Unsupervised Hashing via Similarity Distribution Calibration
arxiv(2023)
摘要
Existing unsupervised hashing methods typically adopt a feature similarity preservation paradigm. As a result, they overlook the intrinsic similarity capacity discrepancy between the continuous feature and discrete hash code spaces. Specifically, since the feature similarity distribution is intrinsically biased (e.g., moderately positive similarity scores on negative pairs), the hash code similarities of positive and negative pairs often become inseparable (i.e., the similarity collapse problem). To solve this problem, in this paper a novel Similarity Distribution Calibration (SDC) method is introduced. Instead of matching individual pairwise similarity scores, SDC aligns the hash code similarity distribution towards a calibration distribution (e.g., beta distribution) with sufficient spread across the entire similarity capacity/range, to alleviate the similarity collapse problem. Extensive experiments show that our SDC outperforms the state-of-the-art alternatives on both coarse category-level and instance-level image retrieval tasks, often by a large margin. Code is available at https://github.com/kamwoh/sdc.
更多查看译文
关键词
similarity distribution calibration
AI 理解论文
溯源树
样例
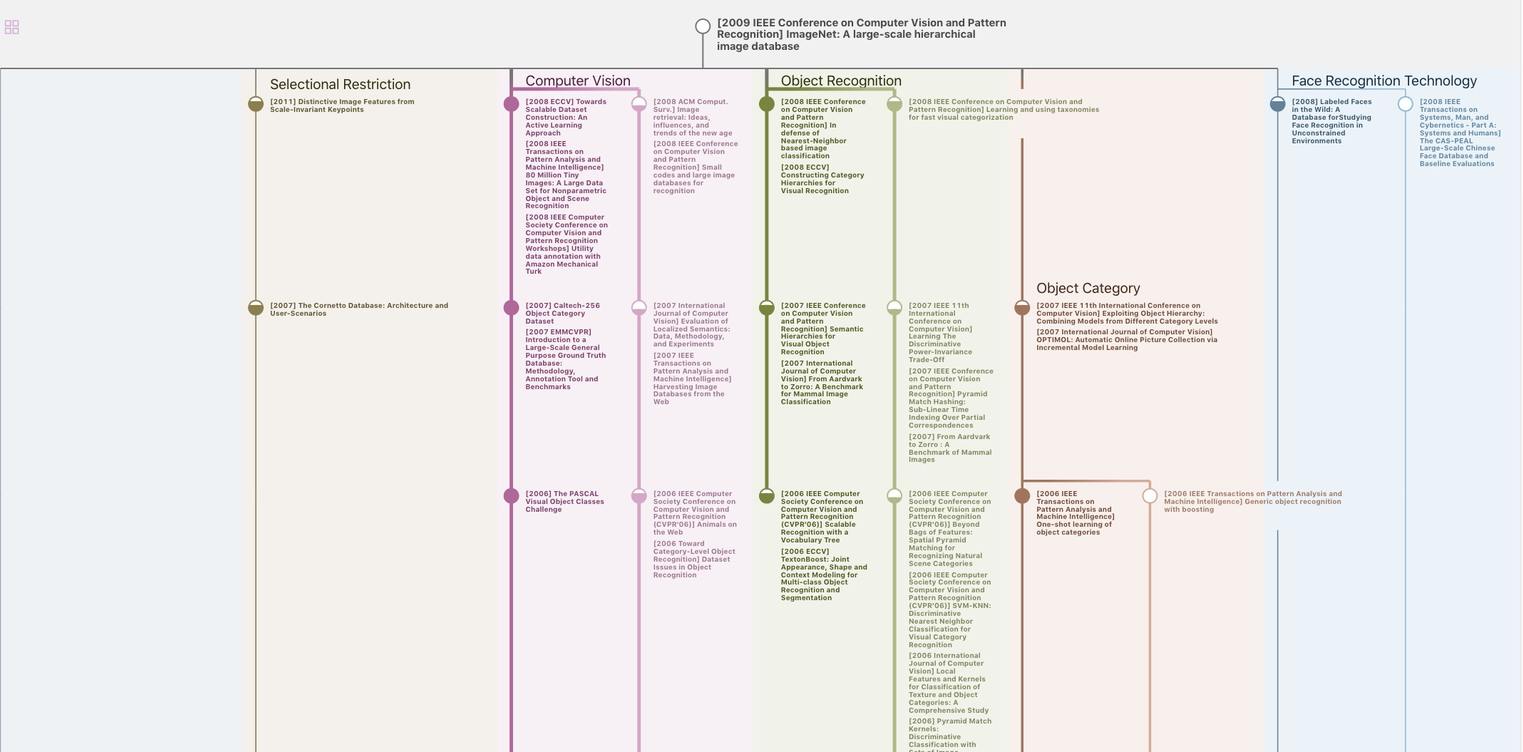
生成溯源树,研究论文发展脉络
Chat Paper
正在生成论文摘要