LU-Net: Invertible Neural Networks Based on Matrix Factorization
2023 INTERNATIONAL JOINT CONFERENCE ON NEURAL NETWORKS, IJCNN(2023)
摘要
LU-Net is a simple and fast architecture for invertible neural networks (INN) that is based on the factorization of quadratic weight matrices A=LU, where L is a lower triangular matrix with ones on the diagonal and U an upper triangular matrix. Instead of learning a fully occupied matrix A, we learn L and U separately. If combined with an invertible activation function, such layers can easily be inverted whenever the diagonal entries of U are different from zero. Also, the computation of the determinant of the Jacobian matrix of such layers is cheap. Consequently, the LU architecture allows for cheap computation of the likelihood via the change of variables formula and can be trained according to the maximum likelihood principle. In our numerical experiments, we test the LU-Net architecture as generative model on several academic datasets. We also provide a detailed comparison with conventional invertible neural networks in terms of performance, training as well as run time.
更多查看译文
AI 理解论文
溯源树
样例
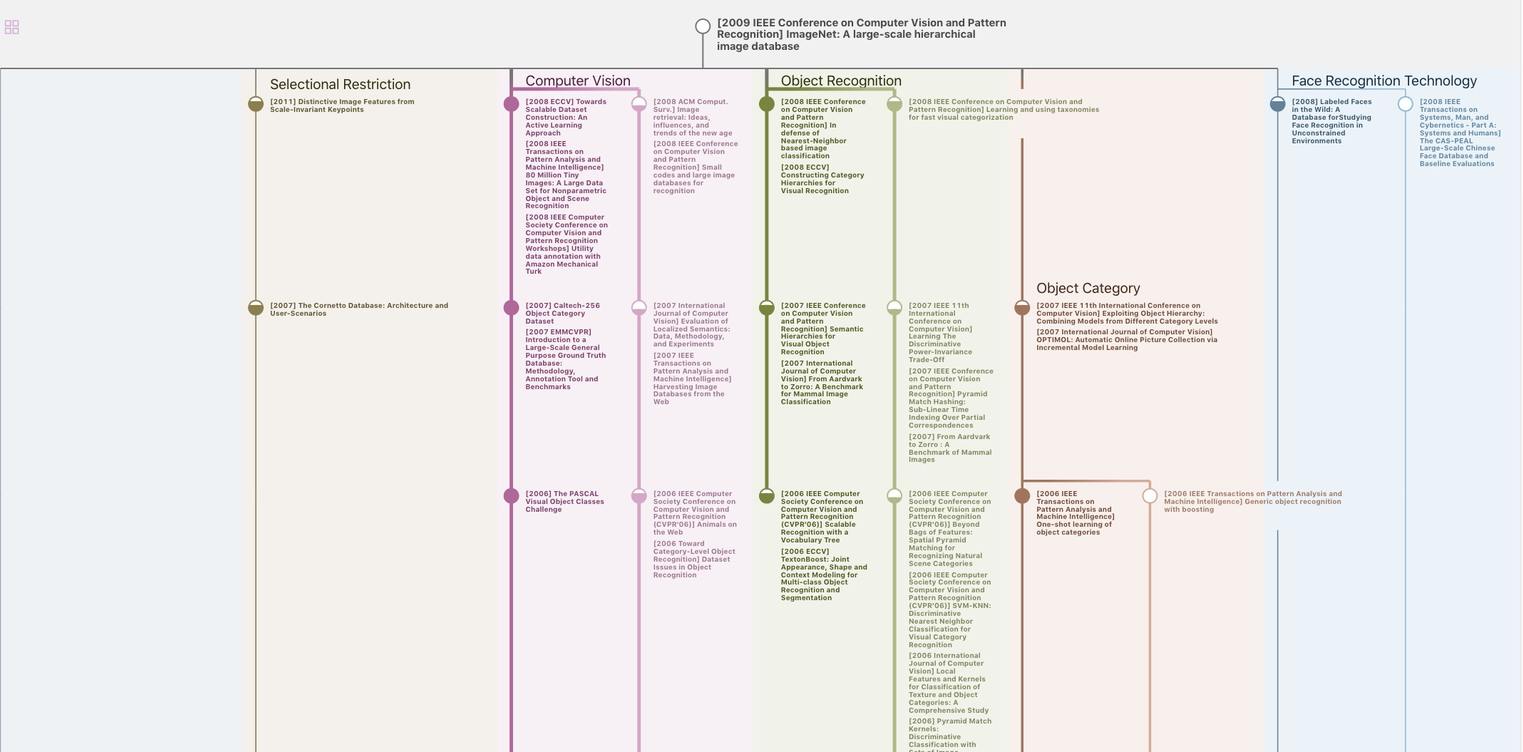
生成溯源树,研究论文发展脉络
Chat Paper
正在生成论文摘要