Learning From Biased Soft Labels
CoRR(2023)
摘要
Knowledge distillation has been widely adopted in a variety of tasks and has achieved remarkable successes. Since its inception, many researchers have been intrigued by the dark knowledge hidden in the outputs of the teacher model. Recently, a study has demonstrated that knowledge distillation and label smoothing can be unified as learning from soft labels. Consequently, how to measure the effectiveness of the soft labels becomes an important question. Most existing theories have stringent constraints on the teacher model or data distribution, and many assumptions imply that the soft labels are close to the ground-truth labels. This paper studies whether biased soft labels are still effective. We present two more comprehensive indicators to measure the effectiveness of such soft labels. Based on the two indicators, we give sufficient conditions to ensure biased soft label based learners are classifier-consistent and ERM learnable. The theory is applied to three weakly-supervised frameworks. Experimental results validate that biased soft labels can also teach good students, which corroborates the soundness of the theory.
更多查看译文
关键词
biased soft labels,learning
AI 理解论文
溯源树
样例
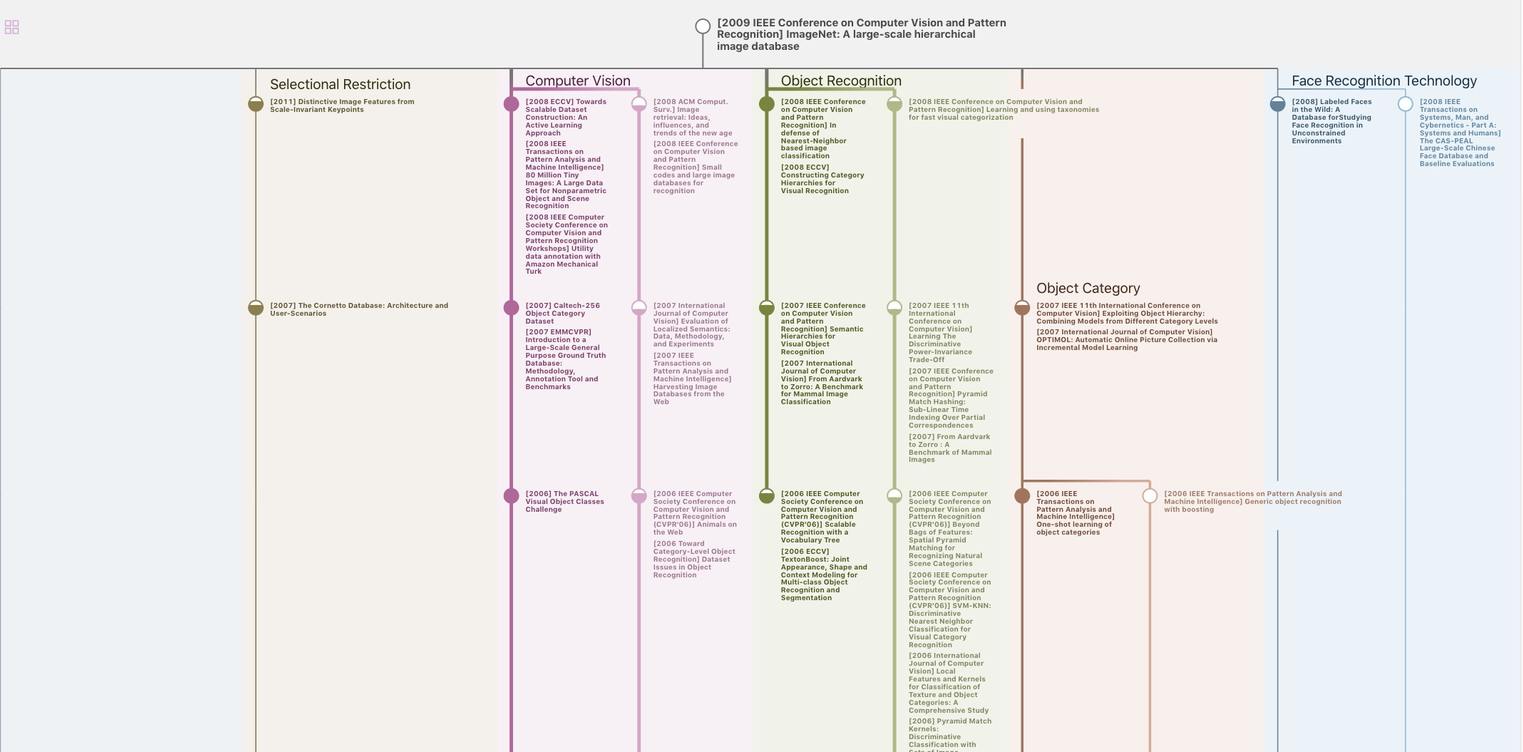
生成溯源树,研究论文发展脉络
Chat Paper
正在生成论文摘要