Can accurate demographic information about people who use prescription medications nonmedically be derived from Twitter?
Proceedings of the National Academy of Sciences of the United States of America(2023)
摘要
Traditional substance use (SU) surveillance methods, such as surveys, incur substantial lags. Due to the continuously evolving trends in SU, insights obtained via such methods are often outdated. Social media-based sources have been proposed for obtaining timely insights, but methods leveraging such data cannot typically provide fine-grained statistics about subpopulations, unlike traditional approaches. We address this gap by developing methods for automatically characterizing a large Twitter nonmedical prescription medication use (NPMU) cohort (n = 288,562) in terms of age-group, race, and gender. Our natural language processing and machine learning methods for automated cohort characterization achieved 0.88 precision (95% CI:0.84 to 0.92) for age-group, 0.90 (95% CI: 0.85 to 0.95) for race, and 94% accuracy (95% CI: 92 to 97) for gender, when evaluated against manually annotated gold-standard data. We compared automatically derived statistics for NPMU of tranquilizers, stimulants, and opioids from Twitter with statistics reported in the National Survey on Drug Use and Health (NSDUH) and the National Emergency Department Sample (NEDS). Distributions automatically estimated from Twitter were mostly consistent with the NSDUH [Spearman : race: 0.98 (< 0.005); age-group: 0.67 (< 0.005); gender: 0.66 (= 0.27)] and NEDS, with 34/65 (52.3%) of the Twitter-based estimates lying within 95% CIs of estimates from the traditional sources. Explainable differences (e.g., overrepresentation of younger people) were found for age-group-related statistics. Our study demonstrates that accurate subpopulation-specific estimates about SU, particularly NPMU, may be automatically derived from Twitter to obtain earlier insights about targeted subpopulations compared to traditional surveillance approaches.
更多查看译文
关键词
Twitter,machine learning,natural language processing,substance use,toxicovigilance
AI 理解论文
溯源树
样例
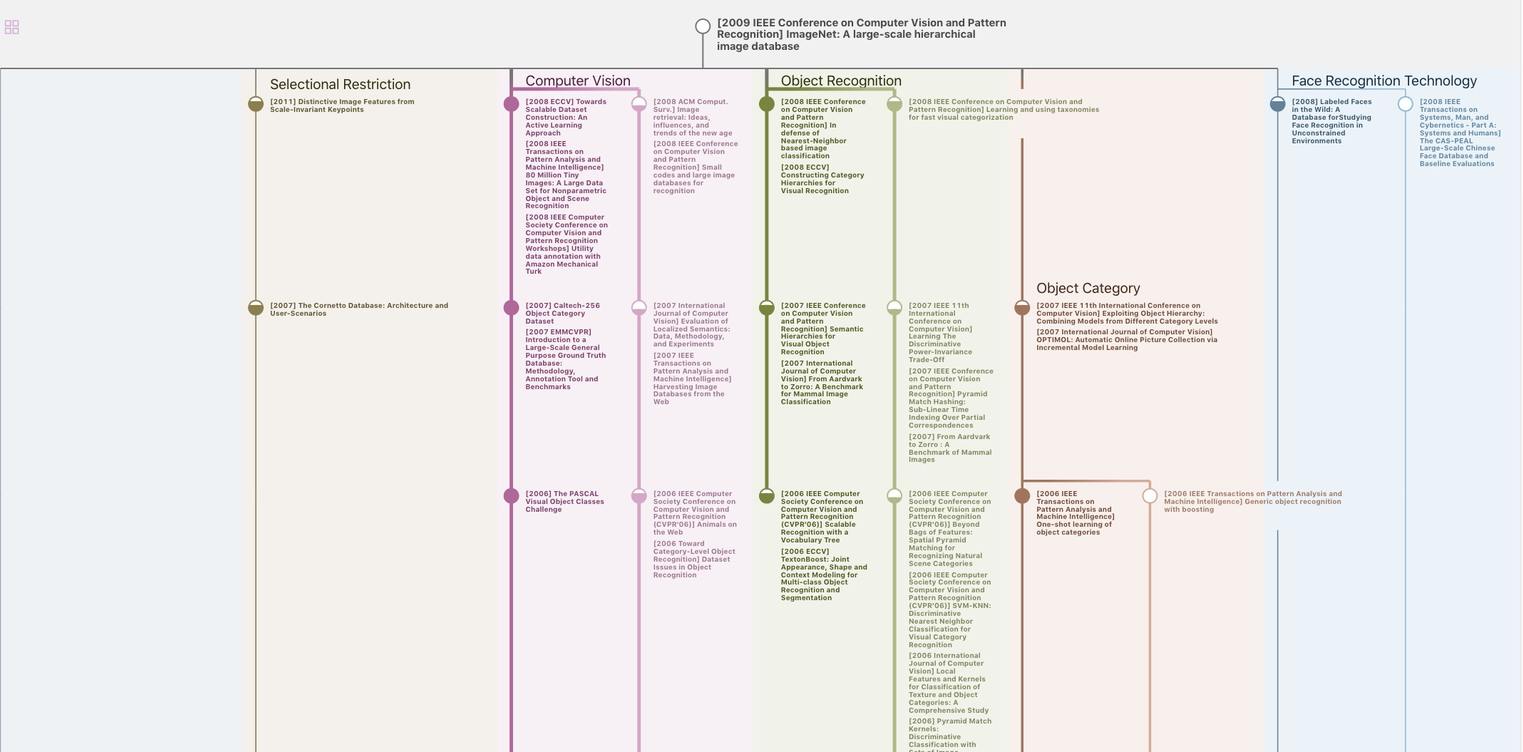
生成溯源树,研究论文发展脉络
Chat Paper
正在生成论文摘要