Hessian Based Smoothing Splines for Manifold Learning
arxiv(2023)
摘要
We propose a multidimensional smoothing spline algorithm in the context of manifold learning. We generalize the bending energy penalty of thin-plate splines to a quadratic form on the Sobolev space of a flat manifold, based on the Frobenius norm of the Hessian matrix. This leads to a natural definition of smoothing splines on manifolds, which minimizes square error while optimizing a global curvature penalty. The existence and uniqueness of the solution is shown by applying the theory of reproducing kernel Hilbert spaces. The minimizer is expressed as a combination of Green's functions for the biharmonic operator, and 'linear' functions of everywhere vanishing Hessian. Furthermore, we utilize the Hessian estimation procedure from the Hessian Eigenmaps algorithm to approximate the spline loss when the true manifold is unknown. This yields a particularly simple quadratic optimization algorithm for smoothing response values without needing to fit the underlying manifold. Analysis of asymptotic error and robustness are given, as well as discussion of out-of-sample prediction methods and applications.
更多查看译文
关键词
manifold learning
AI 理解论文
溯源树
样例
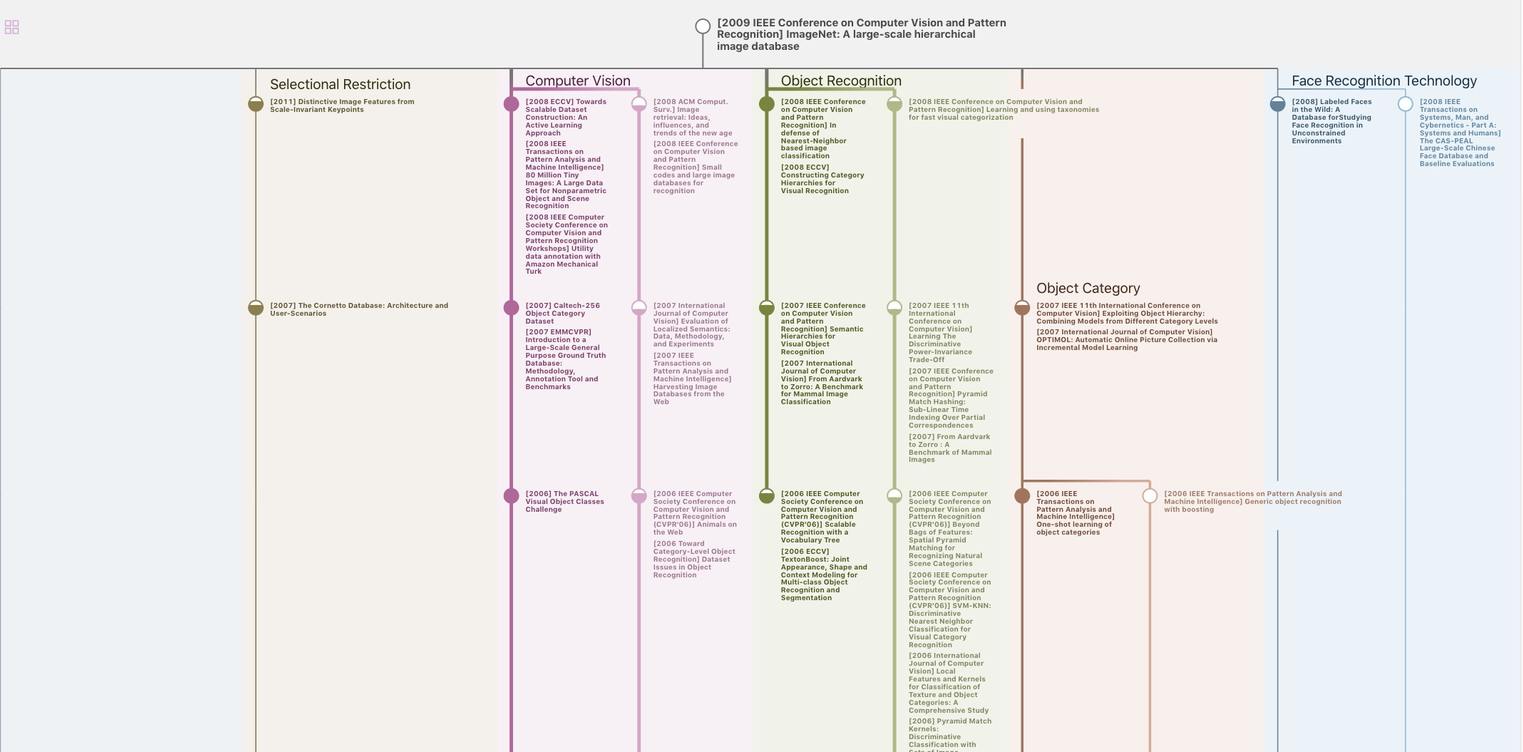
生成溯源树,研究论文发展脉络
Chat Paper
正在生成论文摘要