Difference-based Deep Convolutional Neural Network for Simulation-to-reality UAV Fault Diagnosis
arxiv(2023)
摘要
Identifying the fault in propellers is important to keep quadrotors operating safely and efficiently. The simulation-to-reality (sim-to-real) UAV fault diagnosis methods provide a cost-effective and safe approach to detect the propeller faults. However, due to the gap between simulation and reality, classifiers trained with simulated data usually underperform in real flights. In this work, a new deep neural network (DNN) model is presented to address the above issue. It uses the difference features extracted by deep convolutional neural networks (DDCNN) to reduce the sim-to-real gap. Moreover, a new domain adaptation method is presented to further bring the distribution of the real-flight data closer to that of the simulation data. The experimental results show that the proposed approach can achieve an accuracy of 97.9\% in detecting propeller faults in real flight. Feature visualization was performed to help better understand our DDCNN model.
更多查看译文
关键词
deep convolutional neural network,difference-based,simulation-to-reality
AI 理解论文
溯源树
样例
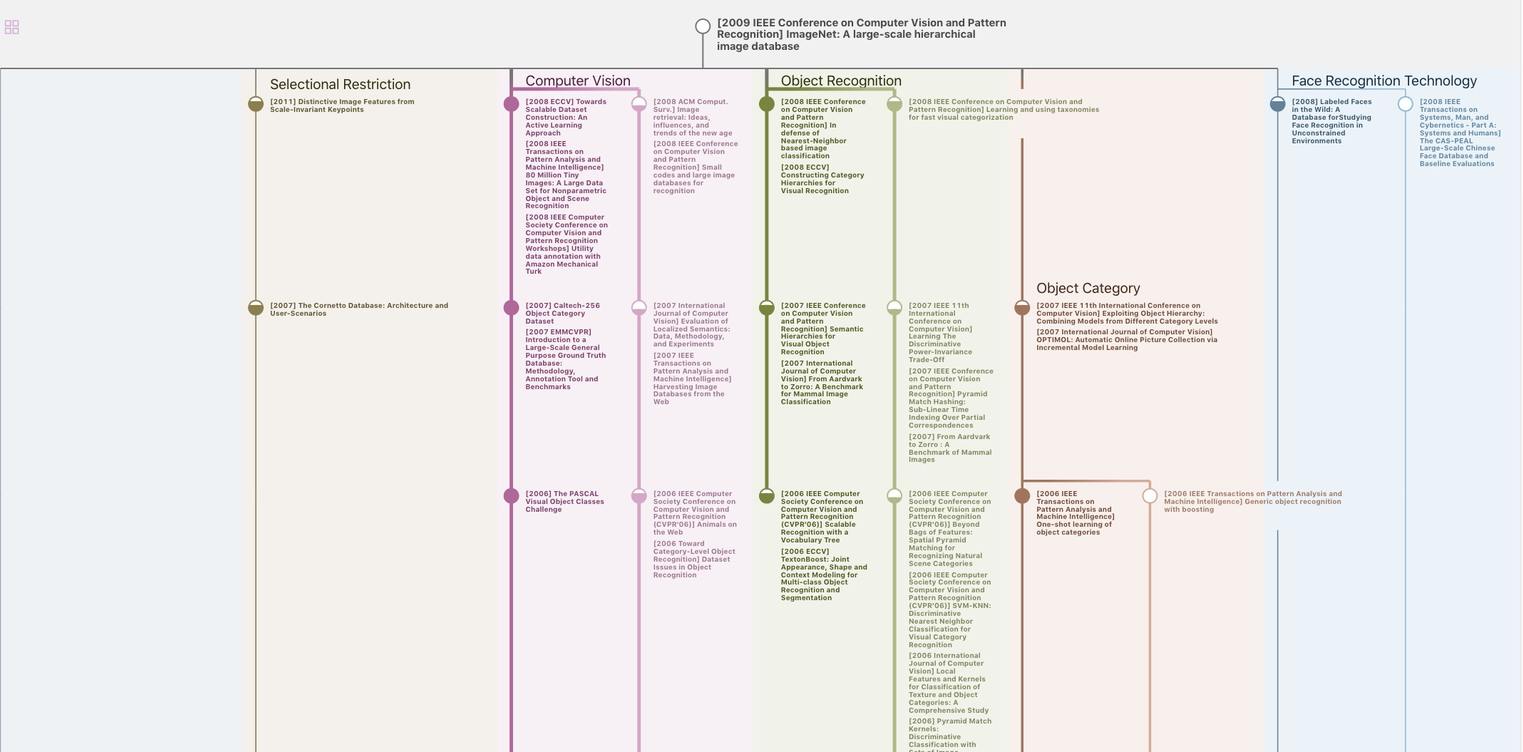
生成溯源树,研究论文发展脉络
Chat Paper
正在生成论文摘要