Emulating the evolution of phase separating microstructures using low-dimensional tensor decomposition and nonlinear regression
MRS BULLETIN(2023)
摘要
The phase-field method is an attractive computational tool for simulating microstructural evolution during phase separation, including solidification and spinodal decomposition. However, the high computational cost associated with solving phase-field equations currently limits our ability to comprehend phase transformations. This article reports a novel phase-field emulator based on the tensor decomposition of the evolving microstructures and their corresponding two-point correlation functions to predict microstructural evolution at arbitrarily small time scales that are otherwise nontrivial to achieve using traditional phase-field approaches. The reported technique is based on obtaining a low-dimensional representation of the microstructures via tensor decomposition, and subsequently, predicting the microstructure evolution in the low-dimensional space using Gaussian process regression (GPR). Once we obtain the microstructure prediction in the low-dimensional space, we employ a hybrid input–output phase-retrieval algorithm to reconstruct the microstructures. As proof of concept, we present the results on microstructure prediction for spinodal decomposition, although the method itself is agnostic of the material parameters. Results show that we are able to predict microstructure evolution sequences that closely resemble the true microstructures (average normalized mean square of 6.78 × 10^-7 ) at time scales half of that employed in obtaining training data. Our data-driven microstructure emulator opens new avenues to predict the microstructural evolution by leveraging phase-field simulations and physical experimentation where the time resolution is often quite large due to limited resources and physical constraints, such as the phase coarsening experiments previously performed in microgravity. Impact statement The phase-field method is an attractive computational tool for simulating microstructural evolution during phase separation, including solidification and spinodal decomposition. However, they remain computationally intensive due to the strict limits on the maximum time and length scales imposed by the numerical methods. This research presents a phase-field emulator that will predict the microstructural evolution observed in phase separating materials by leveraging the already existing data sets obtained from traditional phase-field modeling approaches. The phase-field emulator, which for the first time, couples a tensor representation and nonparametric tensor methods with microstructure modeling and representation, will enable high-throughput and facile prediction of the microstructural evolution, as opposed to solving computationally intensive phase-field simulations whenever a new simulation needs to be performed. Our data-driven microstructure emulator opens new avenues to predict the microstructural evolution by leveraging phase-field simulations and physical experimentation where the time resolution is often quite large due to limited resources and physical constraints, such as the phase coarsening experiments previously performed in microgravity. Graphical Abstract
更多查看译文
关键词
Phase field simulation,Gaussian process regression,Two-point correlation,Phase retrieval,Spinodal decomposition
AI 理解论文
溯源树
样例
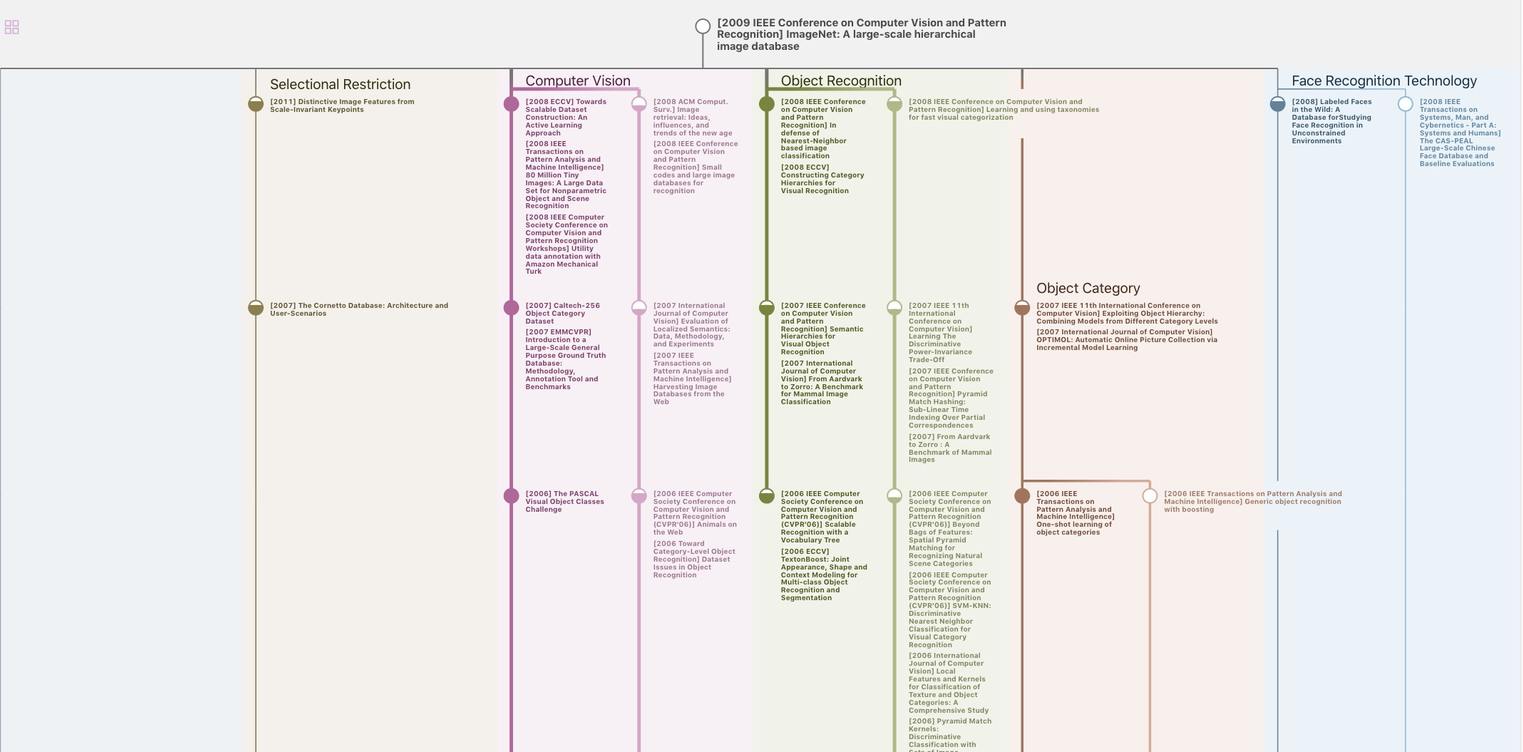
生成溯源树,研究论文发展脉络
Chat Paper
正在生成论文摘要