MATNet: Exploiting Multi-Modal Features for Radiology Report Generation.
IEEE Signal Process. Lett.(2022)
摘要
Medical imaging is widely used in hospital clinical workflows. Assisting physicians in diagnosis by automatically generating reports from radiological images is an unmet clinical demand and requires urgent attention. However, this task suffers from two significant problems: 1) visual and textual data biases, and 2) the Transformer decoder makes no distinction between visual and non-visual words. We propose a novel multi-task approach combining natural language processing with machine learning techniques to meet this clinical need, i.e., creating fluent and accurate radiology reports. We name our system as Multi-modal Adaptive Transformer (MATNet), which consists of three key modules. First, Multi-Modal Encoder (MME) explores the relationship between radiology images and clinical notes. Second, Disease Classifier (DC) classifies the states of each disease topic and provides state-aware disease embeddings to alleviate visual data bias. Last, Adaptive Decoder (AD) dynamically measures the contribution of source signals and target signals when generating the next word. Based on our evaluations using benchmark IU-XRay and MIMIC-CXR datasets, the proposed MATNet outperformed previous state-of-the-art models on language fluency and clinical accuracy metrics such as BLEU scores.
更多查看译文
关键词
matnet,features,report,multi-modal
AI 理解论文
溯源树
样例
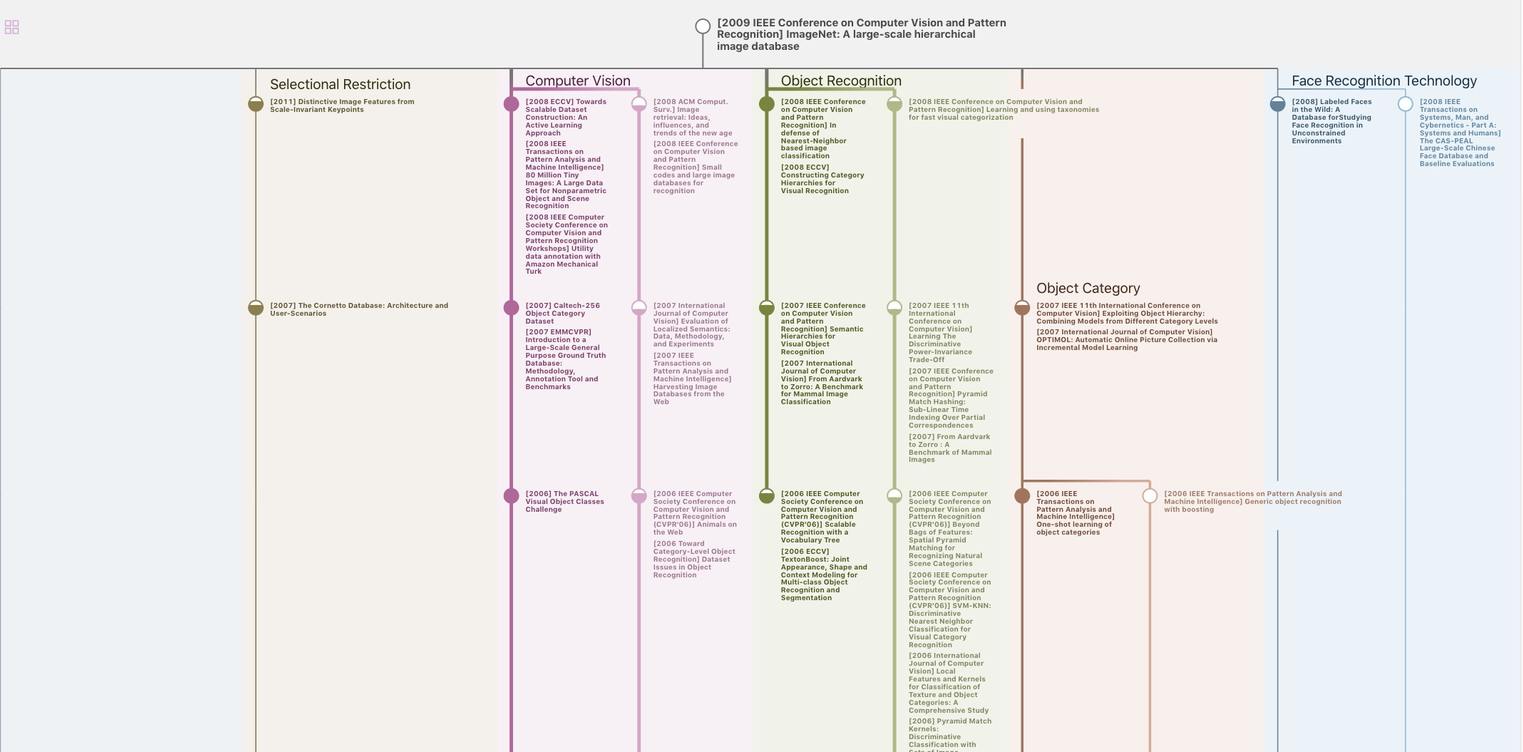
生成溯源树,研究论文发展脉络
Chat Paper
正在生成论文摘要