A federated feature selection algorithm based on particle swarm optimization under privacy protection
Knowledge-Based Systems(2023)
摘要
Feature selection is an important preprocessing technique in the fields of data mining and machine learning. With the promotion of privacy protection awareness, recently it becomes a very practical and challenging issue to select high-quality feature subsets while ensuring the privacy of all participants. However, there is a lack of research results on this issue, i.e., feature selection under privacy protection. Aiming at the issue, this paper proposes a federated feature selection framework for the first time. In the framework, inspiring by the idea of federated learning, a credible third participant is introduced to process and integrate optimal feature subsets from multiple participants. On the basis of the framework, a federated evolutionary feature selection algorithm based on particle swarm optimization is proposed to effectively solve feature selection problems with multiple participants under privacy protection. Two new operators satisfying the requirement of privacy protection, i.e., the feature assembling strategy with multi-participant cooperation and the swarm initialization strategy guided by assembling solution, are designed to improve the ability of the proposed algorithm. Compared with several typical assembling feature selection algorithms on 15 data sets, experimental results show that the proposed algorithm can significantly improve the classification accuracy of the feature subset selected by each participant, while protecting the privacy of data.
更多查看译文
关键词
00-01,99-00
AI 理解论文
溯源树
样例
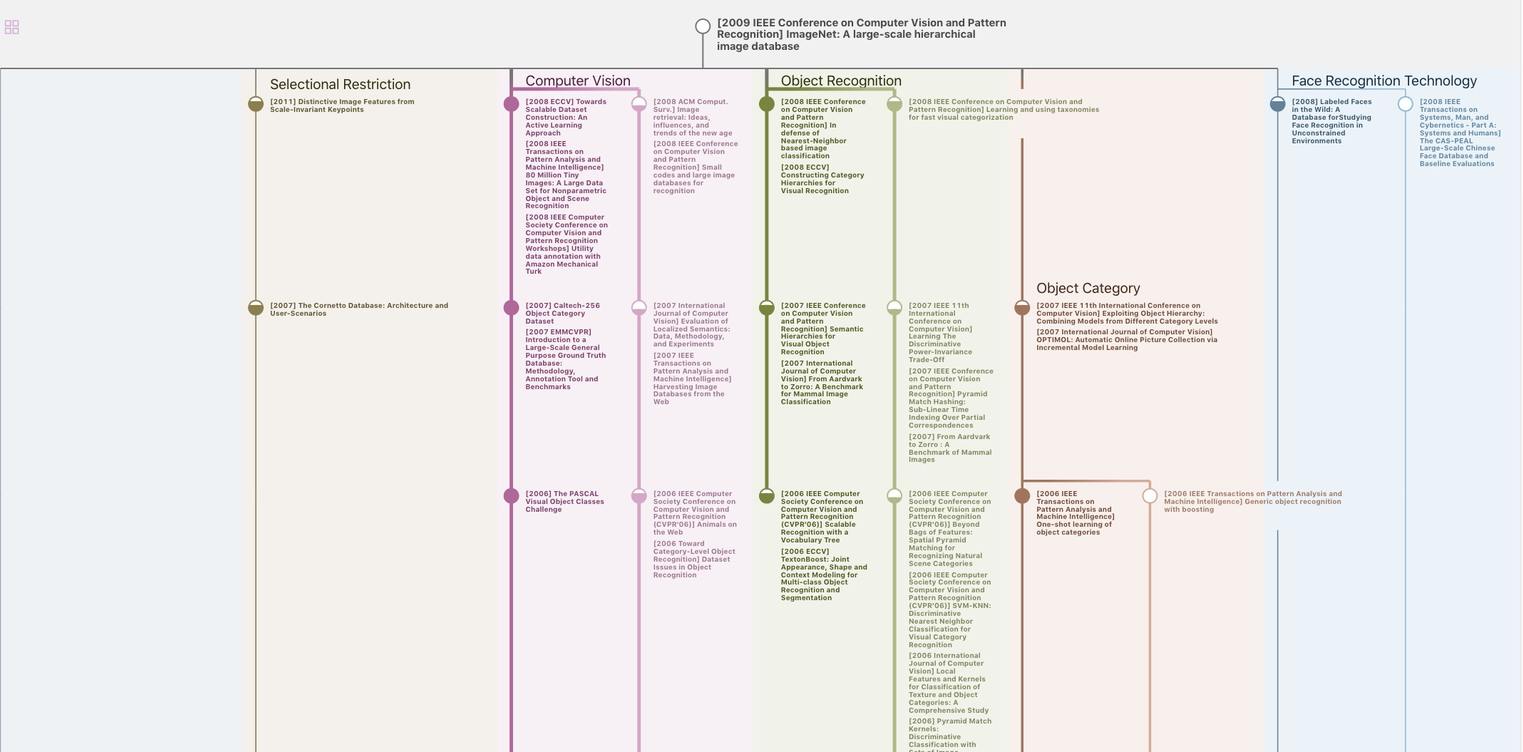
生成溯源树,研究论文发展脉络
Chat Paper
正在生成论文摘要