Physics-Informed Long Short-Term Memory for Forecasting and Reconstruction of Chaos
arxiv(2023)
摘要
We present the Physics-Informed Long Short-Term Memory (PI-LSTM) network to reconstruct and predict the evolution of unmeasured variables in a chaotic system. The training is constrained by a regularization term, which penalizes solutions that violate the system's governing equations. The network is showcased on the Lorenz-96 model, a prototypical chaotic dynamical system, for a varying number of variables to reconstruct. First, we show the PI-LSTM architecture and explain how to constrain the differential equations, which is a non-trivial task in LSTMs. Second, the PI-LSTM is numerically evaluated in the long-term autonomous evolution to study its ergodic properties. We show that it correctly predicts the statistics of the unmeasured variables, which cannot be achieved without the physical constraint. Third, we compute the Lyapunov exponents of the network to infer the key stability properties of the chaotic system. For reconstruction purposes, adding the physics-informed loss qualitatively enhances the dynamical behaviour of the network, compared to a data-driven only training. This is quantified by the agreement of the Lyapunov exponents. This work opens up new opportunities for state reconstruction and learning of the dynamics of nonlinear systems.
更多查看译文
关键词
forecasting,chaos,memory,physics-informed,short-term
AI 理解论文
溯源树
样例
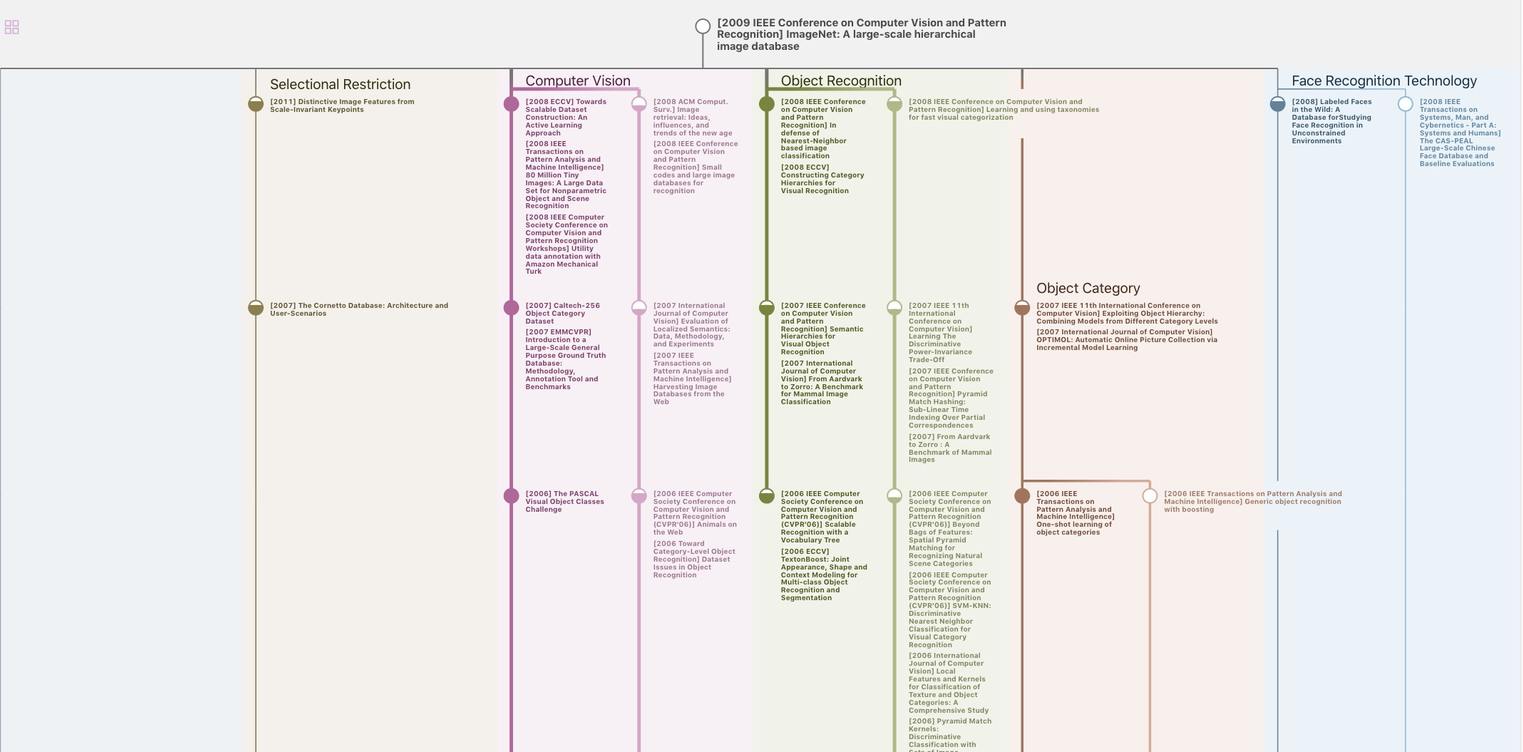
生成溯源树,研究论文发展脉络
Chat Paper
正在生成论文摘要