Boosting Nystr\"{o}m Method
arxiv(2023)
摘要
The Nystr\"{o}m method is an effective tool to generate low-rank approximations of large matrices, and it is particularly useful for kernel-based learning. To improve the standard Nystr\"{o}m approximation, ensemble Nystr\"{o}m algorithms compute a mixture of Nystr\"{o}m approximations which are generated independently based on column resampling. We propose a new family of algorithms, boosting Nystr\"{o}m, which iteratively generate multiple ``weak'' Nystr\"{o}m approximations (each using a small number of columns) in a sequence adaptively - each approximation aims to compensate for the weaknesses of its predecessor - and then combine them to form one strong approximation. We demonstrate that our boosting Nystr\"{o}m algorithms can yield more efficient and accurate low-rank approximations to kernel matrices. Improvements over the standard and ensemble Nystr\"{o}m methods are illustrated by simulation studies and real-world data analysis.
更多查看译文
关键词
method
AI 理解论文
溯源树
样例
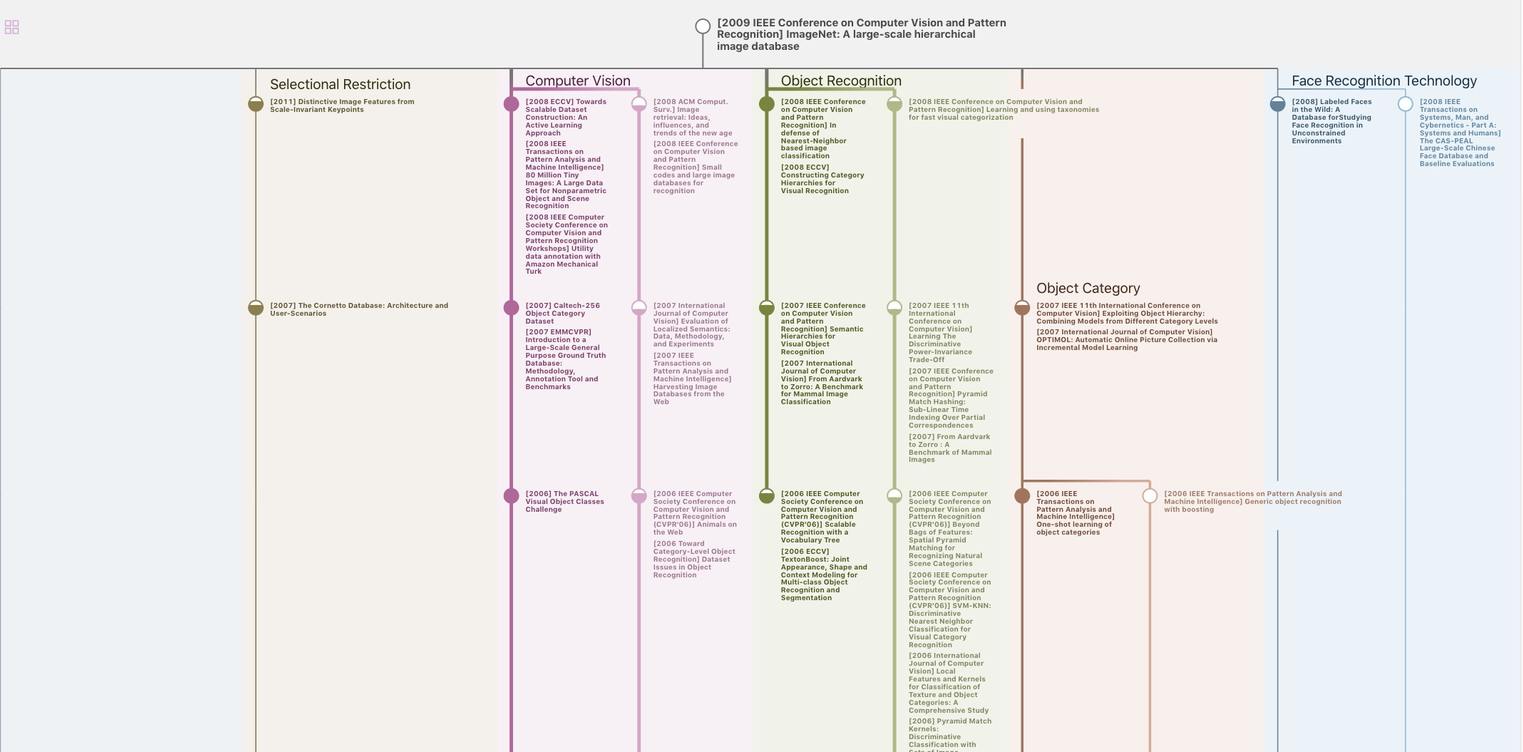
生成溯源树,研究论文发展脉络
Chat Paper
正在生成论文摘要