Tensorial parametric model order reduction of nonlinear dynamical systems
arxiv(2023)
摘要
For a nonlinear dynamical system depending on parameters the paper introduces a novel tensorial reduced order model (TROM). The reduced model is projection-based and for systems with no parameters involved it resembles the proper orthogonal decomposition (POD) combined with the discrete empirical interpolation method (DEIM). For parametric systems, the TROM employs low-rank tensor approximations in place of truncated SVD, a key dimension-reduction technique in POD with DEIM. Three popular low-rank tensor compression formats are considered for this purpose: canonical polyadic, Tucker, and tensor train. The use of multi-linear algebra tools allows to incorporate the information about the parameter dependence of the system into the reduced model and leads to a POD--DEIM type ROM which (i) is parameter-specific (localized) and predicts the system dynamics for out-of-training set (unseen) parameter values, (ii) mitigates the adverse effects of high parameter space dimension, (iii) has online computational costs that depend only on tensor compression ranks but not on the full order model size, and (iv) achieves lower reduced space dimensions compared to the conventional POD--DEIM ROM. The paper explains the method, analyzes its prediction power, and assesses its performance for two specific parameter-dependent non-linear dynamical systems.
更多查看译文
关键词
dynamical
AI 理解论文
溯源树
样例
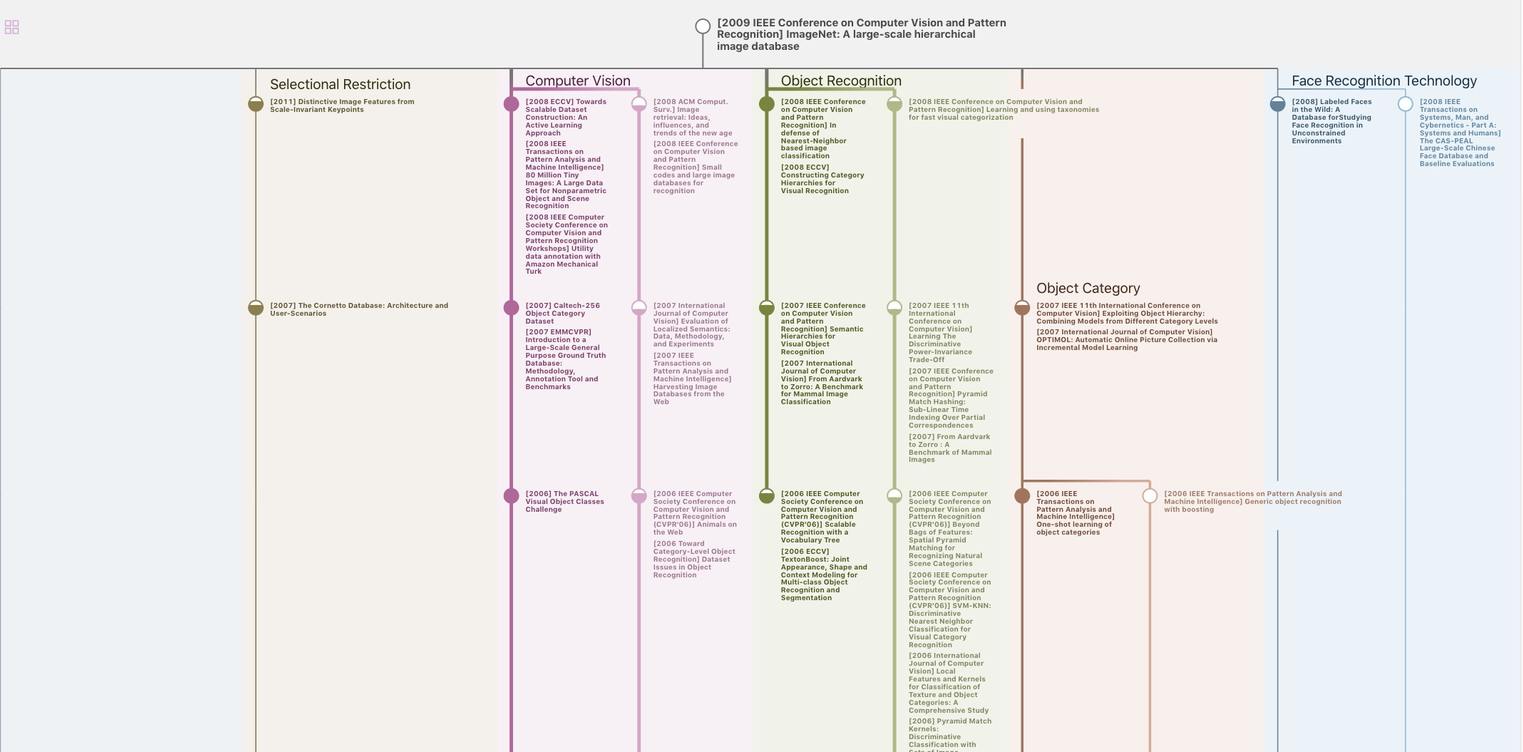
生成溯源树,研究论文发展脉络
Chat Paper
正在生成论文摘要