Filter bank sinc-convolutional network with channel self-attention for high performance motor imagery decoding.
Journal of neural engineering(2023)
摘要
Motor Imagery Brain-Computer Interface (MI-BCI) is an active Brain-Computer Interface (BCI) paradigm focusing on the identification of motor intention, which is one of the most important non-invasive BCI paradigms. In MI-BCI studies, deep learning-based methods (especially lightweight networks) have attracted more attention in recent years, but the decoding performance still needs further improving.To solve this problem, we designed a filter bank structure with sinc-convolutional layers for spatio-temporal feature extraction of MI-electroencephalography in four motor rhythms. The Channel Self-Attention method was introduced for feature selection based on both global and local information, so as to build a model called Filter Bank Sinc-convolutional Network with Channel Self-Attention for high performance MI-decoding. Also, we proposed a data augmentation method based on multivariate empirical mode decomposition to improve the generalization capability of the model.We performed an intra-subject evaluation experiment on unseen data of three open MI datasets. The proposed method achieved mean accuracy of 78.20% (4-class scenario) on BCI Competition IV IIa, 87.34% (2-class scenario) on BCI Competition IV IIb, and 72.03% (2-class scenario) on Open Brain Machine Interface (OpenBMI) dataset, which are significantly higher than those of compared deep learning-based methods by at least 3.05% (p= 0.0469), 3.18% (p= 0.0371), and 2.27% (p= 0.0024) respectively.This work provides a new option for deep learning-based MI decoding, which can be employed for building BCI systems for motor rehabilitation.
更多查看译文
关键词
brain–computer interface,data augmentation,deep learning,motor imagery,self-attention
AI 理解论文
溯源树
样例
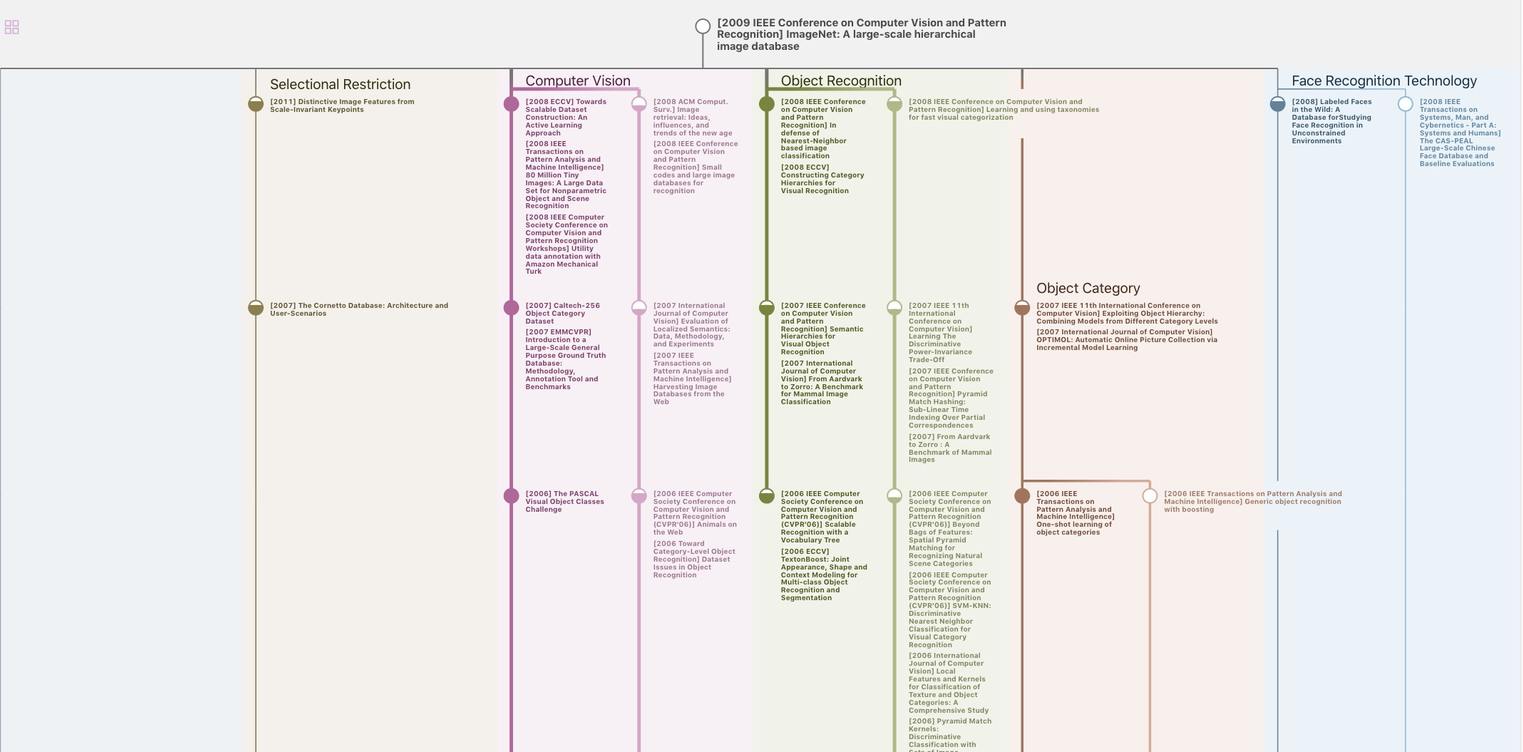
生成溯源树,研究论文发展脉络
Chat Paper
正在生成论文摘要