Semantic Fusion Enhanced Event Detection via Multi-Graph Attention Network with Skip Connection
IEEE TRANSACTIONS ON EMERGING TOPICS IN COMPUTATIONAL INTELLIGENCE(2023)
摘要
The target of event detection (ED) is to recognize and categorize event triggers in a given text. Because dependency trees transmit extensive structural information that has been proven effective, most contemporary ED approaches rely primarily on syntactic information. The majority of these methods, however, are ineffective due to two issues: a) how to mine and use both first-order and multi-order syntactic relations, and b) loss of information due to external dependency analysis toolbox. In this paper, we overcome the aforementioned two challenges by proposing the Semantic Fusion Enhanced Event Detection via Multi-Graph Attention Network with Skip Connection (SMGED) model. To solve the first problem mentioned above, SMGED constructs a multi-layer graph attention network with skip connections to capture syntactic relations with different orders and avoid information over-propagation. Then to solve the second problem, an information fusion module is proposed to fuse the contextual information and syntactic relations of each word to compensate for the lost information. We carried out extensive experiments and compared the proposed SMGED to existing methods. The comparison results validated the proposed model's effectiveness and superiority.
更多查看译文
关键词
Event detection,deep learning,graph convolutional network,dependency parsing tree
AI 理解论文
溯源树
样例
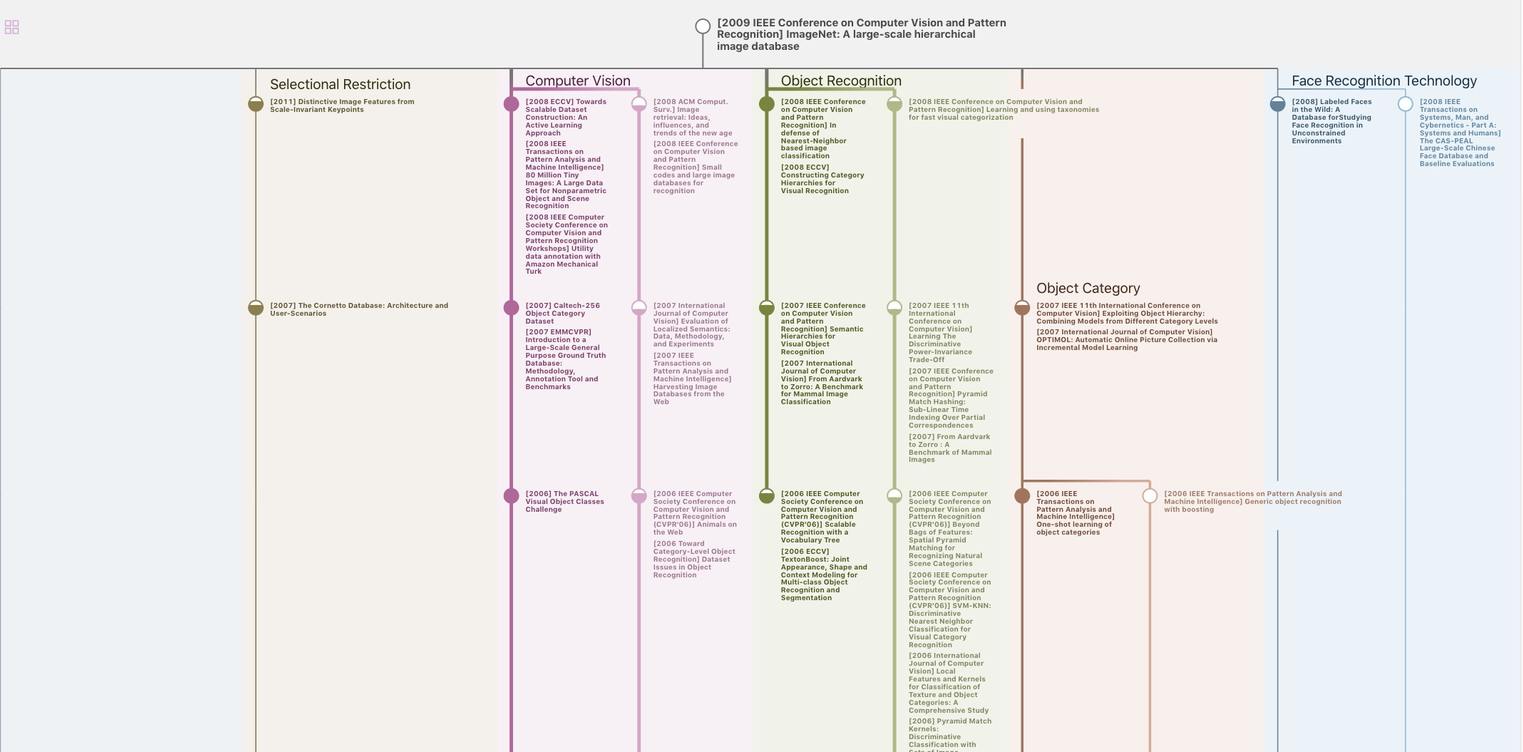
生成溯源树,研究论文发展脉络
Chat Paper
正在生成论文摘要