Enhancing Automatic Attack Detection through Spectral Decomposition of Network Flows
GLOBECOM(2022)
摘要
Flow classification employs machine learning techniques to identify attacks on computer networks. This classification relies on quantitative features that synthesize the information of packets from the same flow. Conventional features, however, such as packet size and the number of bytes, generate redundancies and do not capture the temporal correlations between the packets in a flow. Automated network attacks generate periodic patterns observable through spectral decomposition, which facilitates classification. This paper proposes FENED (Feature Extraction by Network spEctrum Decomposition), a method to extract features from network data. We consider the packet-arrived order within the same flow using the fast Fourier transform for binary classification. The proposed feature vector contains the module of the spectral components of the flow. Experimental results show that FENED outperforms conventional proposals because it extracts features that consider intra-flow packet-arrival order.
更多查看译文
关键词
automatic attack detection,spectral decomposition
AI 理解论文
溯源树
样例
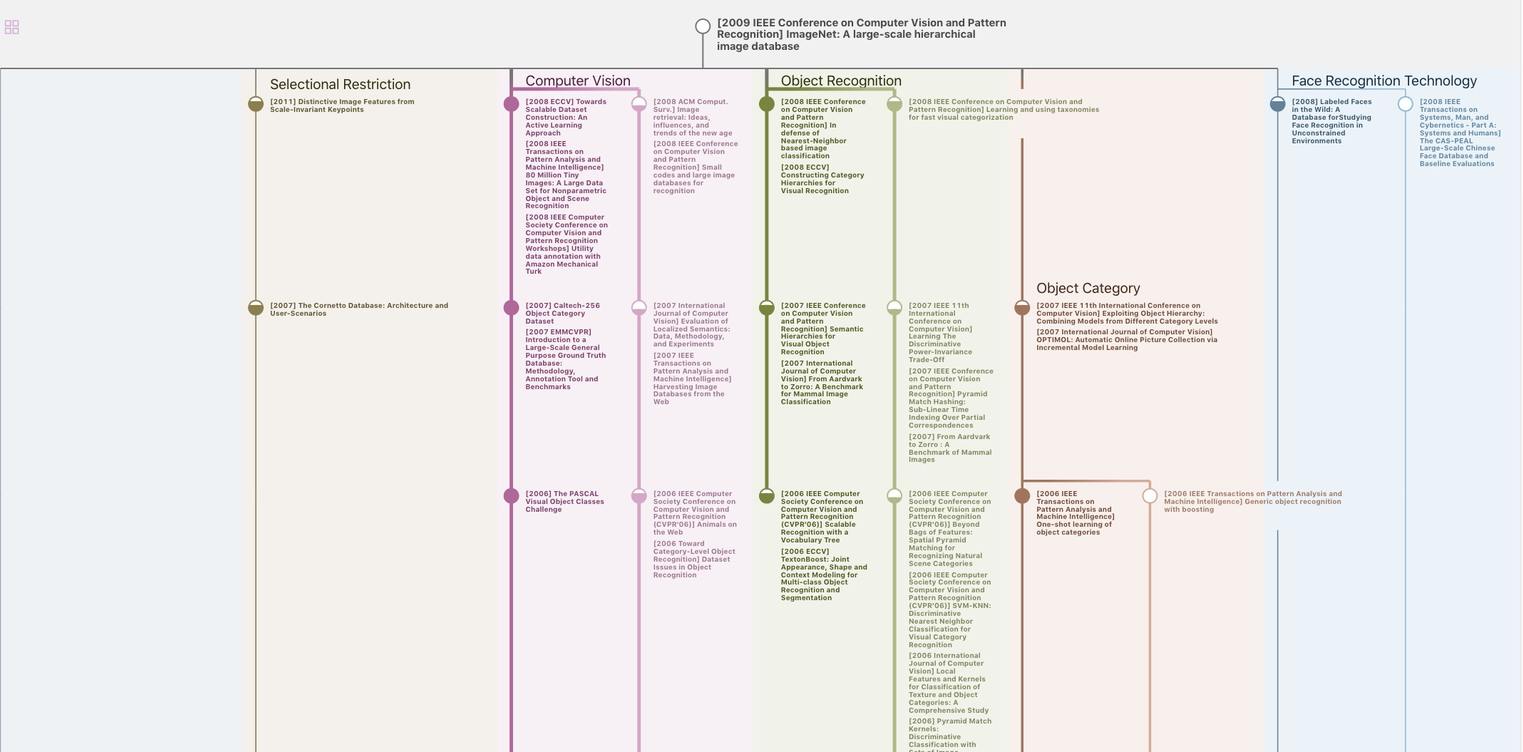
生成溯源树,研究论文发展脉络
Chat Paper
正在生成论文摘要