A non-convex piecewise quadratic approximation of ℓ _0 regularization: theory and accelerated algorithm
JOURNAL OF GLOBAL OPTIMIZATION(2022)
摘要
Non-convex regularization has been recognized as an especially important approach in recent studies to promote sparsity. In this paper, we study the non-convex piecewise quadratic approximation (PQA) regularization for sparse solutions of the linear inverse problem. It is shown that exact recovery of sparse signals and stable recovery of compressible signals are possible through local optimum of this regularization. After developing a thresholding representation theory for PQA regularization, we propose an iterative PQA thresholding algorithm (PQA algorithm) to solve this problem. The PQA algorithm converges to a local minimizer of the regularization, with an eventually linear convergence rate. Furthermore, we adopt the idea of accelerated gradient method to design the accelerated iterative PQA thresholding algorithm (APQA algorithm), which is also linearly convergent, but with a faster convergence rate. Finally, we carry out a series of numerical experiments to assess the performance of both algorithms for PQA regularization. The results show that PQA regularization outperforms ℓ _1 and ℓ _1/2 regularizations in terms of accuracy and sparsity, while the APQA algorithm is demonstrated to be significantly better than the PQA algorithm.
更多查看译文
关键词
Iterative thresholding algorithm,Exact recovery,Sparsity optimization,Non-convex approximation,Accelerated gradient algorithm
AI 理解论文
溯源树
样例
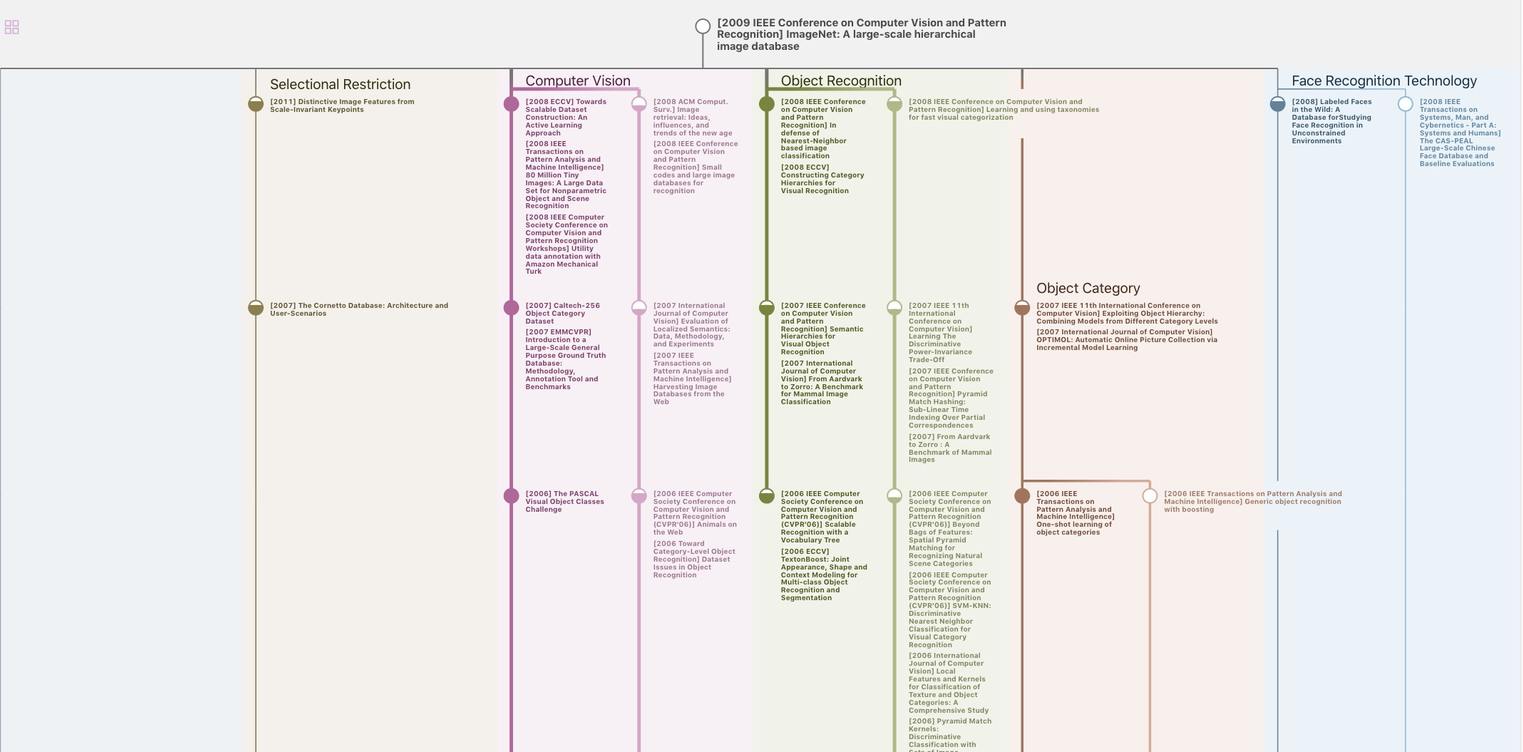
生成溯源树,研究论文发展脉络
Chat Paper
正在生成论文摘要