Balanced masking strategy for multi-label image classification
Neurocomputing(2023)
摘要
Data imbalance is an essential issue in multi-label image classification that may reduce the model’s generalization ability. Unlike oversampling or undersampling in single-label image classification, simply removing or repeating some images in multi-label classification will influence labels in other categories due to complex label dependencies. Current methods usually tackle this issue from either data resampling or loss function design. This paper proposes a balanced masking strategy to solve the imbalance problem in feature space for graph-based methods. By selectively manipulating the label node embedding of the graph, the model can gain the priors of the majority of samples to maintain intra-class and inter-class balance simultaneously, thus performing better and more stable. Extensive experiments verify the adaptability and effectiveness of our method, which can be easily applied to any graph-based multi-label model to achieve highly competitive performance.
更多查看译文
关键词
Multi-label image classification,Data imbalance,Masking strategy,Graph neural network
AI 理解论文
溯源树
样例
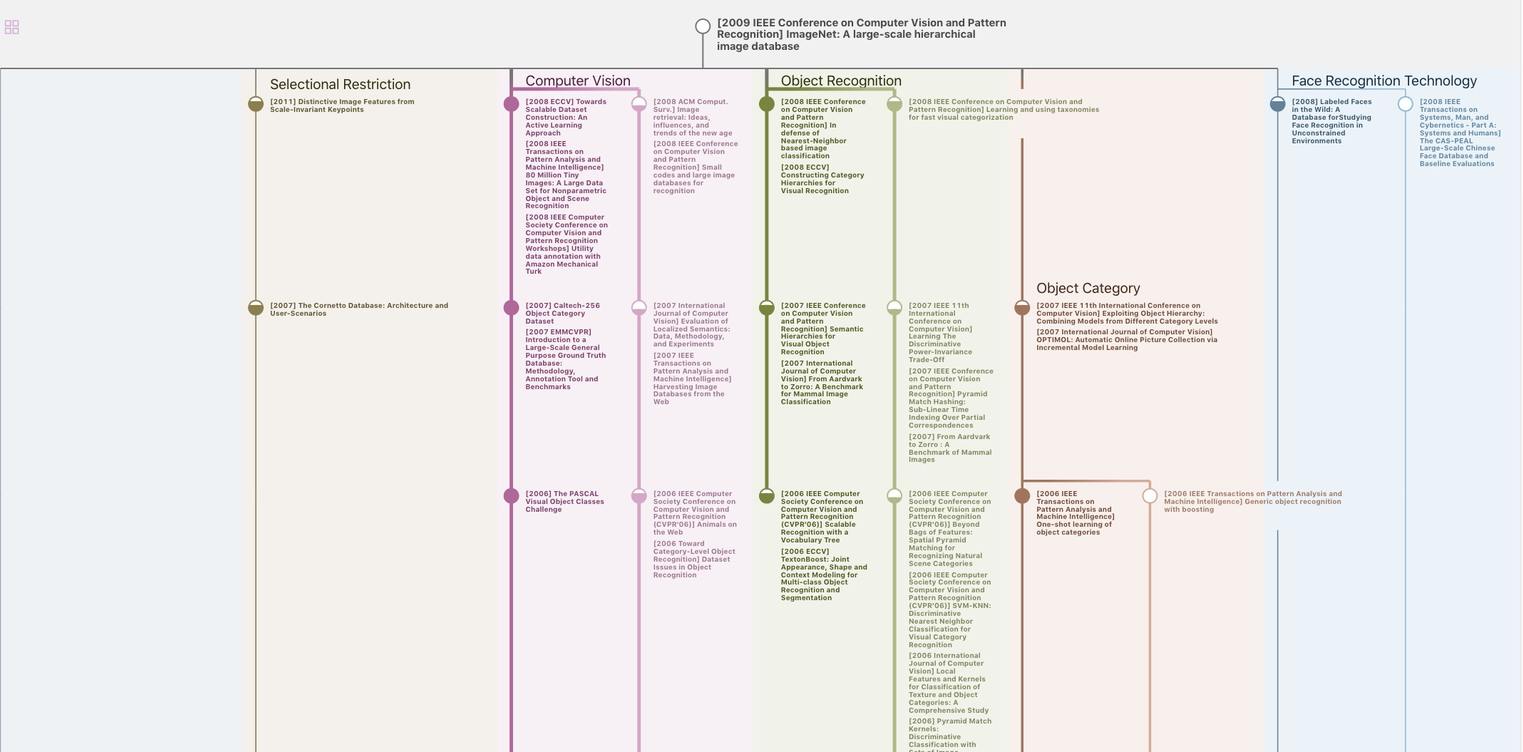
生成溯源树,研究论文发展脉络
Chat Paper
正在生成论文摘要