Truthful Incentive Mechanism Design via Internalizing Externalities and LP Relaxation for Vertical Federated Learning
IEEE TRANSACTIONS ON COMPUTATIONAL SOCIAL SYSTEMS(2023)
摘要
Although vertical federated learning (VFL) has become a new paradigm of distributed machine learning for emerging multiparty joint modeling applications, how to effectively incentivize self-conscious clients to actively and reliably contribute to collaborative learning in VFL has become a critical issue. Existing efforts are inadequate to address this issue since the training sample size needs to be unified before model training in VFL. To this end, selfish clients should unconditionally and honestly declare their private information, such as model training costs and benefits. However, such an assumption is unrealistic. In this article, we develop the first Truthful incEntive mechAnism for VFL,
$\mathbb {TEA}$
, to handle both information self-disclosure and social utility maximization. Specifically, we design a transfer payment rule via internalizing externalities, which bundles the clients’ utilities with the social utility, making truthful reporting by clients be a Nash equilibrium. Theoretically, we prove that
$\mathbb {TEA}$
can achieve truthfulness and social utility maximization, as well as budget balance (BB) or individual rationality (IR). On this basis, we further design a sample size decision rule via linear programming (LP) relaxation to meet the requirements of different scenarios. Finally, extensive experiments on synthetic and real-world datasets validate the theoretical properties of
$\mathbb {TEA}$
and demonstrate its superiority compared with the state-of-the-art.
更多查看译文
关键词
Budgetary balance,incentive mechanism,individual rationality (IR),truthfulness,vertical federated learning (VFL)
AI 理解论文
溯源树
样例
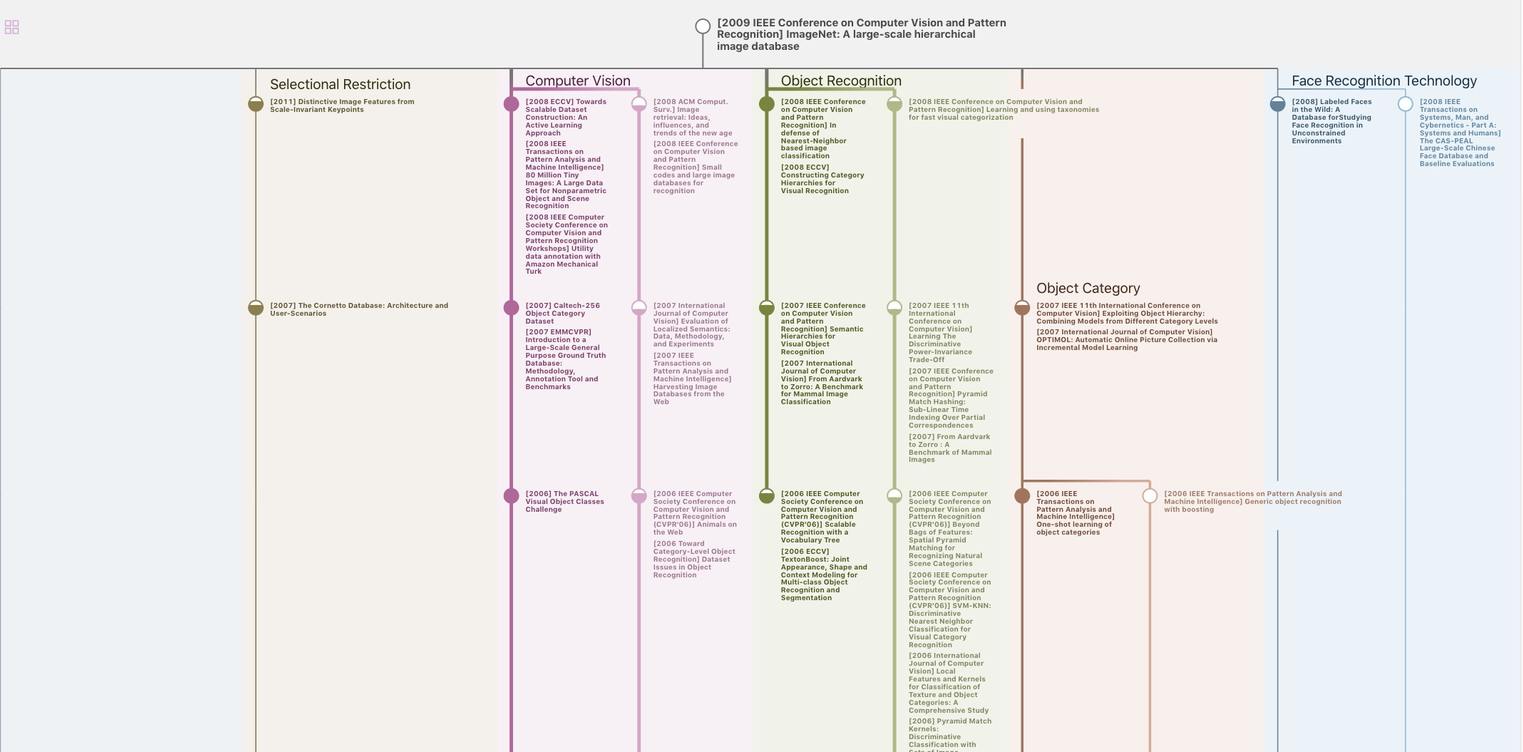
生成溯源树,研究论文发展脉络
Chat Paper
正在生成论文摘要