FedABC: Targeting Fair Competition in Personalized Federated Learning
arxiv(2023)
摘要
Federated learning aims to collaboratively train models without accessing their client's local private data. The data may be Non-IID for different clients and thus resulting in poor performance. Recently, personalized federated learning (PFL) has achieved great success in handling Non-IID data by enforcing regularization in local optimization or improving the model aggregation scheme on the server. However, most of the PFL approaches do not take into account the unfair competition issue caused by the imbalanced data distribution and lack of positive samples for some classes in each client. To address this issue, we propose a novel and generic PFL framework termed Federated Averaging via Binary Classification, dubbed FedABC. In particular, we adopt the ``one-vs-all'' training strategy in each client to alleviate the unfair competition between classes by constructing a personalized binary classification problem for each class. This may aggravate the class imbalance challenge and thus a novel personalized binary classification loss that incorporates both the under-sampling and hard sample mining strategies is designed. Extensive experiments are conducted on two popular datasets under different settings, and the results demonstrate that our FedABC can significantly outperform the existing counterparts.
更多查看译文
关键词
fair competition,fedabc,learning
AI 理解论文
溯源树
样例
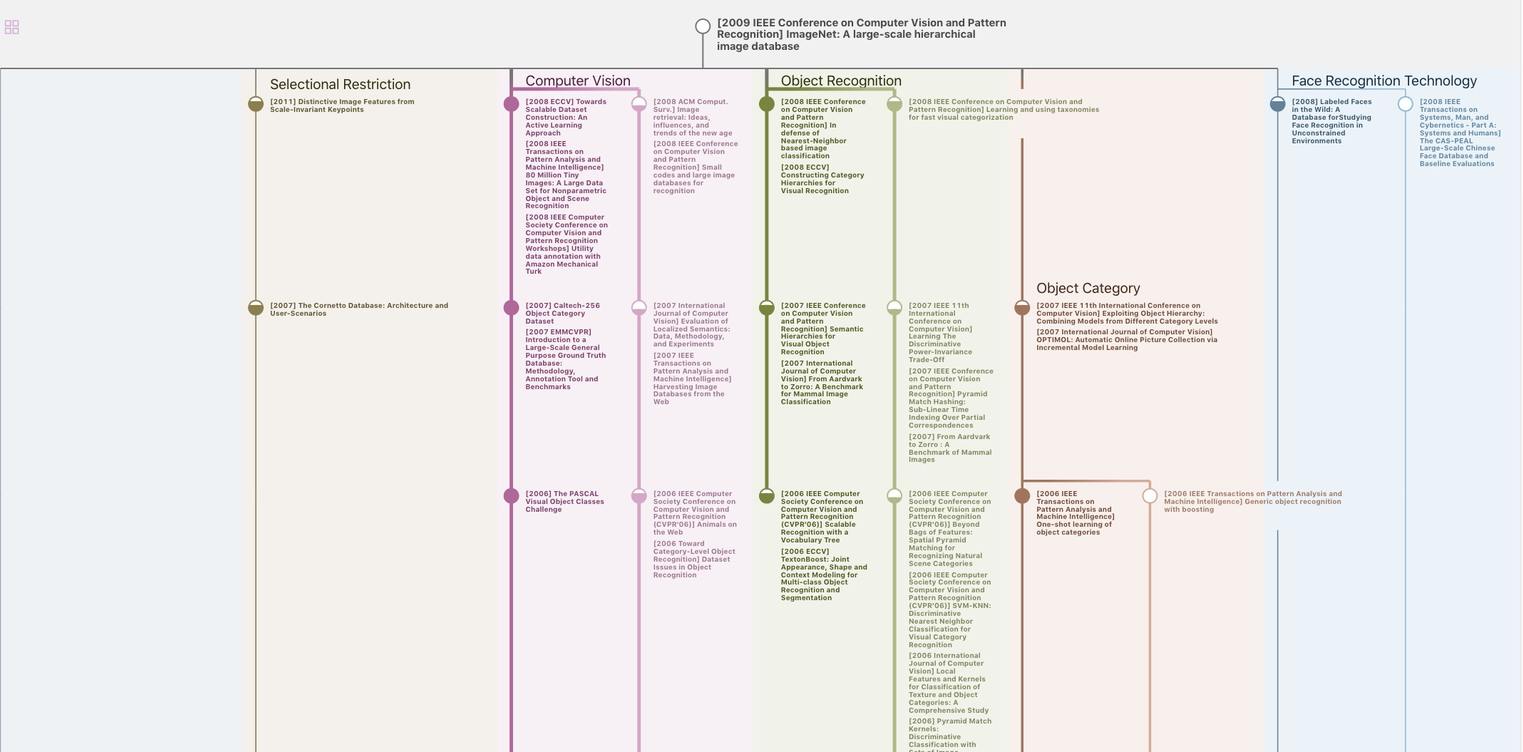
生成溯源树,研究论文发展脉络
Chat Paper
正在生成论文摘要