HyperRS: Hypernetwork-Based Recommender System for the User Cold-Start Problem.
IEEE Access(2023)
摘要
Meta-learning has been proven to be effective for the cold-start problem of recommender systems. Many meta-learning recommender systems that are designed for the user cold-start problem are gradient-based. They use a global parameter learned from existing users to initialize the recommender system parameter for new users that provides a personalized recommendation with limited user-item interactions. Many systems require users' demographic information to learn the global parameter. This requirement raises privacy concerns, and demographic information is not always available. In addition, some of the gradient-based systems need dozens or even hundreds of user-item interactions from existing users to learn the global parameter. This requirement is difficult to satisfy in specific scenarios in which active users and their item interactions are rare. Moreover, gradient-based meta-learning systems rarely capture user preferences over different item attributes, as updating the corresponding weights requires many optimization steps. We proposed HyperRS, a Hypernetwork-based Recommender System, for the user cold-start problem. Our system does not rely on demographic information to provide personalized recommendations. In our system, a hypernetwork generates all weights in the underlying recommender system. The hypernetwork enables the weights to adapt quickly to capture user interest in both item attributes and the contents of the item attributes. The experimental results show that our method outperforms several state-of-the-art meta-learning recommender systems for the user cold-start problem.
更多查看译文
关键词
Recommender systems,Neural networks,Optimization,Privacy,Training data,Logic gates,Meta-learning,Meta-Learning,recommender system,hypernetwork,cold-start problem
AI 理解论文
溯源树
样例
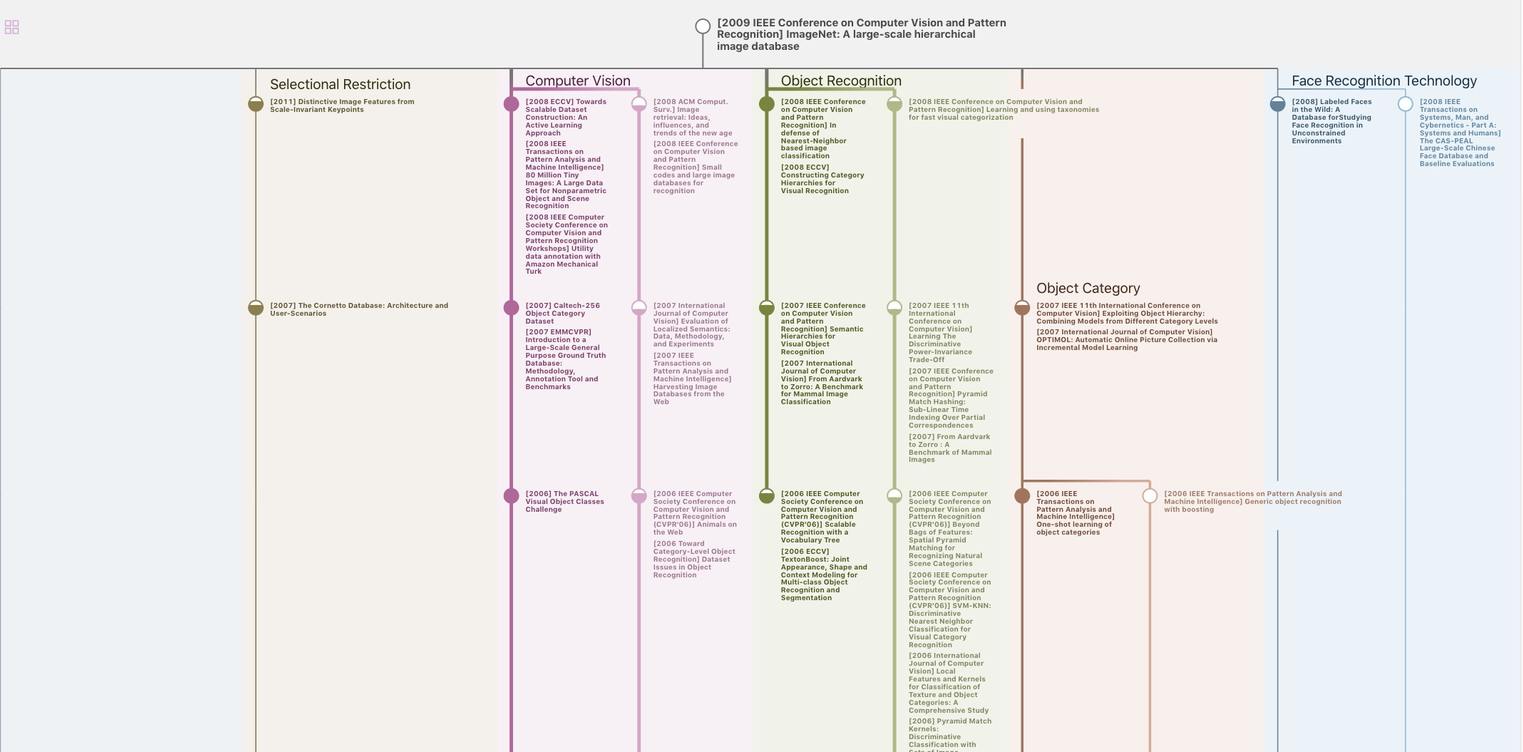
生成溯源树,研究论文发展脉络
Chat Paper
正在生成论文摘要