Empirical Investigation of Neural Symbolic Reasoning Strategies
arxiv(2023)
摘要
Neural reasoning accuracy improves when generating intermediate reasoning steps. However, the source of this improvement is yet unclear. Here, we investigate and factorize the benefit of generating intermediate steps for symbolic reasoning. Specifically, we decompose the reasoning strategy w.r.t. step granularity and chaining strategy. With a purely symbolic numerical reasoning dataset (e.g., A=1, B=3, C=A+3, C?), we found that the choice of reasoning strategies significantly affects the performance, with the gap becoming even larger as the extrapolation length becomes longer. Surprisingly, we also found that certain configurations lead to nearly perfect performance, even in the case of length extrapolation. Our results indicate the importance of further exploring effective strategies for neural reasoning models.
更多查看译文
关键词
neural symbolic reasoning strategies,empirical investigation
AI 理解论文
溯源树
样例
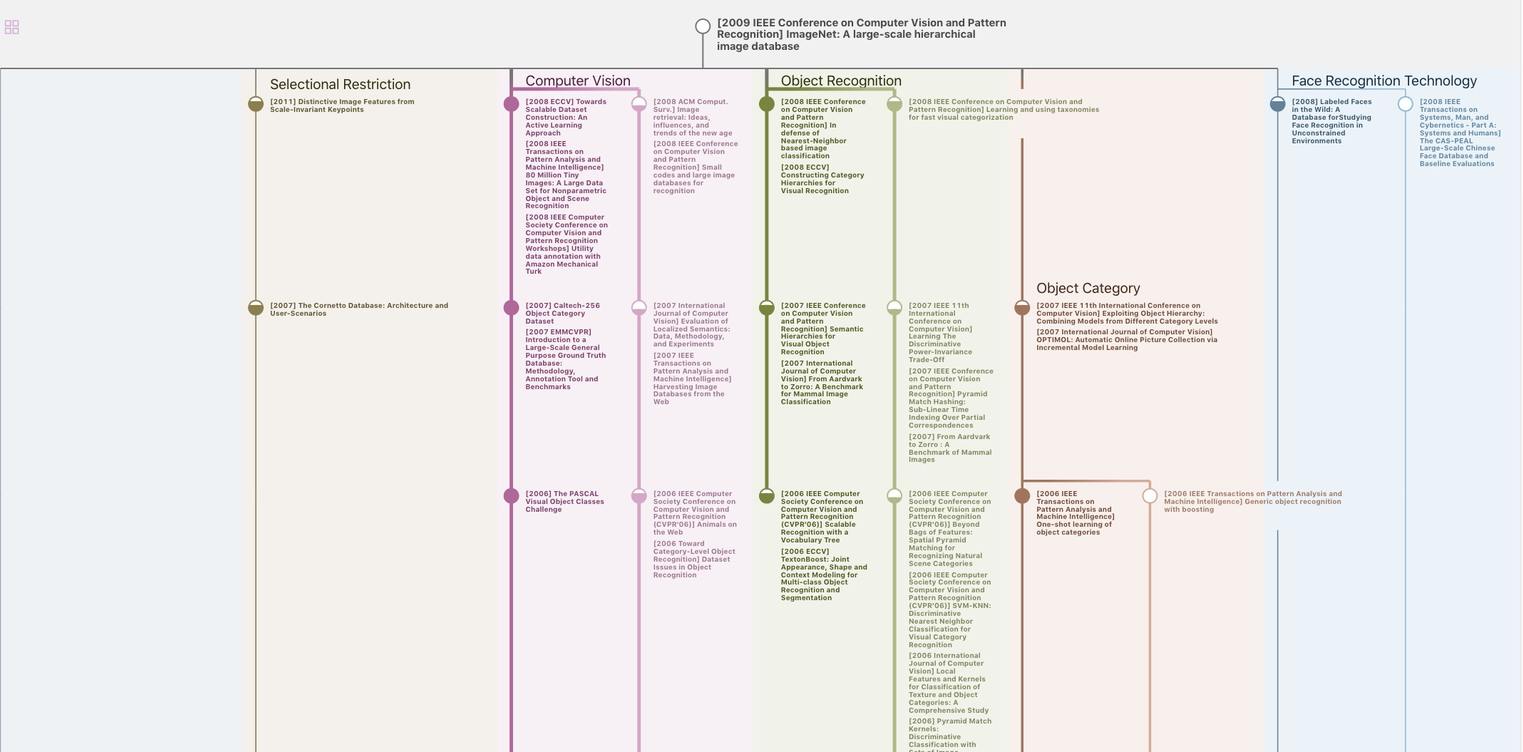
生成溯源树,研究论文发展脉络
Chat Paper
正在生成论文摘要