Learning physical models that can respect conservation laws
PHYSICA D-NONLINEAR PHENOMENA(2024)
摘要
Recent work in scientific machine learning (SciML) has focused on incorporating partial differential equation (PDE) information into the learning process. Much of this work has focused on relatively "easy"PDE operators (e.g., elliptic and parabolic), with less emphasis on relatively "hard"PDE operators (e.g., hyperbolic). Within numerical PDEs, the latter problem class requires control of a type of volume element or conservation constraint, which is known to be challenging. Delivering on the promise of SciML requires seamlessly incorporating both types of problems into the learning process. To address this issue, we propose PROBCONSERV, a framework for incorporating conservation constraints into a generic SciML architecture. To do so, PROBCONSERV combines the integral form of a conservation law with a Bayesian update. We provide a detailed analysis of PROBCONSERV on learning with the Generalized Porous Medium Equation (GPME), a widely-applicable parameterized family of PDEs that illustrates the qualitative properties of both easier and harder PDEs. PROBCONSERV is effective for easy GPME variants, performing well with state-of-the-art competitors; and for harder GPME variants it outperforms other approaches that do not guarantee volume conservation. PROBCONSERV seamlessly enforces physical conservation constraints, maintains probabilistic uncertainty quantification (UQ), and deals well with shocks and heteroscedasticities. In each case, it achieves superior predictive performance on downstream tasks.
更多查看译文
关键词
Scientific machine learning,Conservation laws,Physically constrained machine learning,Partial differential equations,Uncertainty quantification,Shock location detection
AI 理解论文
溯源树
样例
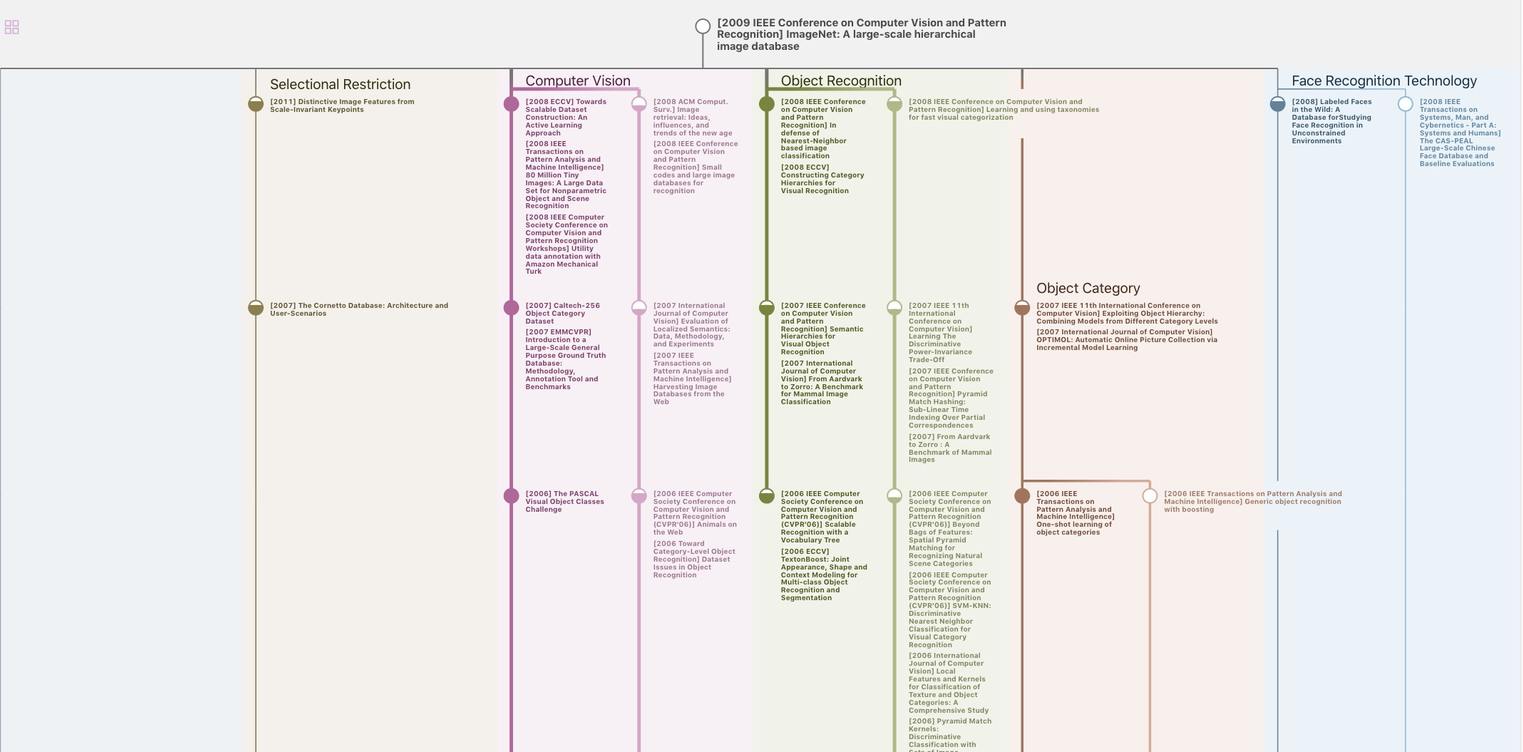
生成溯源树,研究论文发展脉络
Chat Paper
正在生成论文摘要