MS-CLAM: Mixed supervision for the classification and localization of tumors in Whole Slide Images.
Medical image analysis(2023)
摘要
Given the size of digitized Whole Slide Images (WSIs), it is generally laborious and time-consuming for pathologists to exhaustively delineate objects within them, especially with datasets containing hundreds of slides to annotate. Most of the time, only slide-level labels are available, giving rise to the development of weakly-supervised models. However, it is often difficult to obtain from such models accurate object localization, e.g., patches with tumor cells in a tumor detection task, as they are mainly designed for slide-level classification. Using the attention-based deep Multiple Instance Learning (MIL) model as our base weakly-supervised model, we propose to use mixed supervision - i.e., the use of both slide-level and patch-level labels - to improve both the classification and the localization performances of the original model, using only a limited amount of patch-level labeled slides. In addition, we propose an attention loss term to regularize the attention between key instances, and a paired batch method to create balanced batches for the model. First, we show that the changes made to the model already improve its performance and interpretability in the weakly-supervised setting. Furthermore, when using only between 12 and 62% of the total available patch-level annotations, we can reach performance close to fully-supervised models on the tumor classification datasets DigestPath2019 and Camelyon16.
更多查看译文
关键词
Camelyon16,Deep learning,DigestPath2019,Digital pathology,Mixed supervision
AI 理解论文
溯源树
样例
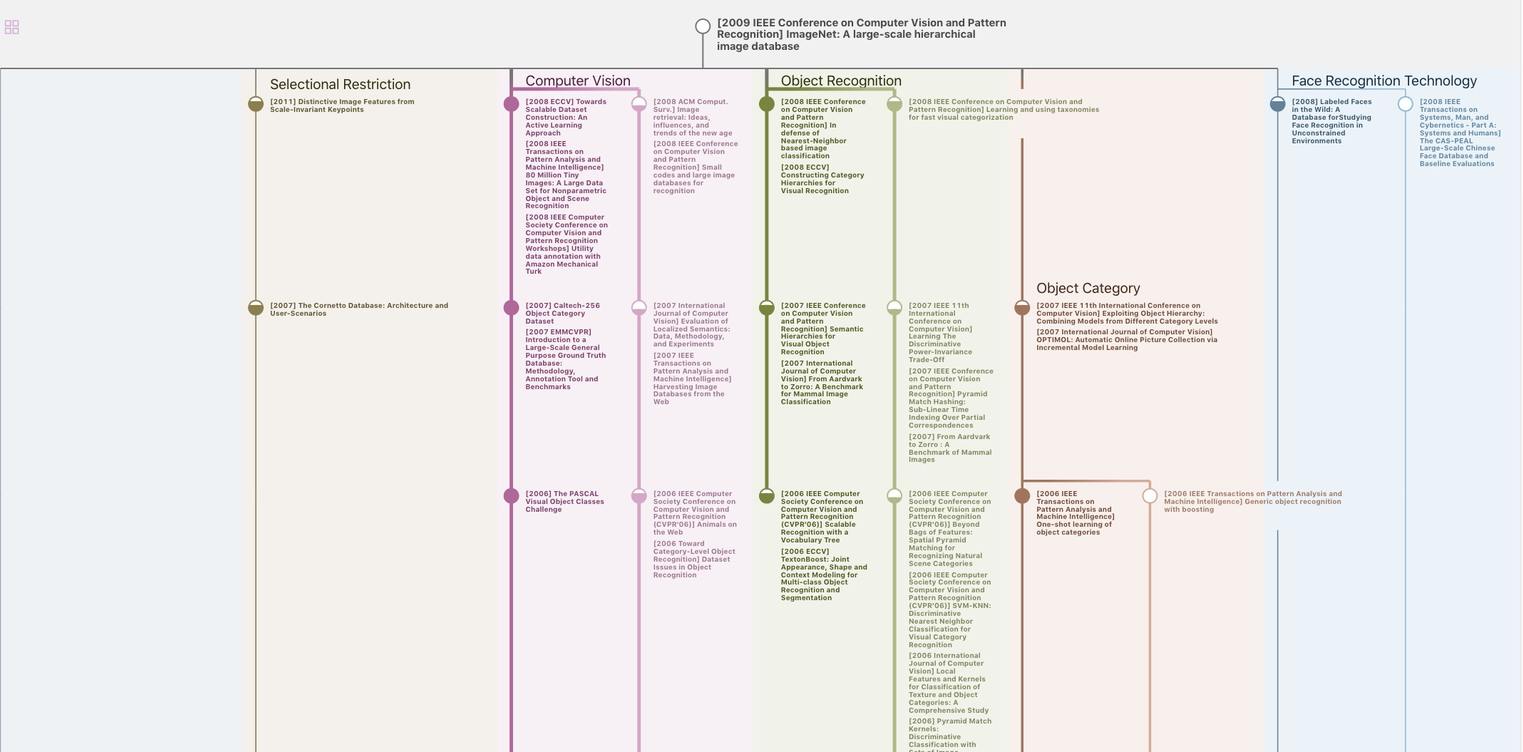
生成溯源树,研究论文发展脉络
Chat Paper
正在生成论文摘要