Decoupled neural network training with re-computation and weight prediction.
PloS one(2023)
摘要
To break the three lockings during backpropagation (BP) process for neural network training, multiple decoupled learning methods have been investigated recently. These methods either lead to significant drop in accuracy performance or suffer from dramatic increase in memory usage. In this paper, a new form of decoupled learning, named decoupled neural network training scheme with re-computation and weight prediction (DTRP) is proposed. In DTRP, a re-computation scheme is adopted to solve the memory explosion problem, and a weight prediction scheme is proposed to deal with the weight delay caused by re-computation. Additionally, a batch compensation scheme is developed, allowing the proposed DTRP to run faster. Theoretical analysis shows that DTRP is guaranteed to converge to crical points under certain conditions. Experiments are conducted by training various convolutional neural networks on several classification datasets, showing comparable or better results than the state-of-the-art methods and BP. These experiments also reveal that adopting the proposed method, the memory explosion problem is effectively solved, and a significant acceleration is achieved.
更多查看译文
关键词
neural network training,neural network,prediction,re-computation
AI 理解论文
溯源树
样例
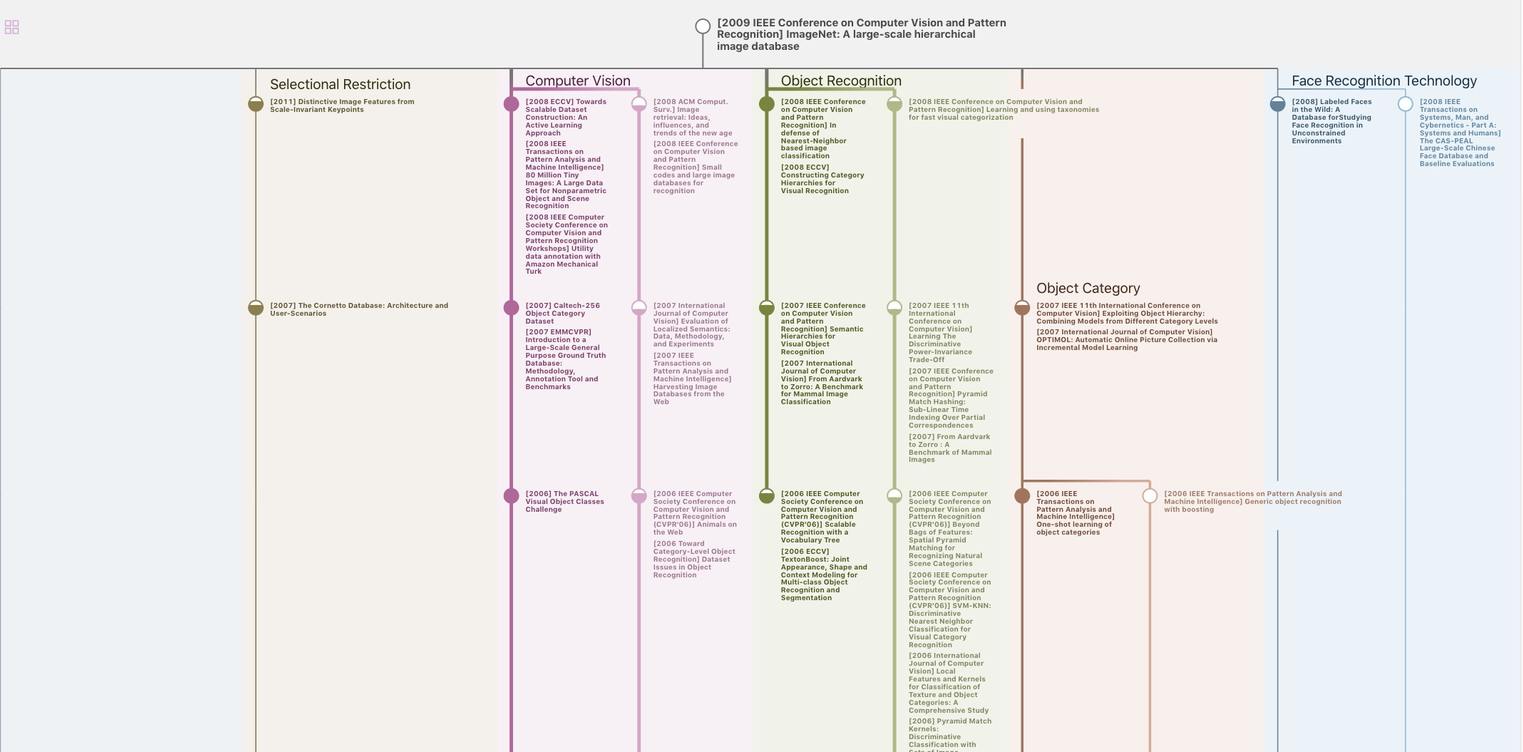
生成溯源树,研究论文发展脉络
Chat Paper
正在生成论文摘要