Pushing the Boundaries of Private, Large-Scale Query Answering
arxiv(2023)
摘要
We address the problem of efficiently and effectively answering large numbers of queries on a sensitive dataset while ensuring differential privacy (DP). We separately analyze this problem in two distinct settings, grounding our work in a state-of-the-art DP mechanism for large-scale query answering: the Relaxed Adaptive Projection (RAP) mechanism. The first setting is a classic setting in DP literature where all queries are known to the mechanism in advance. Within this setting, we identify challenges in the RAP mechanism's original analysis, then overcome them with an enhanced implementation and analysis. We then extend the capabilities of the RAP mechanism to be able to answer a more general and powerful class of queries (r-of-k thresholds) than previously considered. Empirically evaluating this class, we find that the mechanism is able to answer orders of magnitude larger sets of queries than prior works, and does so quickly and with high utility. We then define a second setting motivated by real-world considerations and whose definition is inspired by work in the field of machine learning. In this new setting, a mechanism is only given partial knowledge of queries that will be posed in the future, and it is expected to answer these future-posed queries with high utility. We formally define this setting and how to measure a mechanism's utility within it. We then comprehensively empirically evaluate the RAP mechanism's utility within this new setting. From this evaluation, we find that even with weak partial knowledge of the future queries that will be posed, the mechanism is able to efficiently and effectively answer arbitrary queries posed in the future. Taken together, the results from these two settings advance the state of the art on differentially private large-scale query answering.
更多查看译文
关键词
query,large-scale
AI 理解论文
溯源树
样例
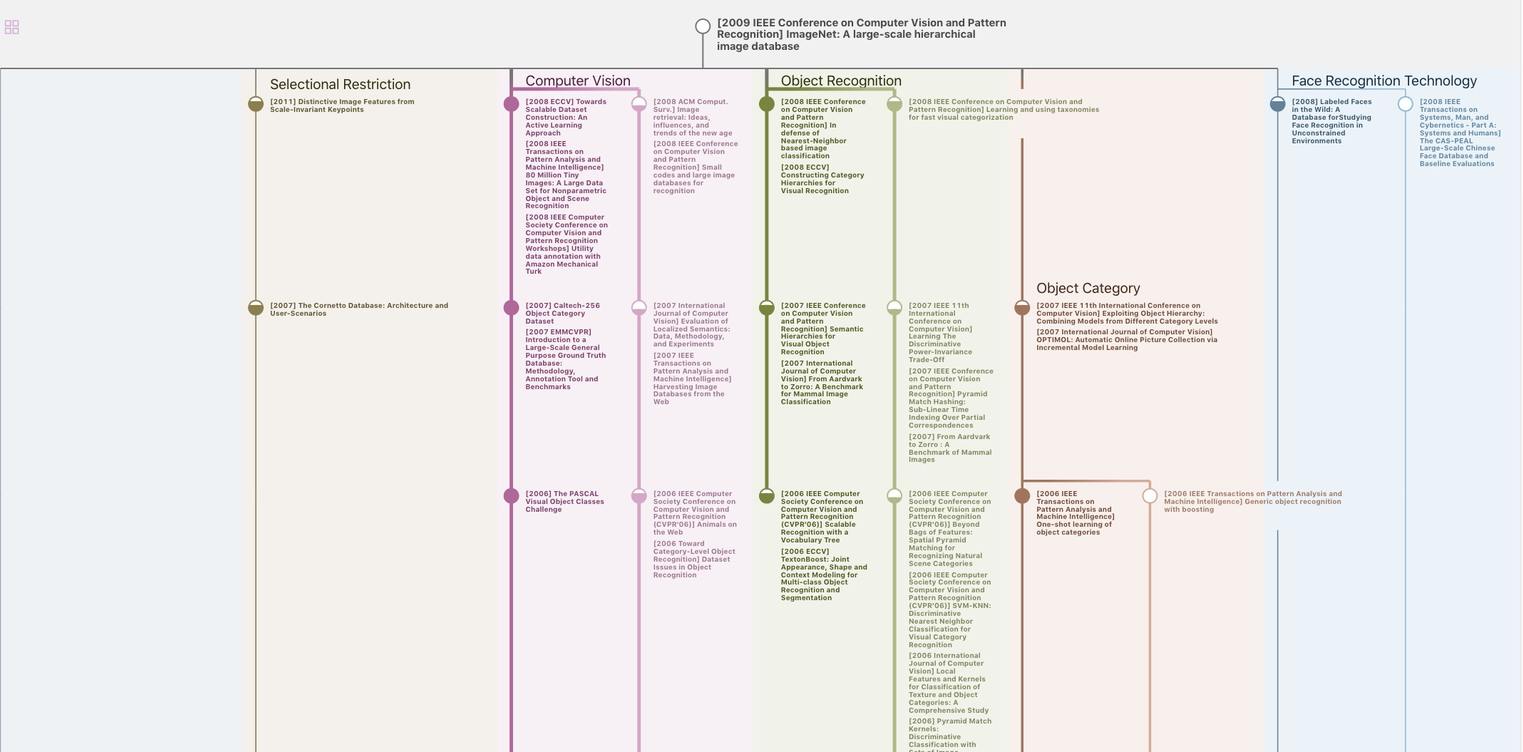
生成溯源树,研究论文发展脉络
Chat Paper
正在生成论文摘要