A deep learning-based fully automatic and clinical-ready framework for regional myocardial segmentation and myocardial ischemia evaluation
Medical & biological engineering & computing(2023)
摘要
Myocardial ischemia diagnosis with CT perfusion imaging (CTP) is important in coronary artery disease management. Traditional analysis procedure is time-consuming and error-prone due to the semi-manual and operator-dependent nature. To improve the diagnostic performance, a deep learning-based, fully automatic, and clinical-ready framework was developed. Two collaborating deep learning networks including a 3D U-Net for left ventricle segmentation and a CNN for anatomical landmarks detection were trained on 276 subjects. With our processing framework, the 17-segment left ventricular model was automatically generated conformed to the clinical standard. Myocardial blood flow computed by commercial software was extracted within each segment and visualized against the bull’s eye plot. The performance was validated on another 45 subjects. Coronary angiography and invasive fractional flow reserve measurements were also performed in these patients to serve as the gold standard for myocardial ischemia diagnosis. As a result, the diagnostic accuracy for our method was 81.08%, much higher than that for commercially available CTP analysis software (56.75%), and our method demonstrated a higher consistency (Kappa coefficient 0.759 vs. 0.585). Besides, the average processing time of our method was much lower (30 ± 10.5 s/subject vs. over 30 min/subject). In conclusion, the proposed deep learning-based framework could be a promising tool for assisting CTP analysis. Graphical Abstract
更多查看译文
关键词
Computed tomography perfusion (CTP),Diagnosis,Coronary artery disease,Myocardial segmentation,Deep learning
AI 理解论文
溯源树
样例
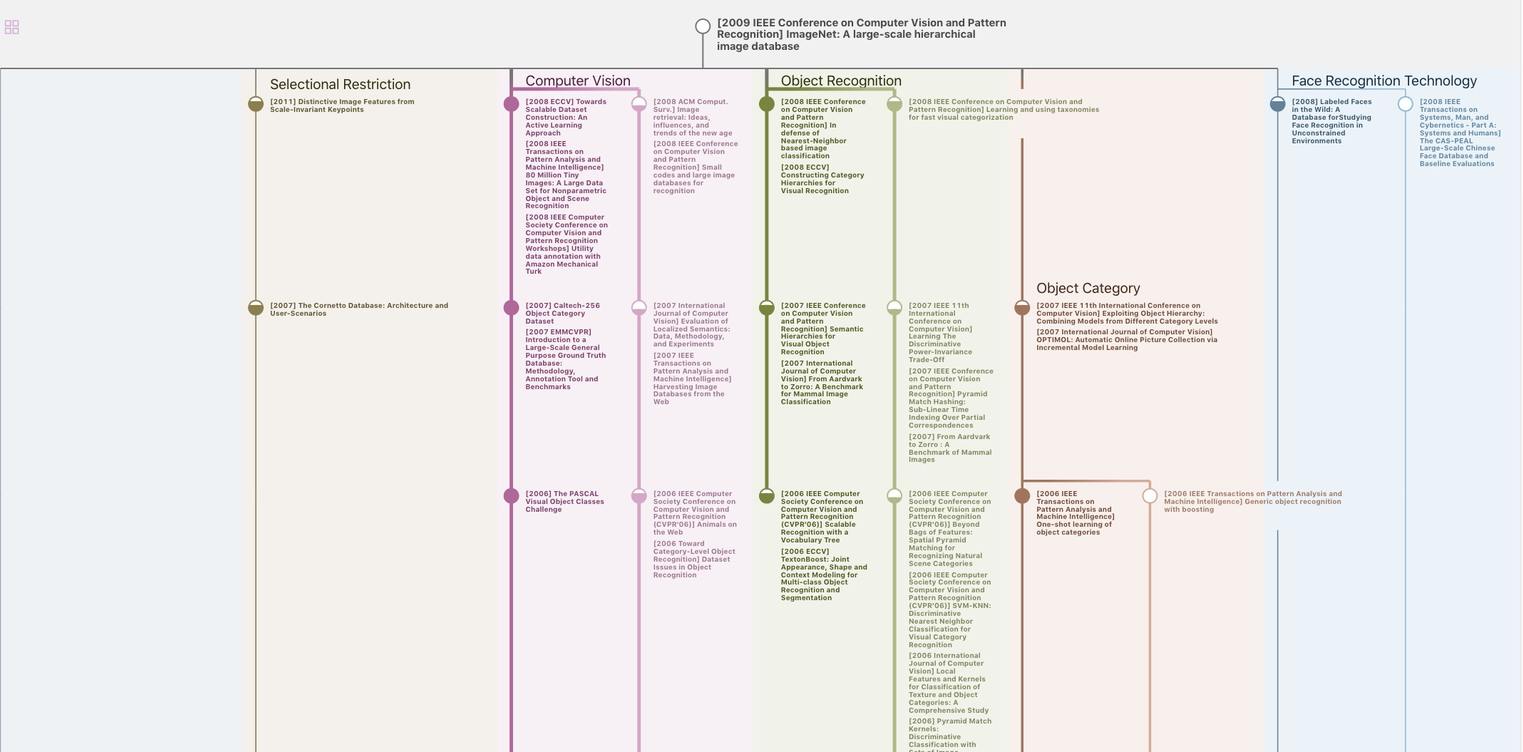
生成溯源树,研究论文发展脉络
Chat Paper
正在生成论文摘要