Identifying key multifunctional components shared by critical cancer and normal liver pathways via SparseGMM.
Cell reports methods(2023)
摘要
Despite the abundance of multimodal data, suitable statistical models that can improve our understanding of diseases with genetic underpinnings are challenging to develop. Here, we present SparseGMM, a statistical approach for gene regulatory network discovery. SparseGMM uses latent variable modeling with sparsity constraints to learn Gaussian mixtures from multiomic data. By combining coexpression patterns with a Bayesian framework, SparseGMM quantitatively measures confidence in regulators and uncertainty in target gene assignment by computing gene entropy. We apply SparseGMM to liver cancer and normal liver tissue data and evaluate discovered gene modules in an independent single-cell RNA sequencing (scRNA-seq) dataset. SparseGMM identifies PROCR as a regulator of angiogenesis and PDCD1LG2 and HNF4A as regulators of immune response and blood coagulation in cancer. Furthermore, we show that more genes have significantly higher entropy in cancer compared with normal liver. Among high-entropy genes are key multifunctional components shared by critical pathways, including p53 and estrogen signaling.
更多查看译文
关键词
normal liver pathways,critical cancer,normal liver
AI 理解论文
溯源树
样例
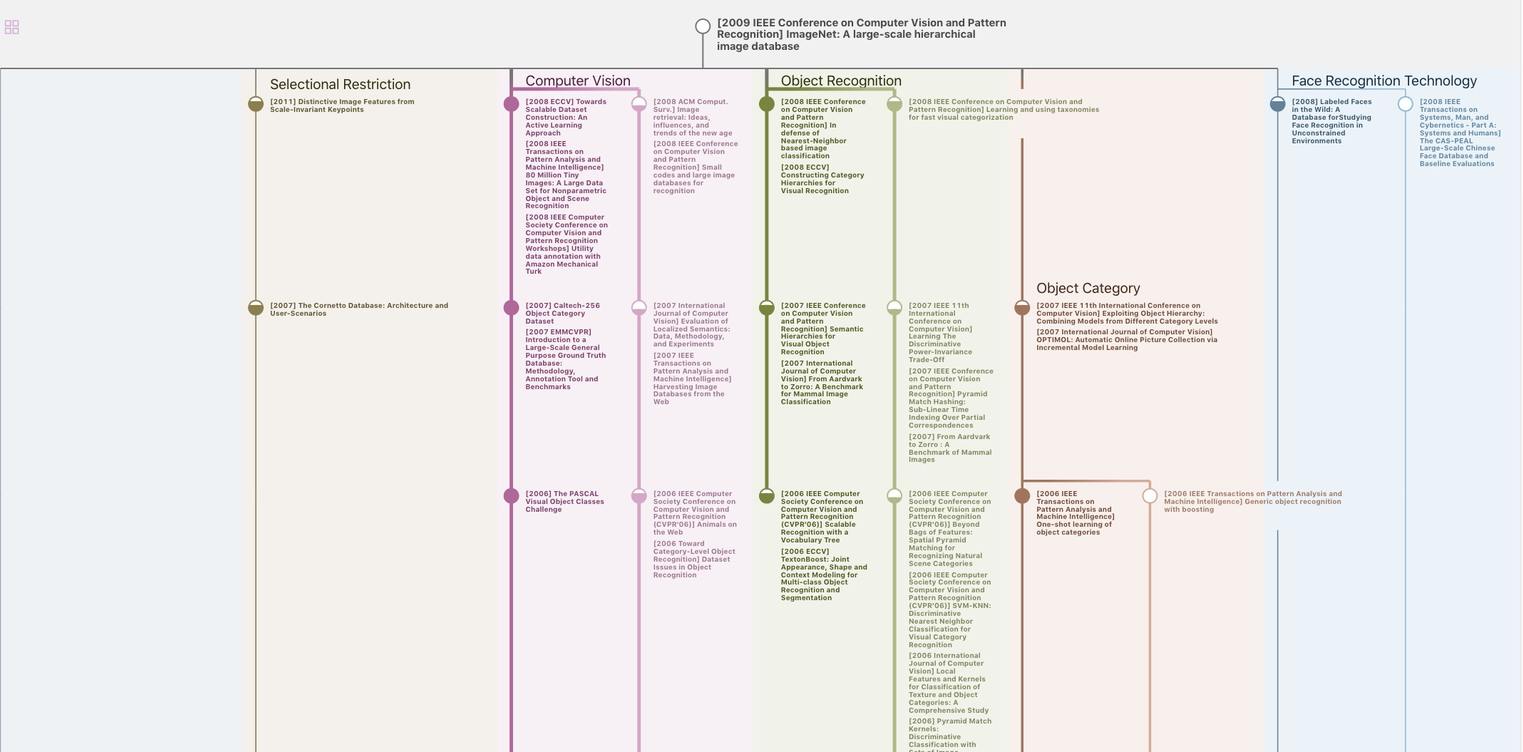
生成溯源树,研究论文发展脉络
Chat Paper
正在生成论文摘要