Communication-Efficient Federated Bayesian Learning via Client Selection.
GLOBECOM (Workshops)(2022)
摘要
Distributed Stein Variational Gradient Descent (DSVGD) is a non-parametric distributed learning framework for federated Bayesian learning, where multiple clients jointly train a machine learning model by communicating a number of non-random and interacting particles with the server. Since communication resources are limited, selecting the clients with most informative local learning updates can improve the model convergence and communication efficiency. In this paper, we propose a client selection scheme for DSVGD based on Hilbert Inner Product (HIP). We derive the upper bound on the decrease of the global free energy per iteration, which is then minimized to speed up the model convergence. We evaluate and compare our scheme with conventional schemes in terms of model accuracy, convergence speed, and stability using various learning tasks and datasets.
更多查看译文
关键词
client,learning,selection,communication-efficient
AI 理解论文
溯源树
样例
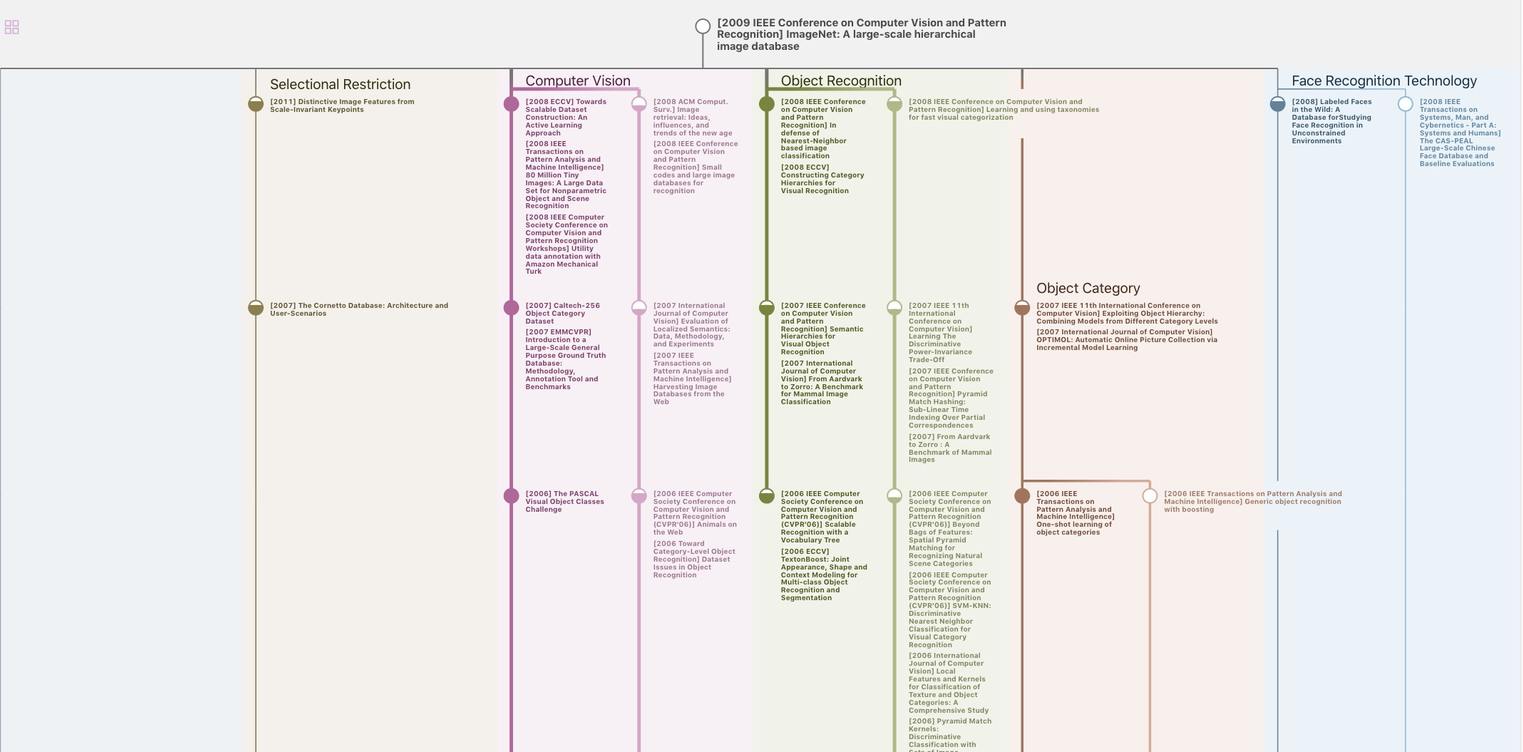
生成溯源树,研究论文发展脉络
Chat Paper
正在生成论文摘要