Time-attenuating Twin Delayed DDPG Reinforcement Learning for Trajectory Tracking Control of Quadrotors
arxiv(2023)
摘要
Continuous trajectory tracking control of quadrotors is complicated when considering noise from the environment. Due to the difficulty in modeling the environmental dynamics, tracking methodologies based on conventional control theory, such as model predictive control, have limitations on tracking accuracy and response time. We propose a Time-attenuating Twin Delayed DDPG, a model-free algorithm that is robust to noise, to better handle the trajectory tracking task. A deep reinforcement learning framework is constructed, where a time decay strategy is designed to avoid trapping into local optima. The experimental results show that the tracking error is significantly small, and the operation time is one-tenth of that of a traditional algorithm. The OpenAI Mujoco tool is used to verify the proposed algorithm, and the simulation results show that, the proposed method can significantly improve the training efficiency and effectively improve the accuracy and convergence stability.
更多查看译文
关键词
ddpg reinforcement learning,tracking control,trajectory
AI 理解论文
溯源树
样例
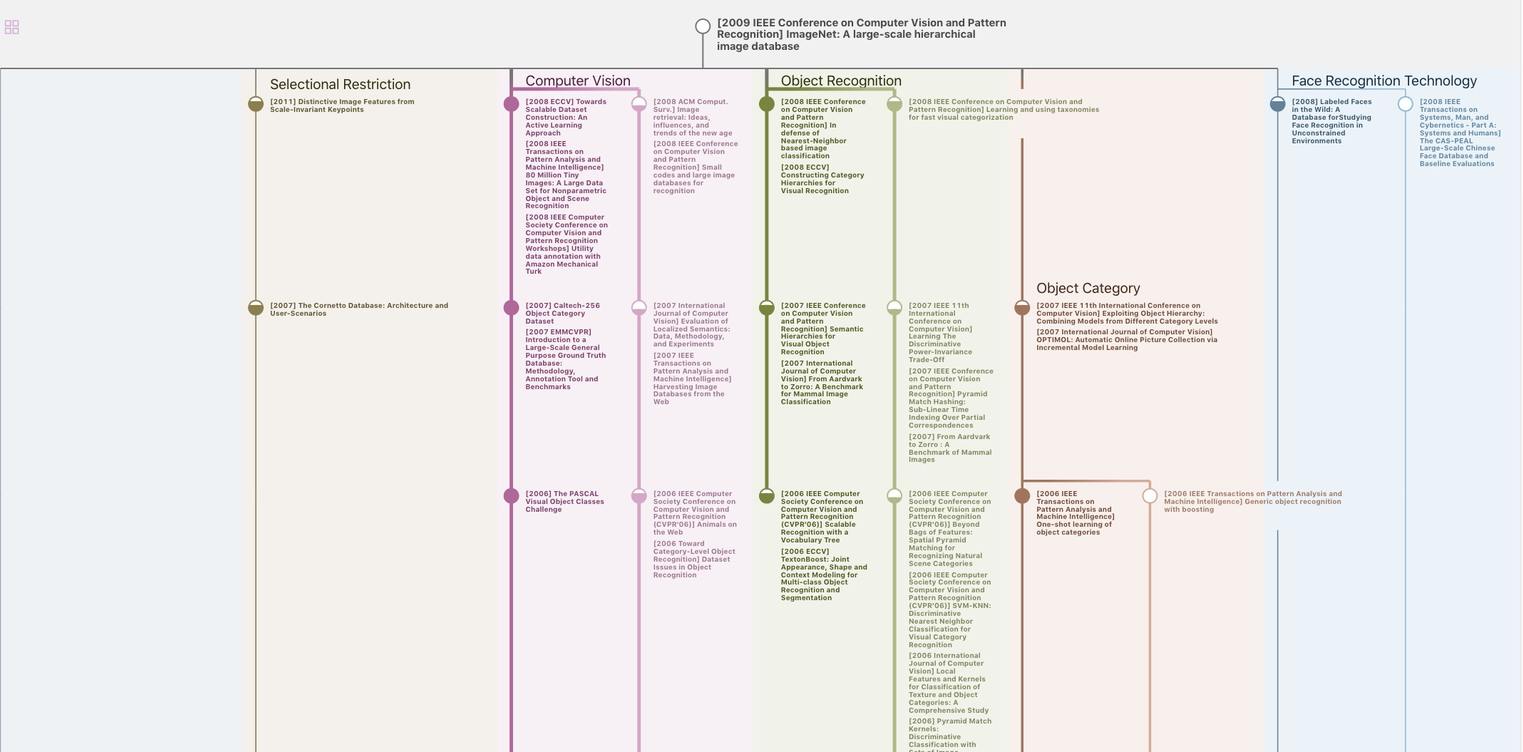
生成溯源树,研究论文发展脉络
Chat Paper
正在生成论文摘要