Intrinsic Imaging Model Enhanced Contrastive Face Representation Learning
2023 IEEE 17th International Conference on Automatic Face and Gesture Recognition (FG)(2023)
摘要
Humans can easily perceive numerous information from faces, only part of which has been achieved by a machine, thanks to the availability of large-scale face images with supervision signals of those specific tasks. More face perception tasks, like rare expression or attribute recognition, and genetic syndrome diagnosis, are not solved due to a critical shortage of supervised data. One possible way to solve these tasks is leveraging ubiquitous large-scale unsupervised face images and building a foundation face model via methods like contrastive learning (CL), which is, however, not aware of the intrinsic physics of the human face. In consideration of this shortcoming, this paper proposes to enhance contrastive face representation learning by the physical imaging model. Specifically, besides the CL-backbone network, we also design an auxiliary bypass pathway to constrain the CL-backbone to support the ability of accurately re-rendering the face with a differentiable physical imaging model after decomposing an input face image into intrinsic 3D imaging factors. With this design, the CL network is endowed the capacity of implicitly “knowing” the 3D of the face rather than the 2D pixels only. In experiments, we learn face representations from the CelebA and WebFace-42M datasets in unsupervised mode and evaluate the generalization capability of the representations with three different downstream tasks in case of limited supervised data. The experimental results clearly justify the effectiveness of the proposed method.
更多查看译文
AI 理解论文
溯源树
样例
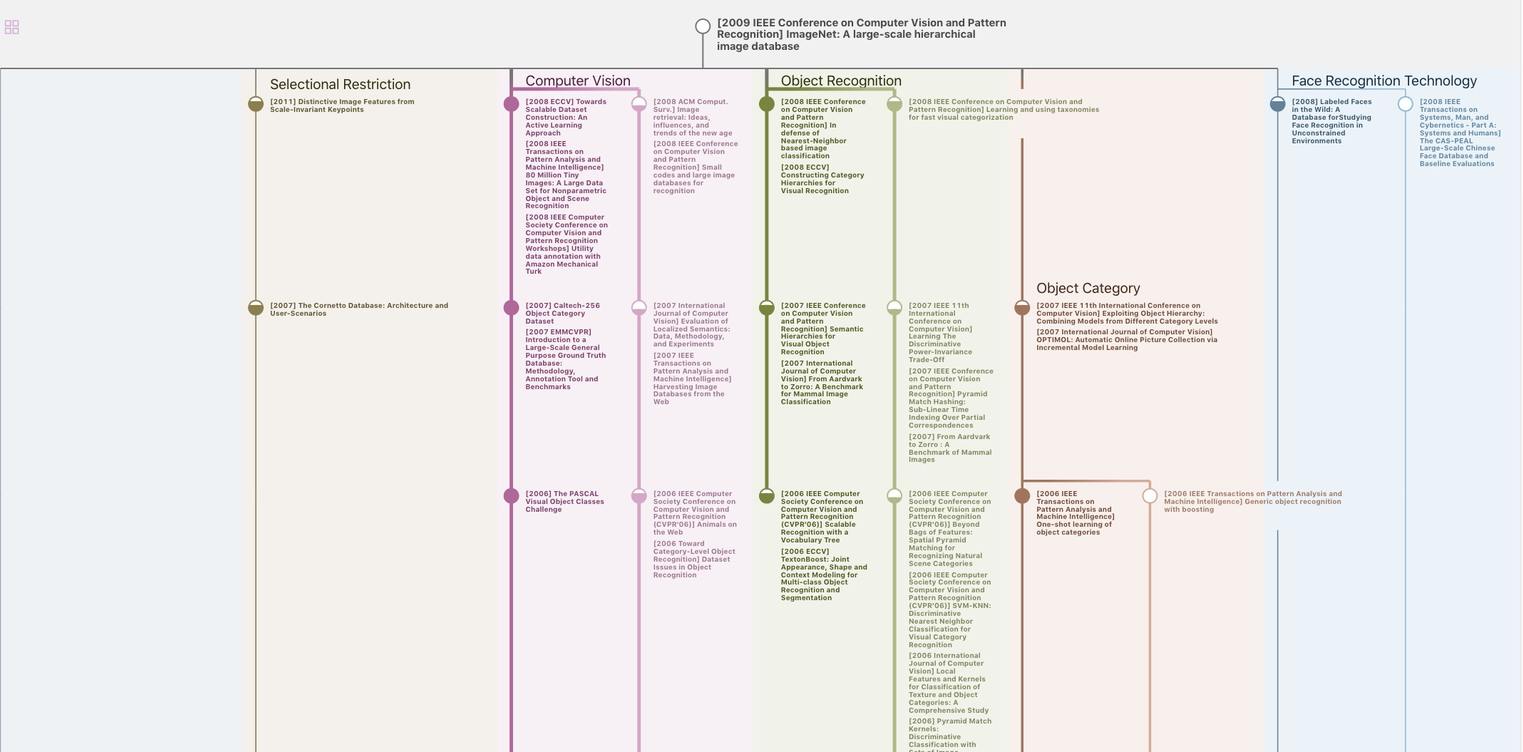
生成溯源树,研究论文发展脉络
Chat Paper
正在生成论文摘要