ReSemble: Reinforced Ensemble Framework for Data Prefetching
SC22: International Conference for High Performance Computing, Networking, Storage and Analysis(2022)
摘要
Data prefetching hides memory latency by predicting and loading necessary data into cache beforehand. Most prefetchers in the literature are efficient for specific memory address patterns thereby restricting their utility to specialized applications-they do not perform well on hybrid applications with multifarious access patterns. Therefore we propose ReSem-ble: a Reinforcement Learning (RL) based adaptive enSemble framework that enables multiple prefetchers to complement each other on hybrid applications. Our RL trained ensemble controller takes prefetch suggestions from all prefetchers as input, selects the best suggestion dynamically, and learns online toward getting higher cumulative rewards, which are collected from prefetch hits/misses. Our ensemble framework using a simple multilayer perceptron as the controller achieves on the average 85.27 % (accuracy) and 44.22 % (coverage), leading to 31.02 % IPC improvement, which outperforms state-of-the-art individual prefetchers by 8.35%-26.11 %, while also outperforming SBP, a state-of-the-art (non-RL) ensemble prefetcher by 5.69%.
更多查看译文
关键词
reinforcement learning,ensemble,prefetching
AI 理解论文
溯源树
样例
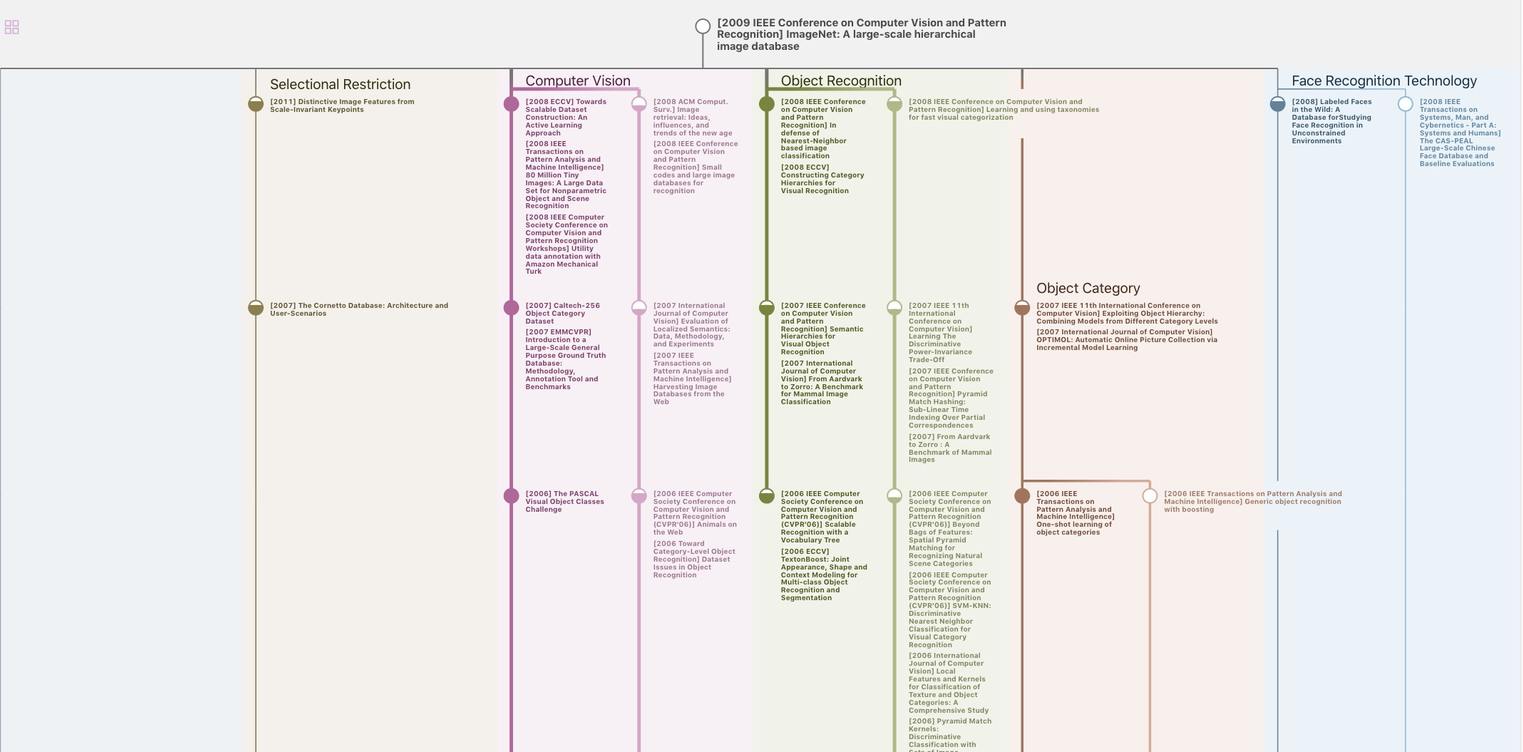
生成溯源树,研究论文发展脉络
Chat Paper
正在生成论文摘要