Efficient Shared Feature Learning for Cross-modality Person Re-identification
2022 14th International Conference on Wireless Communications and Signal Processing (WCSP)(2022)
摘要
Visible-infrared person re-identification is a challenging task, which aims to achieve cross-modal pedestrian retrieval. How to reduce the intra- and cross-modal discrepancies of the same pedestrian is the difficult part of this task. In response to the aforementioned issue, we propose a new framework named Shared Local Feature Learning Network (SLFL-Net) for mining more efficient shared features. Focusing on network designing, SLFL-Net uses ResNet50 as the backbone and employs the mixed attention mechanism to highlight important features. In addition, to obtain the fine-grained information of heterogeneous pedestrian images, we explore the local feature learning module for cross-modality person re-identification. For metric learning, we present two center-based losses to shorten intra- and cross-modal distances. The learned representation is more effective when the proposed loss function and local feature learning module are combined. Numerous experiments are performed on the public dataset, and the experimental results illustrate that our method can significantly improve the performance of the cross-modality task.
更多查看译文
关键词
Cross-modality,person re-identification,shared feature,loss
AI 理解论文
溯源树
样例
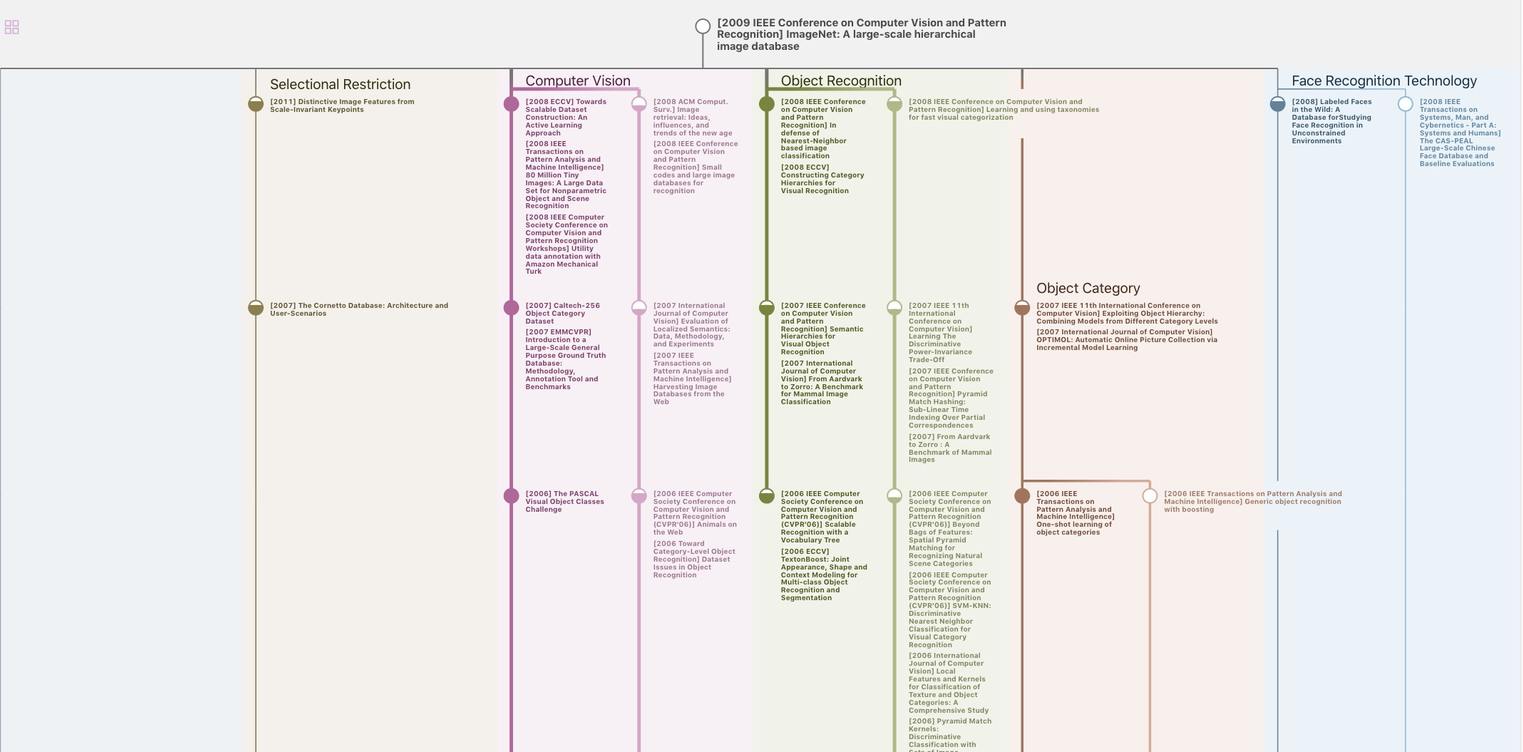
生成溯源树,研究论文发展脉络
Chat Paper
正在生成论文摘要