Overcoming data barriers in spatial agri-food systems analysis: A flexible imputation framework
JOURNAL OF AGRICULTURAL ECONOMICS(2023)
摘要
Suppressions in public data severely limit the usefulness of spatial data and hinder research applications. In this context, data imputation is necessary to deal with suppressed values. We present and validate a flexible data imputation method that can aid in the completion of under-determined data systems. The validations use Monte Carlo and optimisation modelling techniques to recover suppressed data tables from the 2017 US Census of Agriculture. We then use econometric models to evaluate the accuracy of imputations from alternative models. Various metrics of forecast accuracy (i.e., MAPE, BIC, etc.) show the flexibility and capacity of this approach to accurately recover suppressed data. To illustrate the value of our method, we compare the livestock water withdrawal estimations with imputed data and suppressed data to show the bias in research applications when suppressions are simply dropped from analysis.
更多查看译文
关键词
census of agriculture,data suppressions,mathematical programing,Monte Carlo,spatial data systems
AI 理解论文
溯源树
样例
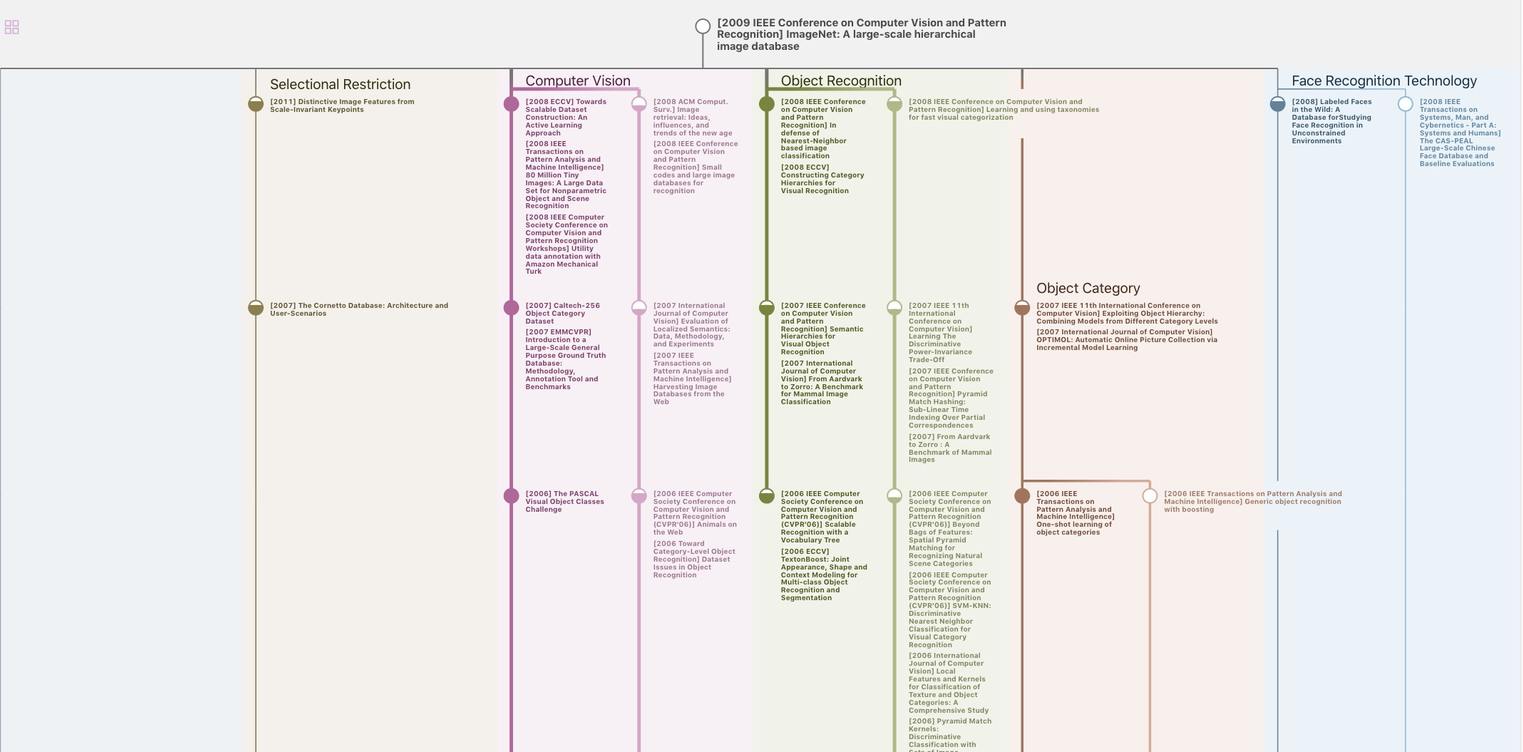
生成溯源树,研究论文发展脉络
Chat Paper
正在生成论文摘要