Detecting peatland vegetation patterns with multi-temporal field spectroscopy
GISCIENCE & REMOTE SENSING(2022)
摘要
Peatlands are one of the most significant terrestrial carbon pools, and the processes behind the carbon cycle in peatlands are strongly associated with different vegetation patterns. Handheld spectroradiometer data has been widely applied in ecological research, but there is a lack of studies on peatlands assessing how the temporal and spectral resolution affect the detectability of vegetation patterns. We collected field spectroscopy and vegetation inventory data at two northern boreal peatlands, Lompolojankka and Halssiaapa, between late May and August 2019. We conducted multivariate random forest regressions to examine the appropriate periods, benefits of multi-temporal data, and optimal spectral bandwidth and sampling interval for detecting plant communities and the two-dimensional (2D) %-cover, above-ground biomass (AGB) and leaf area index (LAI) of seven plant functional types (PFTs). In the best cross-site regression models for detecting plant community clusters (PCCs), R-2 was 42.6-48.0% (root mean square error (RMSE) 0.153-0.193), and for PFT 2D %-cover 53.9-69.8% (RMSE 8.2-17.6%), AGB 43.1-61.5% (RMSE 86.2-165.5 g/m(2)) and LAI 46.3-51.3% (RMSE 0.220-0.464 m(2)/m(2)). The multi-temporal data of the whole season increased R-2 by 13.7-24.6%-points and 10.2-33.0%-points for the PCC and PFT regressions, respectively. There was no single optimal temporal window for vegetation pattern detection for the two sites; in Lompolojankka the early growing season between late May and mid-June had the highest regression performance, while in Halssiaapa, the optimal period was during the peak season, from July to early August. In general, the spectral sampling interval between 1 to 10 nm yielded the best regression performance for most of the vegetation characteristics in Lompolojankka, whereas the optimal range extended to 20 nm in Halssiaapa. Our findings underscore the importance of fieldwork timing and the use of multi-temporal and hyperspectral data in detecting vegetation in spatially heterogeneous landscapes.
更多查看译文
关键词
Hyperspectral remote sensing,peatlands,plant communities,plant functional types,above-ground biomass,leaf area index,field spectroscopy
AI 理解论文
溯源树
样例
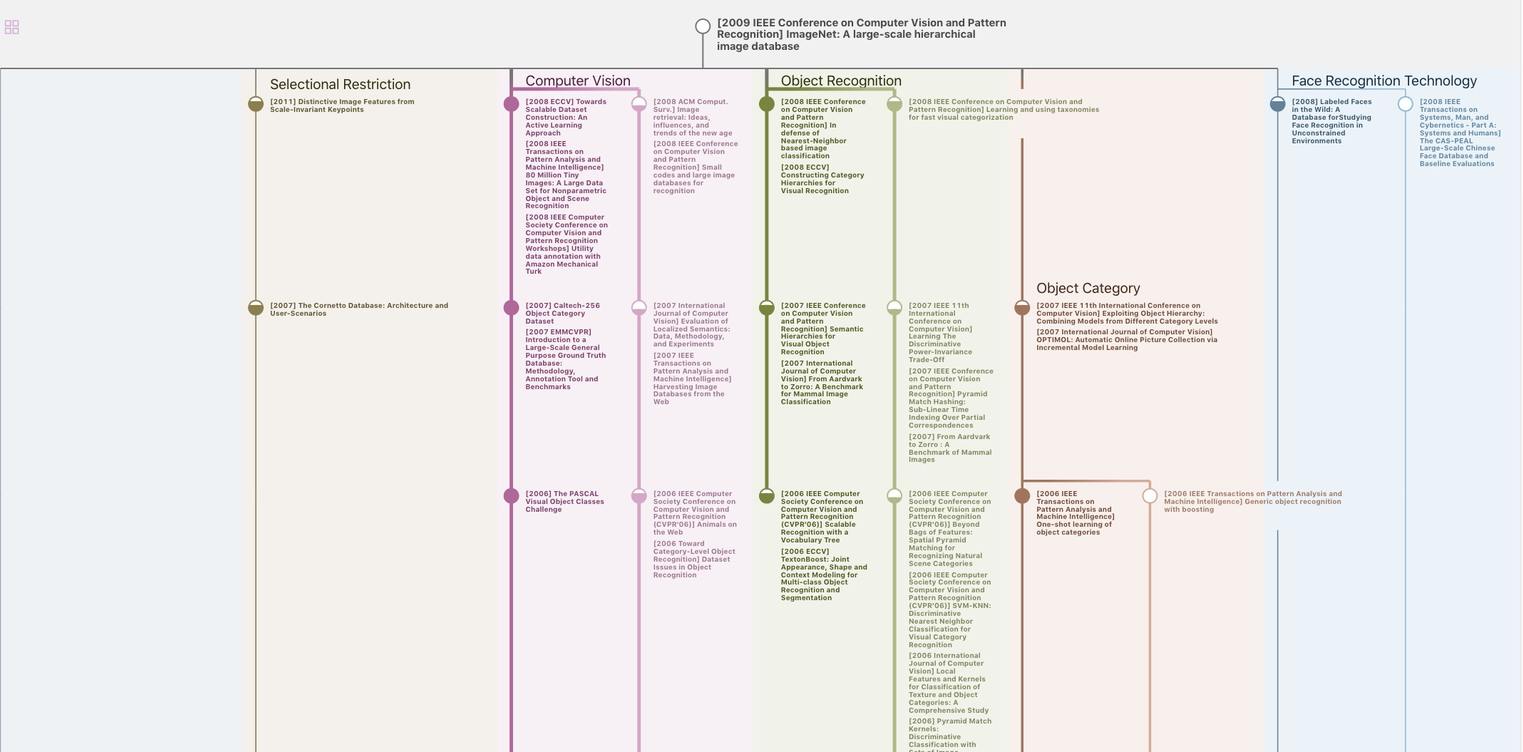
生成溯源树,研究论文发展脉络
Chat Paper
正在生成论文摘要