Towards Fairer and More Efficient Federated Learning via Multidimensional Personalized Edge Models
arxiv(2023)
摘要
Federated learning (FL) is an emerging technique that trains massive and geographically distributed edge data while maintaining privacy. However, FL has inherent challenges in terms of fairness and computational efficiency due to the rising heterogeneity of edges, and thus usually results in sub-optimal performance in recent state-of-the-art (SOTA) solutions. In this paper, we propose a Customized Federated Learning (CFL) system to eliminate FL heterogeneity from multiple dimensions. Specifically, CFL tailors personalized models from the specially designed global model for each client jointly guided by an online trained model-search helper and a novel aggregation algorithm. Extensive experiments demonstrate that CFL has full-stack advantages for both FL training and edge reasoning and significantly improves the SOTA performance w.r.t. model accuracy (up to 7.2% in the non-heterogeneous environment and up to 21.8% in the heterogeneous environment), efficiency, and FL fairness.
更多查看译文
关键词
more efficient federated learning,models
AI 理解论文
溯源树
样例
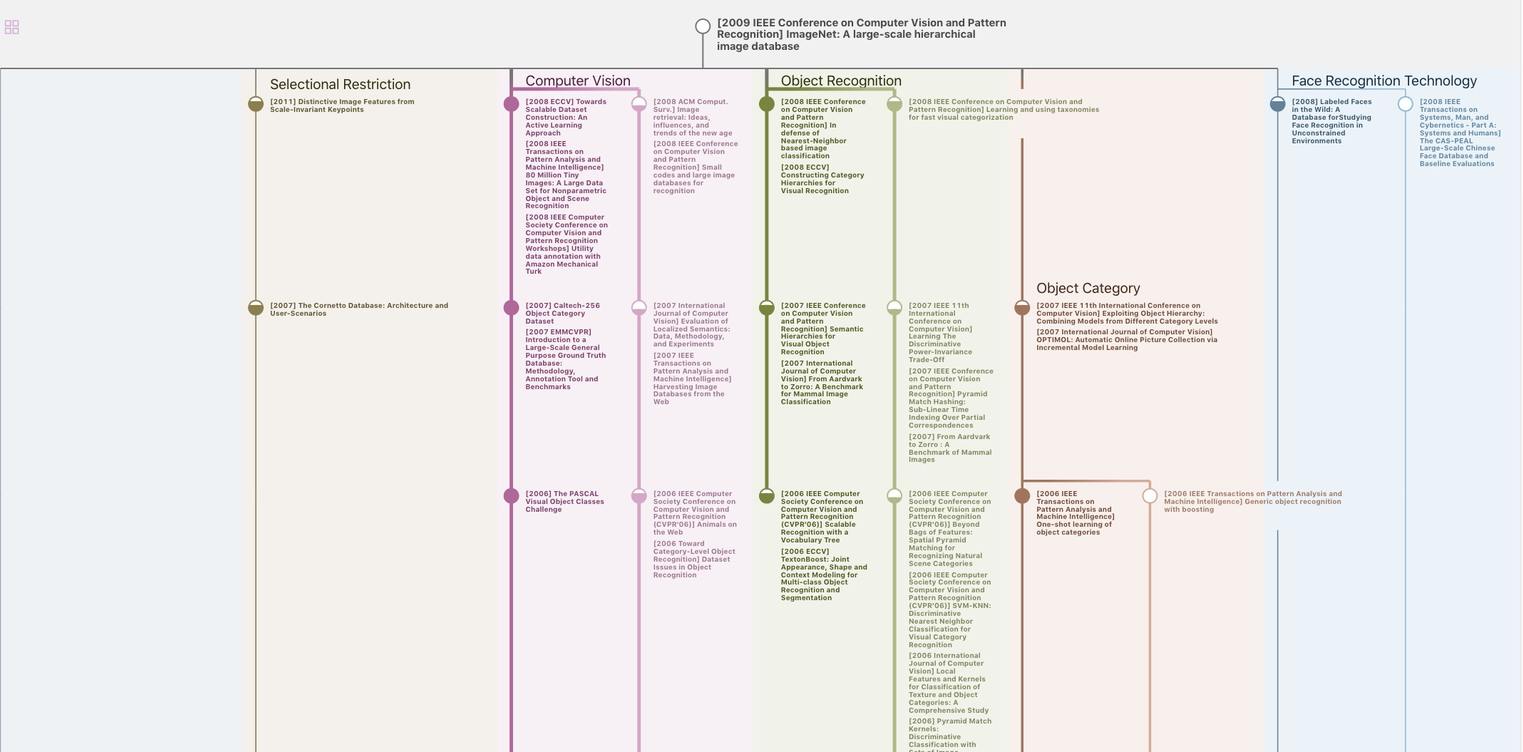
生成溯源树,研究论文发展脉络
Chat Paper
正在生成论文摘要