On stability and regularization for data-driven solution of parabolic inverse source problems
Journal of Computational Physics(2023)
摘要
The diffusion process from some internal source arising in engineering situations can be mathematically described by a parabolic system. We consider an inverse source problem for parabolic system using parametric approximations, where deep neural networks (DNNs) are used to approximate the solution of the inverse problem. First, we prove generalization error estimates depending on training errors and data noise levels by establishing conditional stability of the inverse problem. Following our analysis, we propose a new loss function involving the derivative of the residuals for PDE and measurement data. These extra terms effectively induce higher regularity in solution to deal with the ill-posedness of the inverse problem. Using these regularization terms, we develop reconstruction schemes and demonstrate the effectiveness of our proposed methodology on a number of test problems.
更多查看译文
关键词
Data-driven,Deep neural networks,Inverse source problem,Generalization error estimates,Numerics
AI 理解论文
溯源树
样例
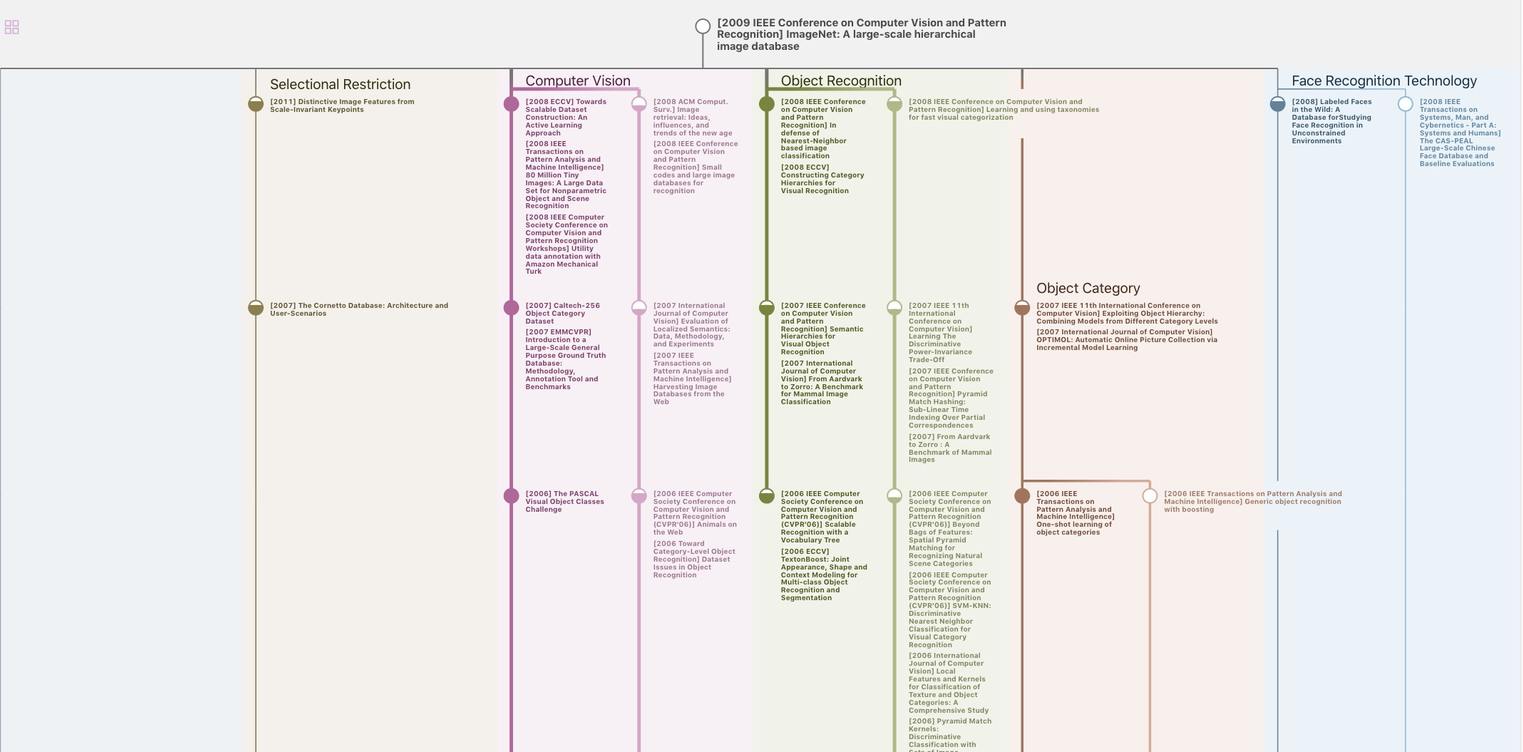
生成溯源树,研究论文发展脉络
Chat Paper
正在生成论文摘要