Learning dynamical systems from data: A simple cross-validation perspective, Part V: Sparse Kernel Flows for 132 chaotic dynamical systems
PHYSICA D-NONLINEAR PHENOMENA(2024)
摘要
Learning equations of complex dynamical systems from data is one of the principal problems in scientific machine learning. The method of Kernel Flows (KFs) has offered an effective learning strategy that interpolates the vector -field of dynamical system with a data -adapted kernel. It is based on the premise that a kernel is good if the number of interpolation points can be halved without significant loss in accuracy. However, KFs is limited by the choice of a base kernel. In this paper, we introduce the method of Sparse Kernel Flows in order to learn the "best"kernel starting from a preset kernel library. First, we design a parameterized base kernel that is a linear combination of several existing kernel functions. Then, under the assumption of KFs that a kernel is good if the number of interpolation points can be halved without significant loss in accuracy, we design the kernel learning loss function by incorporating l(1) regularization. Then, Least absolute shrinkage and selection operator (LASSO) is performed to extract the fewest active terms from the base overdetermined set of candidate kernel functions, thereby estimating the weight coefficient. Furthermore, we apply our proposal to a benchmark dataset including 132 chaotic dynamical systems.
更多查看译文
关键词
System approximation,Chaotic dynamical systems,Learning Kernels,Sparse Kernel Flows,LASSO
AI 理解论文
溯源树
样例
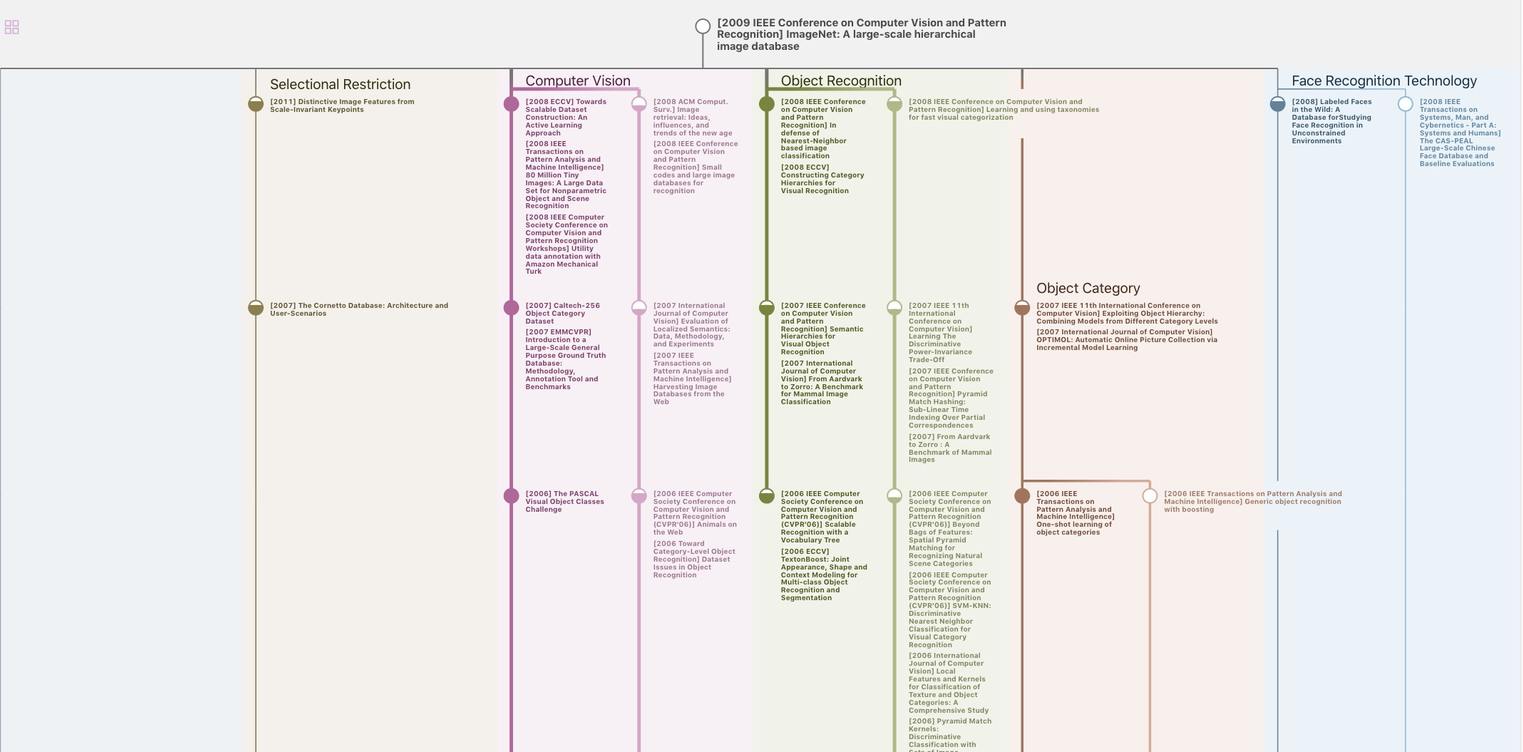
生成溯源树,研究论文发展脉络
Chat Paper
正在生成论文摘要