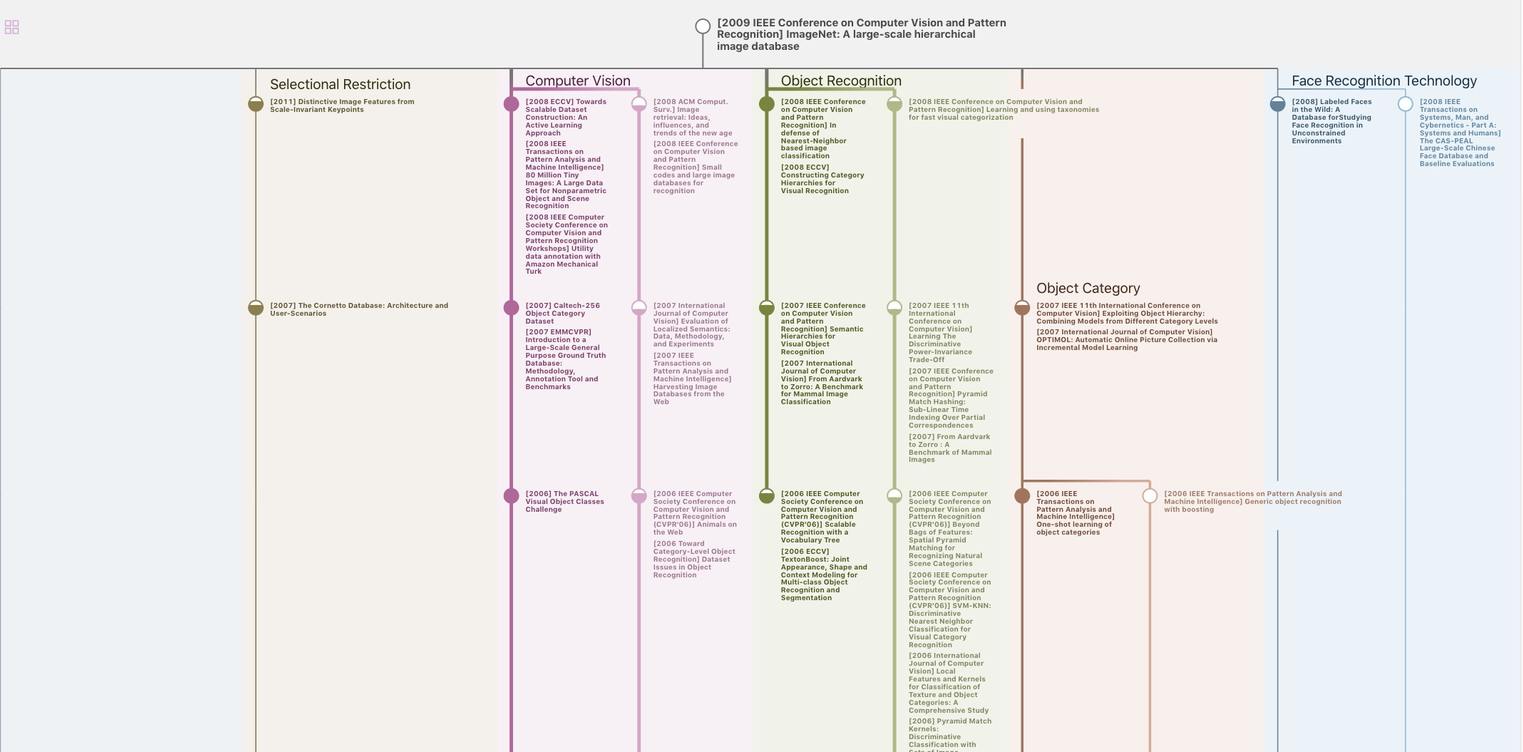
生成溯源树,研究论文发展脉络
Chat Paper
正在生成论文摘要